mfEGRA: Multifidelity efficient global reliability analysis through active learning for failure boundary location
STRUCTURAL AND MULTIDISCIPLINARY OPTIMIZATION(2021)
摘要
This paper develops mfEGRA, a multifidelity active learning method using data-driven adaptively refined surrogates for failure boundary location in reliability analysis. This work addresses the issue of prohibitive cost of reliability analysis using Monte Carlo sampling for expensive-to-evaluate high-fidelity models by using cheaper-to-evaluate approximations of the high-fidelity model. The method builds on the efficient global reliability analysis (EGRA) method, which is a surrogate-based method that uses adaptive sampling for refining Gaussian process surrogates for failure boundary location using a single- fidelity model. Our method introduces a two-stage adaptive sampling criterion that uses a multifidelity Gaussian process surrogate to leverage multiple information sources with different fidelities. The method combines expected feasibility criterion from EGRA with one-step lookahead information gain to refine the surrogate around the failure boundary. The computational savings from mfEGRA depends on the discrepancy between the different models, and the relative cost of evaluating the different models as compared to the high-fidelity model. We show that accurate estimation of reliability using mfEGRA leads to computational savings of ∼ 46
更多查看译文
关键词
Multi-fidelity, Active learning, Adaptive sampling, Probability of failure, Contour location, Classification, Gaussian process, Kriging, Multiple information sources, EGRA
AI 理解论文
溯源树
样例
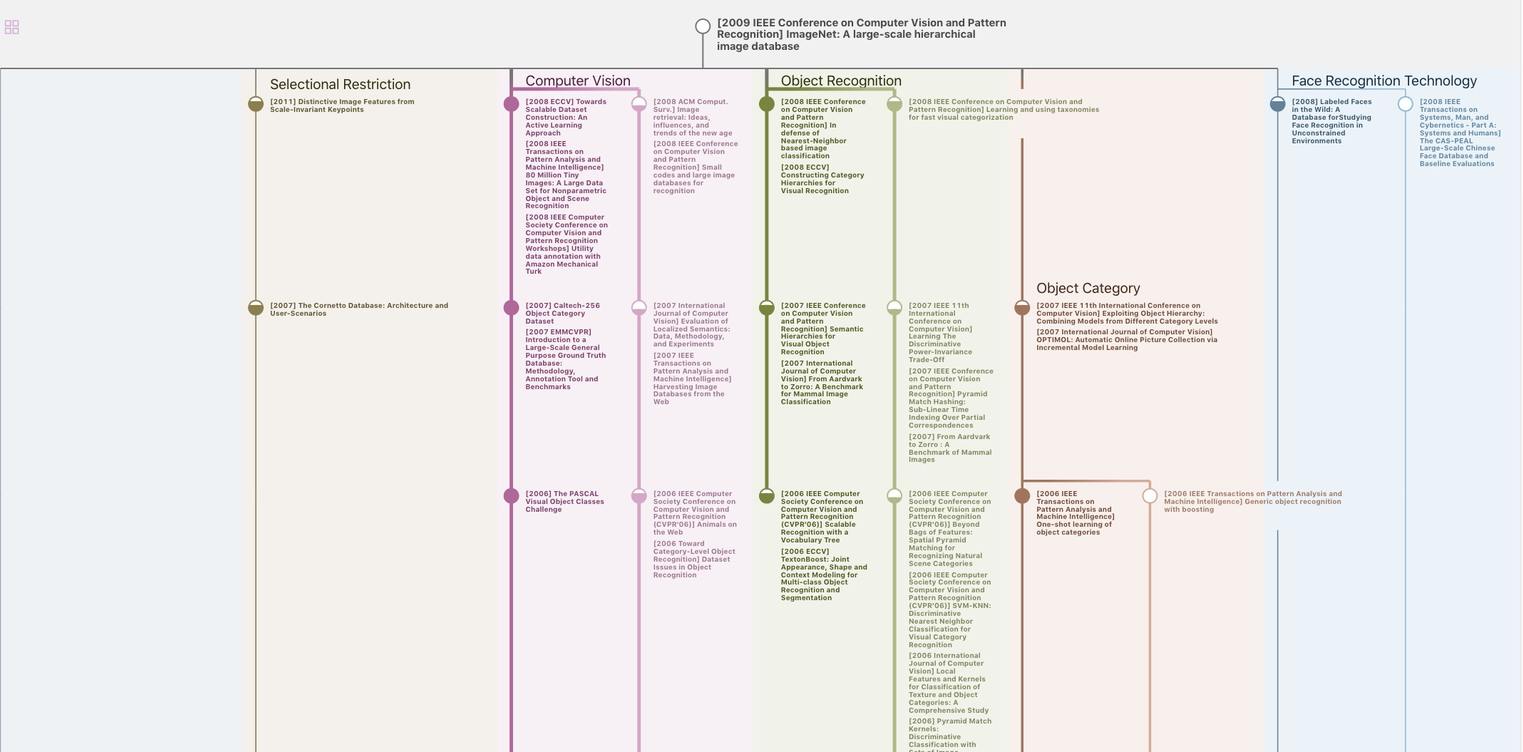
生成溯源树,研究论文发展脉络
Chat Paper
正在生成论文摘要