1248-P: Estimating Diabetes Duration from Electronic Medical Records (EMRs) with an Intrinsic Diabetes Risk Prediction Model: From Clinical Trial to Real-World Clinical Practice
Diabetes(2019)
摘要
We have previously described the utility and high predictive value of the Building, Relating, Assessing, and Validating Outcomes (BRAVO) model, which was developed from the Action to Control Cardiovascular Risk in Diabetes (ACCORD) trial data. However, it uses diabetes duration as a key factor for modelling diabetes progression, and diabetes duration is not routinely collected in EMRs. This study use machine learning to estimate diabetes duration with EMR information. Among individuals (N=8,026) with complete records from the ACCORD trial, diabetes duration was estimated with an ordinary least squares (OLS) model, a least absolute shrinkage and selection operator (LASSO) model, a pruned decision tree model, and a random forest model using 37 baseline predictors such as biomarkers, demographics, disease history, and medications. Model performance was compared using root-mean-square Error (RMSE), mean-absolute error (MAS), and R-squared. All three indicators were calculated through 10-fold cross-validation with 1000 iterations in order to address over-fitting. Age, current HbA1c value, retinopathy, number of oral antidiabetic drugs, number of Insulin types, and use of sulfonylureas were identified as key predictors for patient-reported diabetes duration. The random forest model achieved the best performance on all three indicators (RMSE=6.16, MAE= 4.71, R2=0.30), significantly higher than the pruned decision tree model (RMSE=6.57, MAE= 5.03, R2=0.21) and LASSO model (RMSE=6.60, MAE= 5.08, R2=0.20). Diabetes duration can be predicted using fields routinely included in EMRs. Integration of the results of this prediction equation into predictive diabetes models (such as BRAVO) will allow patient-specific estimates of diabetes duration based solely on EMRs. This will increase precision when inferring risk level and using models to aid clinical decision making among patients with type 2 diabetes. Disclosure H. Shao: None. S. Yang: None. C. Stoecker: None. V. Fonseca: Board Member; Self; American Association of Clinical Endocrinologists. Consultant; Self; Abbott, Asahi Kasei Corporation, Novo Nordisk Inc., Sanofi US, Takeda Pharmaceutical Company Limited. Research Support; Self; Bayer US. Stock/Shareholder; Self; Amgen Inc., BRAVO4HEALTH, Mellitus Health. X. Cheng: None. L. Shi: None.
更多查看译文
AI 理解论文
溯源树
样例
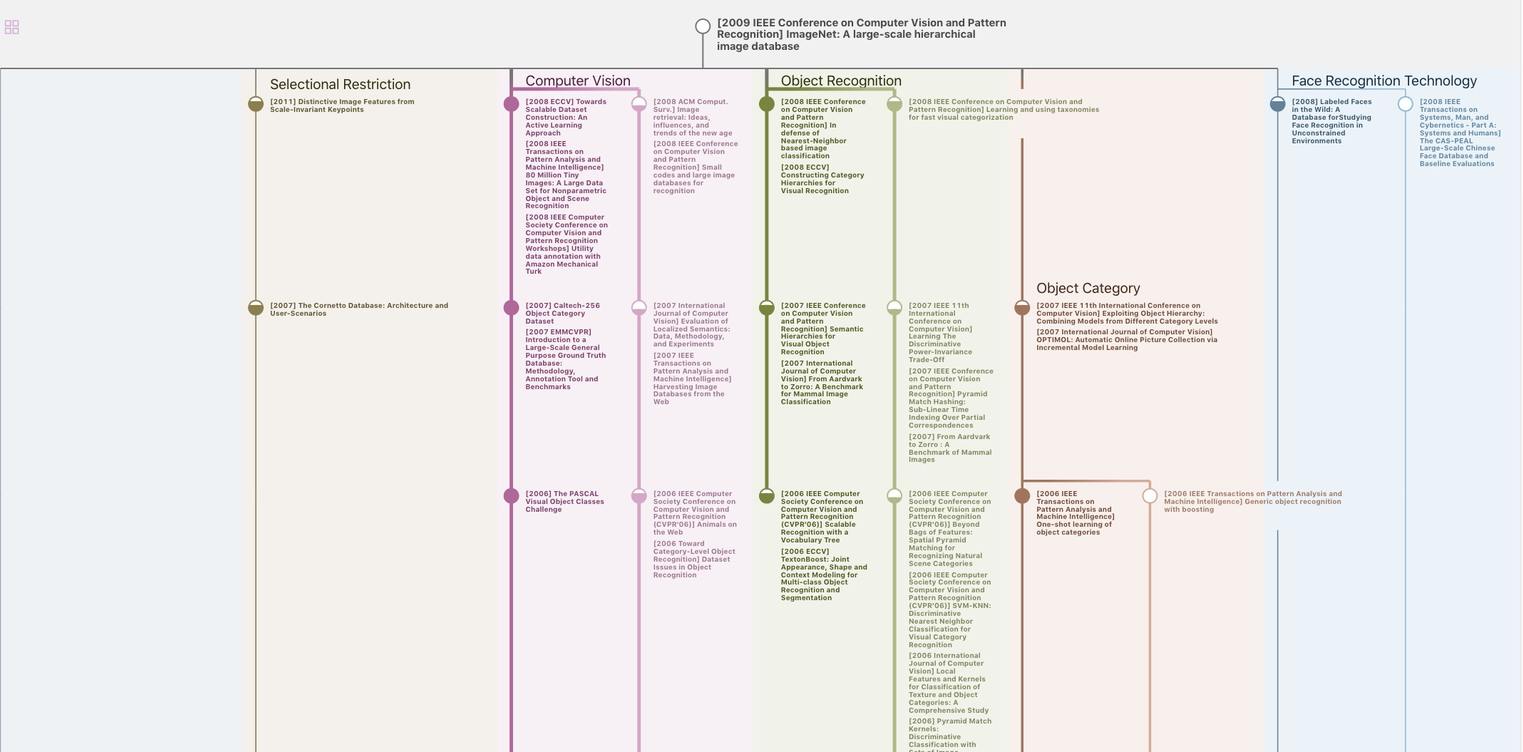
生成溯源树,研究论文发展脉络
Chat Paper
正在生成论文摘要