Identifying When Effect Restoration Will Improve Estimates of Causal Effect.
SDM(2019)
摘要
Several methods have been developed that combine multiple models learned on different data sets and then use that combination to reach conclusions that would not have been possible with any one of the models alone. We examine one such method—effect restoration—which was originally developed to mitigate the effects of poorly measured confounding variables in a causal model. We show how effect restoration can be used to combine results from different machine learning models and how the combined model can be used to estimate causal effects that are not identifiable from either of the original studies alone. We characterize the performance of effect restoration by using both theoretical analysis and simulation studies. Specifically, we show how conditional independence tests and common assumptions can help distinguish when effect restoration should and should not be applied, and we use empirical analysis to show the limited range of conditions under which effect restoration should be applied in practical situations.
更多查看译文
关键词
causal effect,effect restoration,estimates
AI 理解论文
溯源树
样例
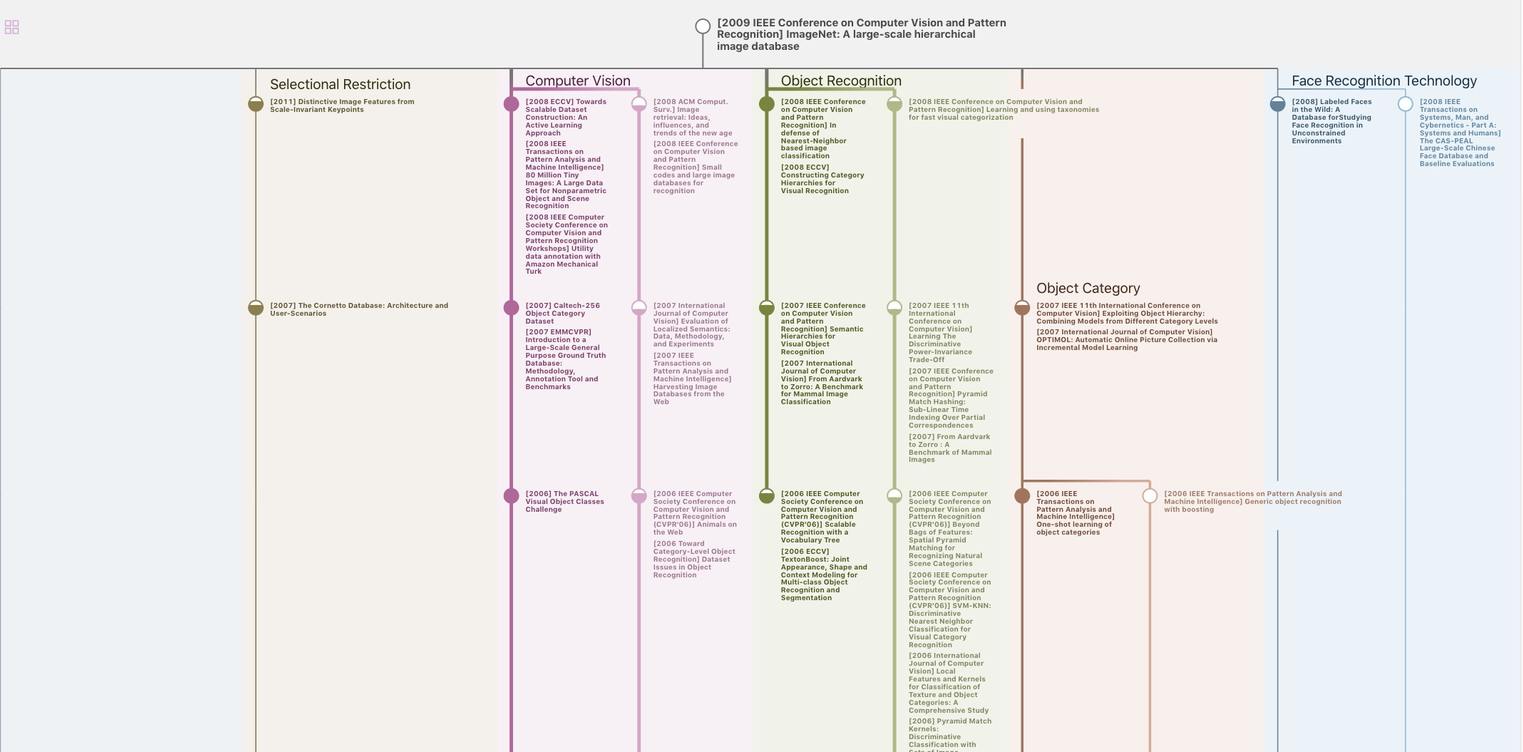
生成溯源树,研究论文发展脉络
Chat Paper
正在生成论文摘要