Structure Characteristic-Aware Pruning Strategy for Convolutional Neural Networks.
HPCC/SmartCity/DSS(2019)
摘要
Convolutional Neural Networks have received considerable attention over the past few years, and they are widely used in various fields. However, the computational complexity and excessive storage space caused by over-parameterized of these networks are also being serious. This will limit further development of deep learning in resource-constrained devices such as the mobile terminal. It has been proposed that pruning is a useful method to optimize networks, whereas there is no contribution focus on utilizing the traits of the network to guide pruning. The primary purpose of this paper is to research the different effects of layers with varying depth and the different number of parameters during the pruning process. By taking advantage of the characteristics of network structure itself, we propose a new pruning strategy with three variants to effectively prune CNNs which can automatically determine the most appropriate sparsity for each conv-layer. Using iterative pruning and retraining, we evaluated our strategy on InceptionV3 and ResNet50. We analyzed our three variants and made a comparison between each other.
更多查看译文
关键词
Convolutional Neural Network, Pruning, Structure characteristic, Image classification, Object detection
AI 理解论文
溯源树
样例
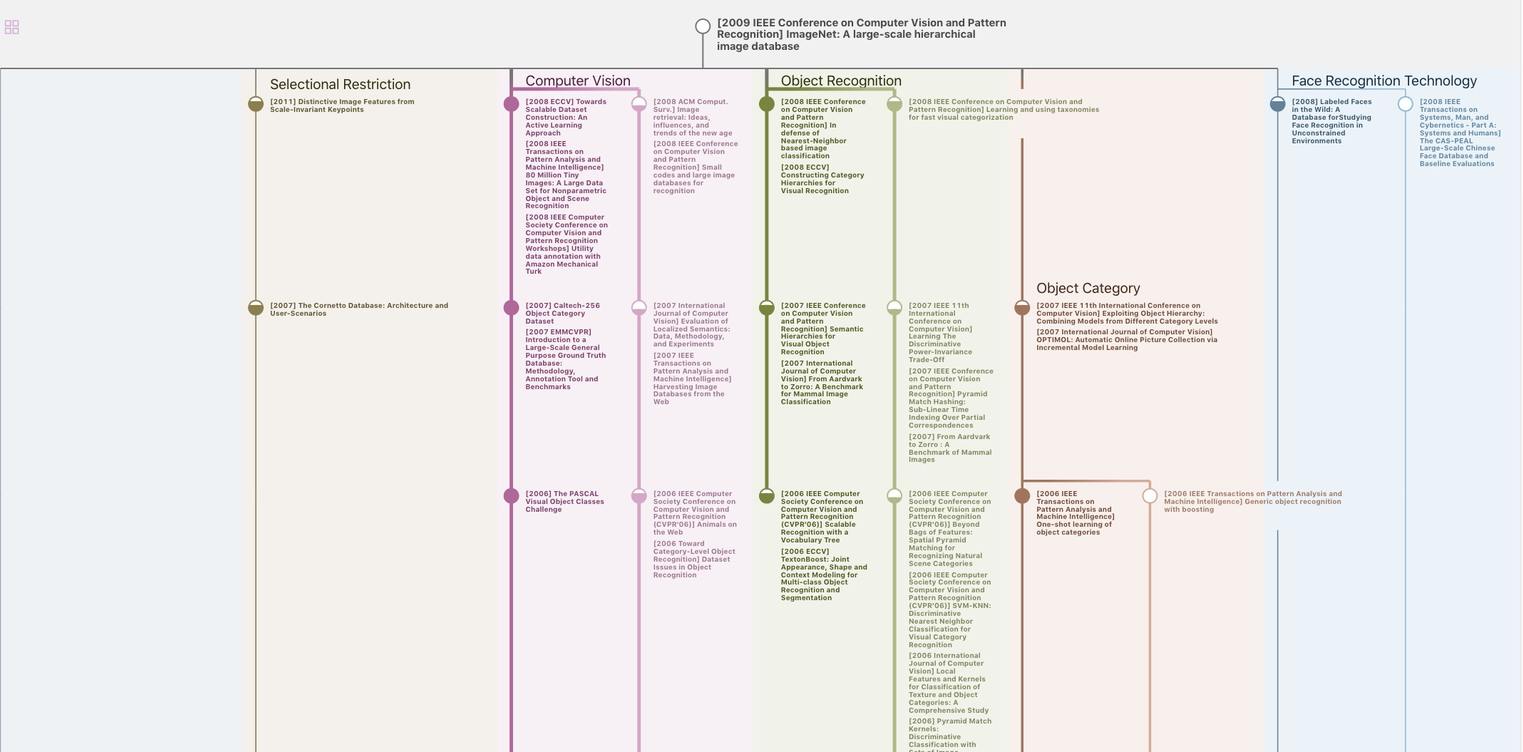
生成溯源树,研究论文发展脉络
Chat Paper
正在生成论文摘要