Data-Driven Operator Theoretic Methods For Global Phase Space Learning
2020 AMERICAN CONTROL CONFERENCE (ACC)(2020)
摘要
In this work, we developed new Koopman operator techniques to explore the global phase space of a nonlinear system from time-series data. In particular, we address the problem of identifying various invariant subsets of a phase space from the spectral properties of the associated Koopman operator constructed from time-series data. Moreover, in the case when the system evolution is known locally in various invariant subspaces, then a phase space stitching result is proposed that can be applied to identify a global Koopman operator. A biological system, the bistable toggle switch is considered to illustrate the proposed results. The construction of this global Koopman operator is very helpful in experimental works as multiple experiments cannot be performed at the same time starting from several initial conditions.
更多查看译文
关键词
data-driven operator theoretic methods,global phase space learning,Koopman operator techniques,nonlinear system,time-series data,invariant subsets,associated Koopman operator,system evolution,invariant subspaces,phase space stitching result,global Koopman operator,biological system
AI 理解论文
溯源树
样例
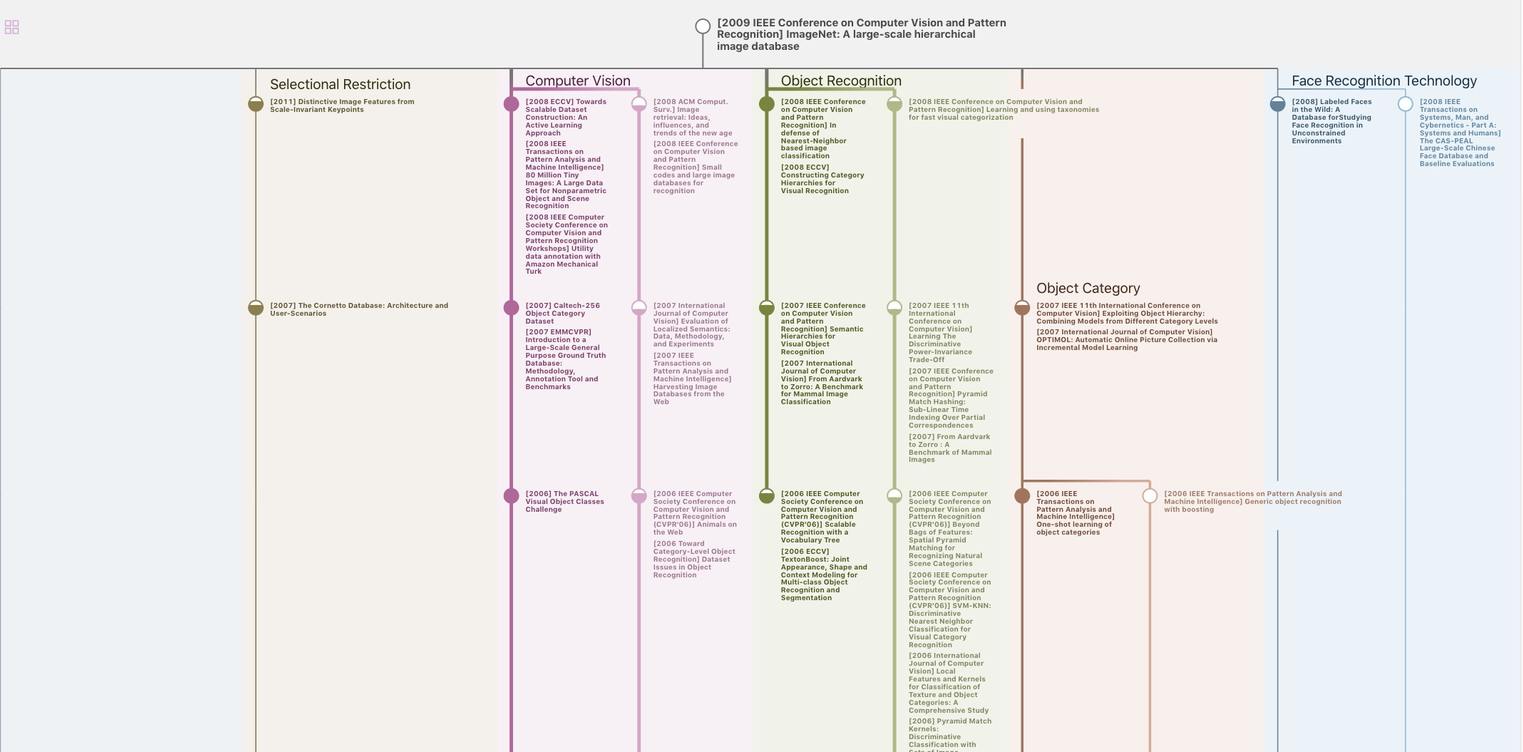
生成溯源树,研究论文发展脉络
Chat Paper
正在生成论文摘要