Label-PEnet: Sequential Label Propagation and Enhancement Networks for Weakly Supervised Instance Segmentation
arxiv(2019)
摘要
Weakly-supervised instance segmentation aims to detect and segment object instances precisely, given image-level labels only. Unlike previous methods which are composed of multiple offline stages, we propose Sequential Label Propagation and Enhancement Networks (referred as Label-PEnet) that progressively transforms image-level labels to pixel-wise labels in a coarse-to-fine manner. We design four cascaded modules including multi-label classification, object detection, instance refinement and instance segmentation, which are implemented sequentially by sharing the same backbone. The cascaded pipeline is trained alternatively with a curriculum learning strategy that generalizes labels from high level images to low-level pixels gradually with increasing accuracy. In addition, we design a proposal calibration module to explore the ability of classification networks to find key pixels that identify object parts, which serves as a post validation strategy running in the inverse order. We evaluate the efficiency of our Label-PEnet in mining instance masks on standard benchmarks: PASCAL VOC 2007 and 2012. Experimental results show that Label-PEnet outperforms the state-of-art algorithms by a clear margin, and obtains comparable performance even with fully supervised approaches.
更多查看译文
关键词
Label-PEnet,sequential label propagation,enhancement networks,weakly-supervised instance segmentation,pixel-wise labels,multilabel classification,instance refinement,high level images,low-level pixels,instance mask mining,image-level labels
AI 理解论文
溯源树
样例
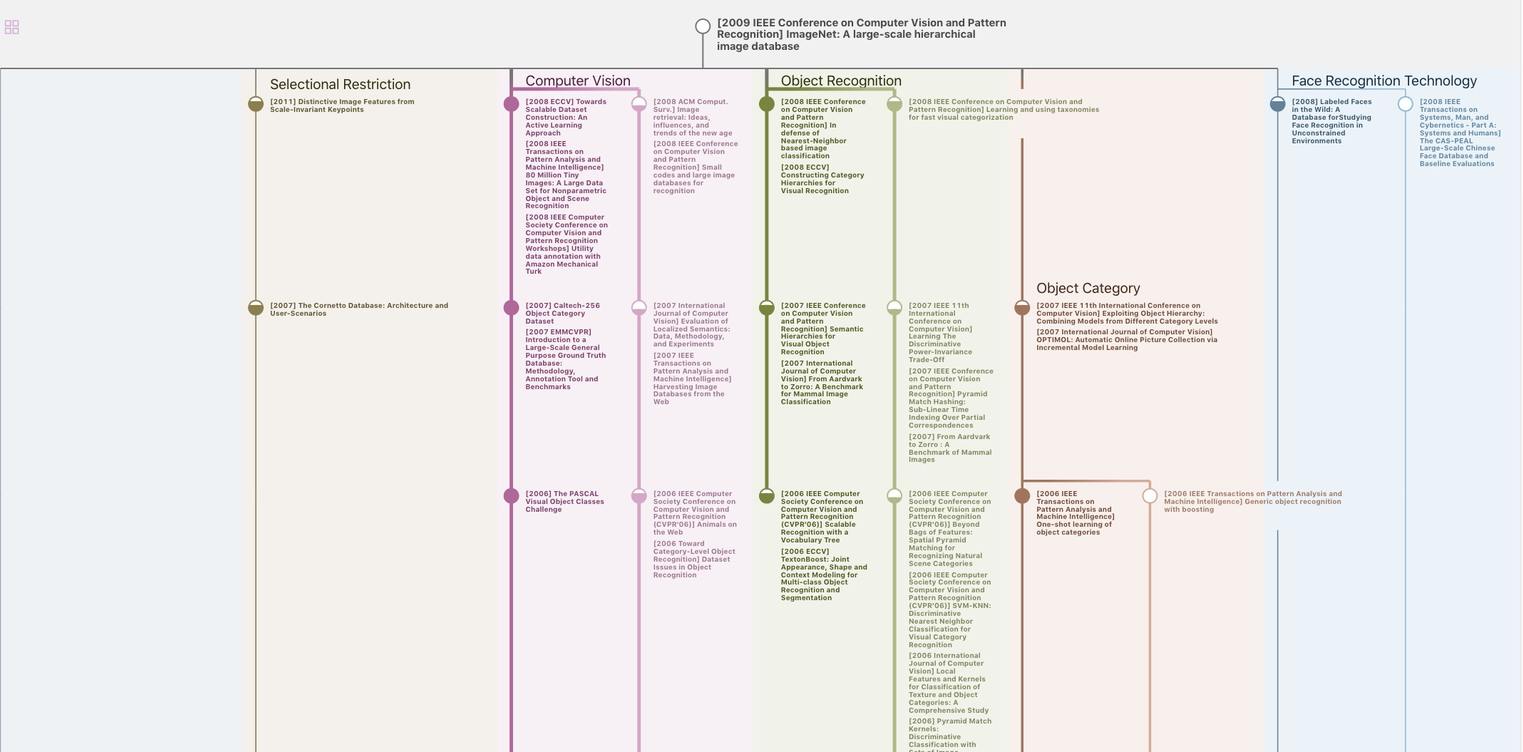
生成溯源树,研究论文发展脉络
Chat Paper
正在生成论文摘要