Learning nonlinear operators via DeepONet based on the universal approximation theorem of operators
NATURE MACHINE INTELLIGENCE(2021)
摘要
It is widely known that neural networks (NNs) are universal approximators of continuous functions. However, a less known but powerful result is that a NN with a single hidden layer can accurately approximate any nonlinear continuous operator. This universal approximation theorem of operators is suggestive of the structure and potential of deep neural networks (DNNs) in learning continuous operators or complex systems from streams of scattered data. Here, we thus extend this theorem to DNNs. We design a new network with small generalization error, the deep operator network (DeepONet), which consists of a DNN for encoding the discrete input function space (branch net) and another DNN for encoding the domain of the output functions (trunk net). We demonstrate that DeepONet can learn various explicit operators, such as integrals and fractional Laplacians, as well as implicit operators that represent deterministic and stochastic differential equations. We study different formulations of the input function space and its effect on the generalization error for 16 different diverse applications.
更多查看译文
关键词
nonlinear operators,universal approximation theorem,deeponet
AI 理解论文
溯源树
样例
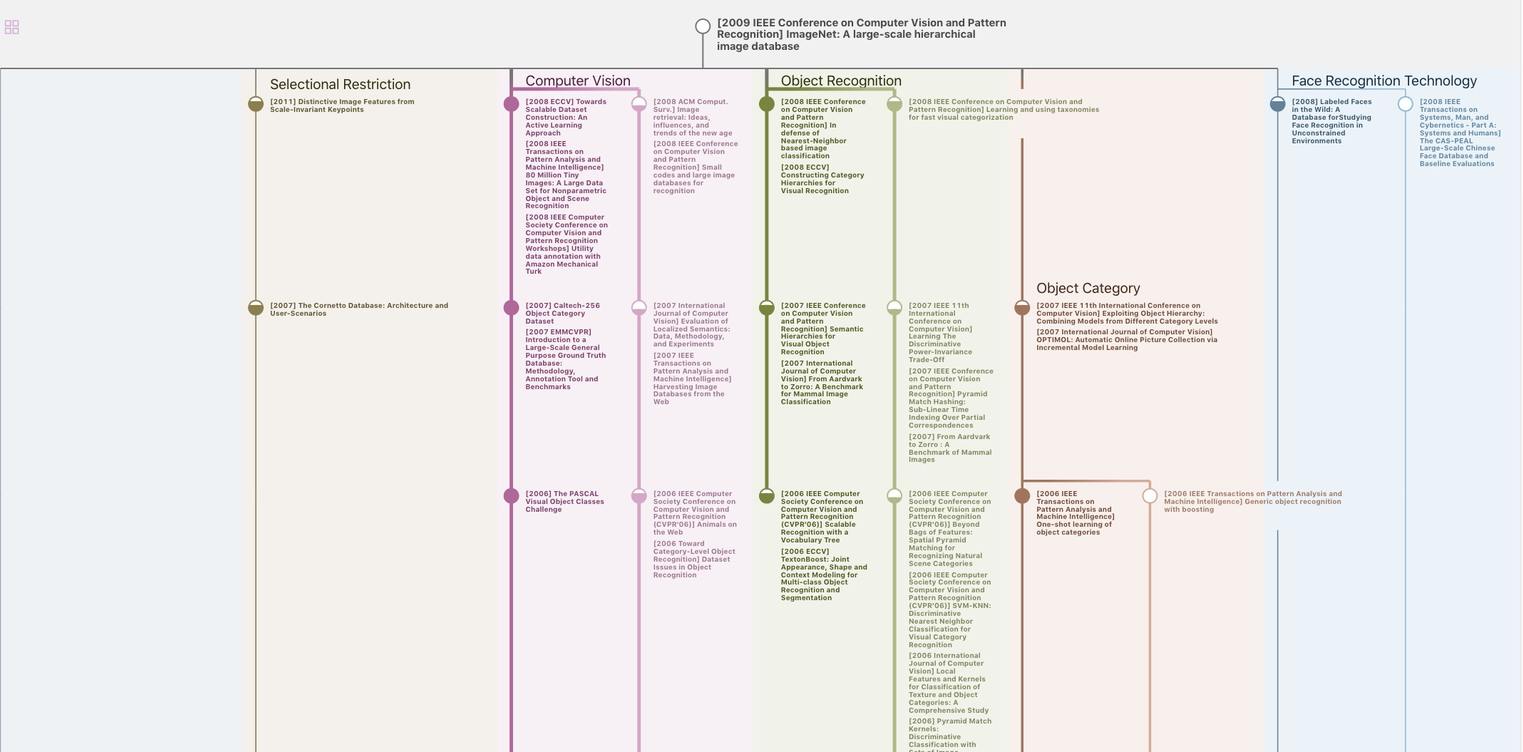
生成溯源树,研究论文发展脉络
Chat Paper
正在生成论文摘要