The PAU Survey: Background light estimation with deep learning techniques
arxiv(2019)
摘要
The PAU Survey (PAUS) is an imaging survey using a 40 narrow-band filter camera, named PAU Camera (PAUCam). Images obtained with the PAUCam are affected by scattered light: an optical effect consisting of light multiply reflected that deposits energy in specific detector regions contaminating the science measurements. Fortunately, scattered light is not a random effect, but it can be predicted and corrected for. However, with the current background estimation method around 8% of the PAUS flux measurements are affected by scattered light and therefore flagged. Additionally, failure to flag scattered light results in photometry and photo-z outliers. This paper introduces BKGnet, a deep neural network to predict the background and its associated error. We have found that BKGnet background predictions are very robust to distorting effects, such as scattered light and absorption, while still being statistically accurate. On average, the use of BKGnet improves the photometric flux measurements by 7% and up to 20% at the bright end. BKGnet also removes a systematic trend with magnitude in the i-band that is present with the current background estimation method. With BKGnet, we reduce the photometric redshift outlier rate by 35% for the best 20% galaxies selected with a photometric quality parameter.
更多查看译文
AI 理解论文
溯源树
样例
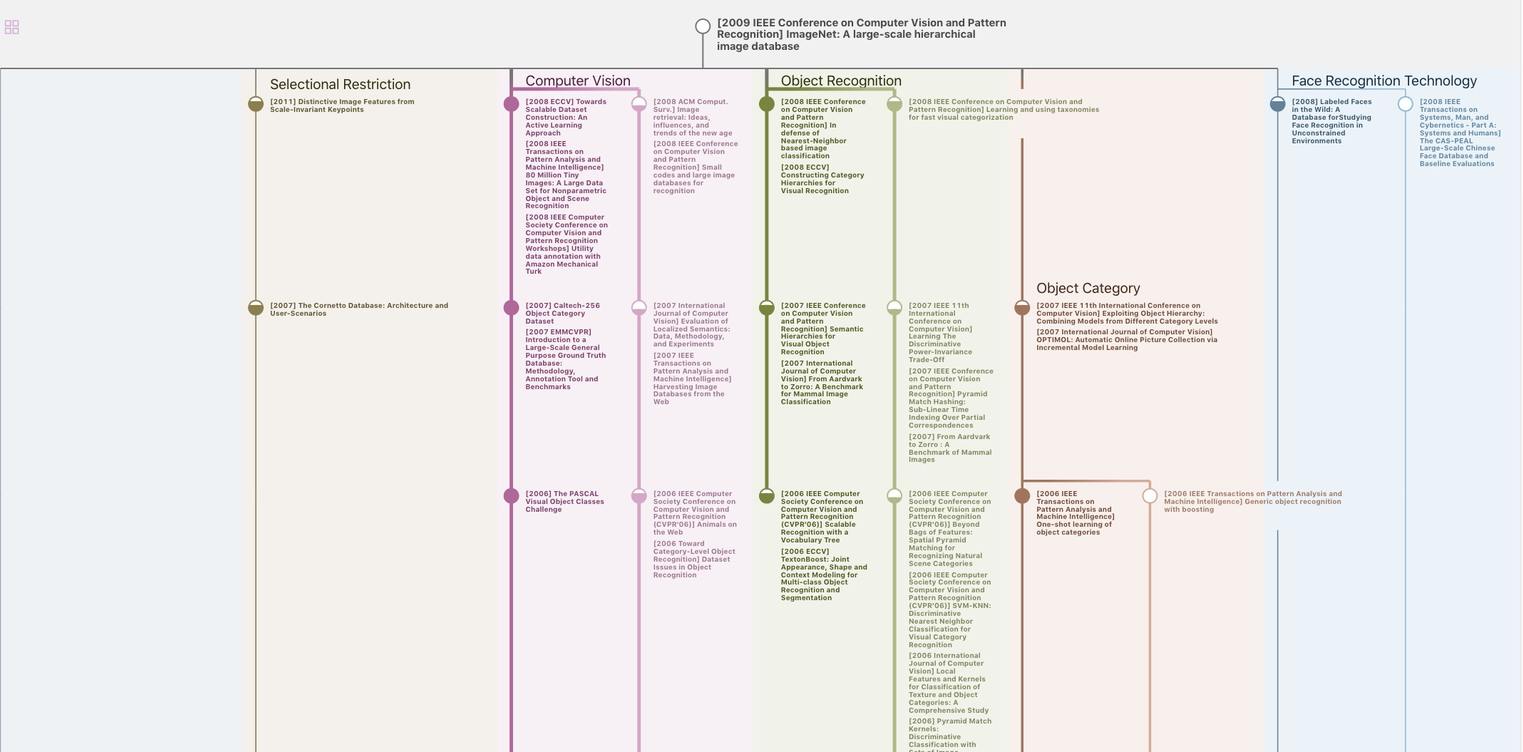
生成溯源树,研究论文发展脉络
Chat Paper
正在生成论文摘要