Experimenting with Machine Learning in Automated Intrusion Response.
Studies in Computational Intelligence(2020)
摘要
Traditionally, Intrusion Response Systems (IRSs) have had a strong reliance on network administrators to perform various responses for a network. Though this is expected, particularly with networks containing sensitive data, it is not completely practical, considering the ever growing demand for speed, scalability and automation in computer networks. This study presents a proof of concept IRS that provides for high speed networks, by using reinforcement learning to analyze and respond to trivial network attacks. This is done by creating a response system that is able to learn from the effectiveness of its own responses. All tests are conducted using an emulated network, that was designed to replicate real network behavior. Simulated attacks were used to train the IRS. Results of training were evaluated at intervals of 100, 500, 1000 and 2000 attacks. The findings of this work indicate that while applying reinforcement learning to IRSs is possible, adjustments may still be required to improve its performance.
更多查看译文
关键词
Machine learning,Intrusion response
AI 理解论文
溯源树
样例
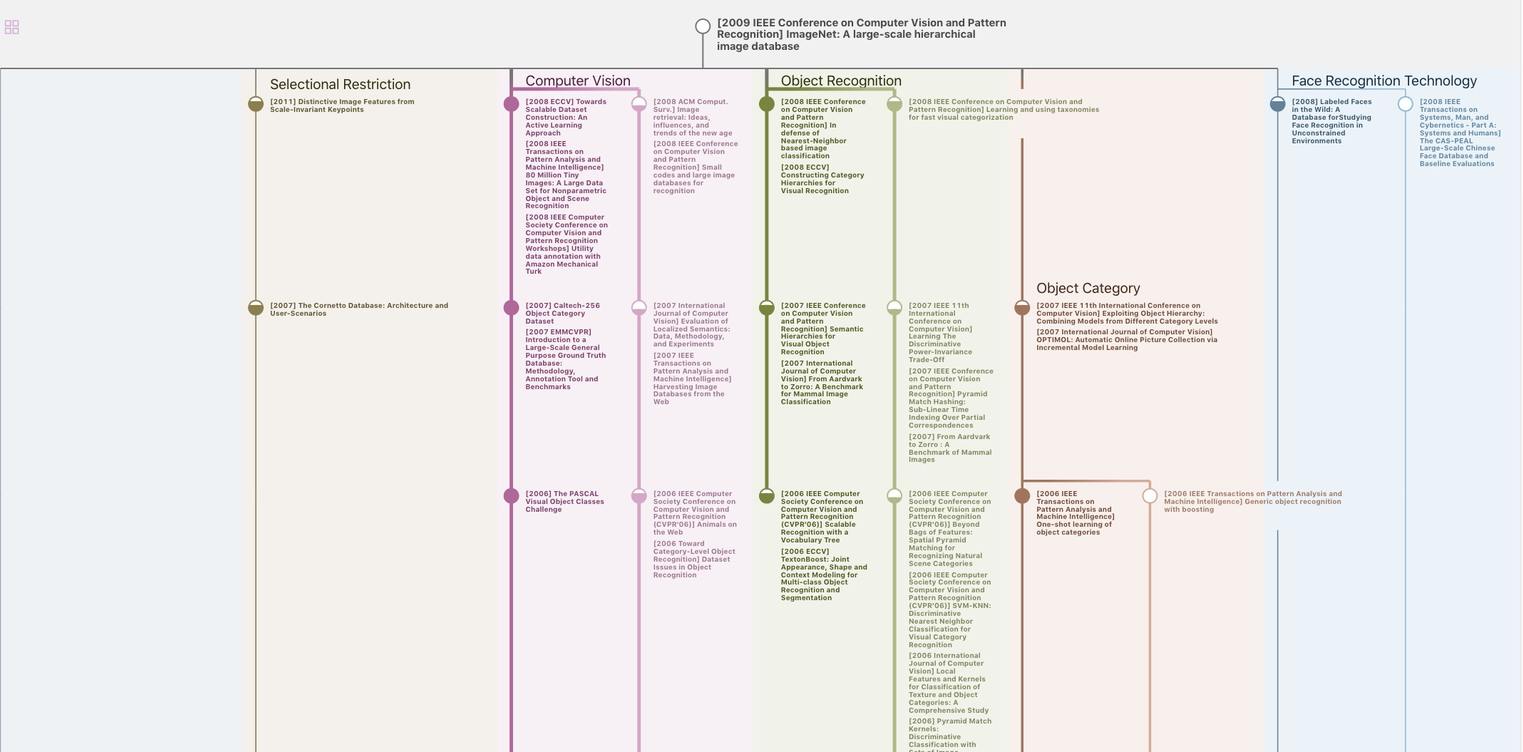
生成溯源树,研究论文发展脉络
Chat Paper
正在生成论文摘要