Deep joint two-stream Wasserstein auto-encoder and selective attention alignment for unsupervised domain adaptation
NEURAL COMPUTING & APPLICATIONS(2019)
摘要
Domain adaptation refers to the process of utilizing the labeled source domain data to learn a model that can perform well in the target domain with limited or missing labels. Several domain adaptation methods combining image translation and feature alignment have been recently proposed. However, there are two primary drawbacks of such methods. First, the majority of the methods assume that synthetic target images have the same distribution as real target images, and thus, only the synthetic target images are employed for training the target classifier, which makes the model’s performance significantly dependent on the quality of the generated images. Second, most of the methods blindly align the discriminative content information by merging spatial and channel-wise information, thereby ignoring the relationships among channels. To address these issues, a two-step approach that joints two-stream Wasserstein auto-encoder (WAE) and selective attention (SA) alignment, named J2WSA, is proposed in this study. In the pre-training step, the two-stream WAE is employed for mapping the four domains to a shared nice manifold structure by minimizing the Wasserstein distance between the distribution of each domain and the corresponding prior distribution. During the fine-tuning step, the SA alignment model initialized by the two-stream WAE is applied for automatically selecting the style part of channels for alignment, while simultaneously suppressing the content part alignment using the SA block. Extensive experiments indicate that the combination of the aforementioned two models can achieve state-of-the-art performance on the Office-31 and digital domain adaptation benchmarks.
更多查看译文
关键词
Domain adaptation,Two-stream Wasserstein auto-encoder,Selective attention alignment
AI 理解论文
溯源树
样例
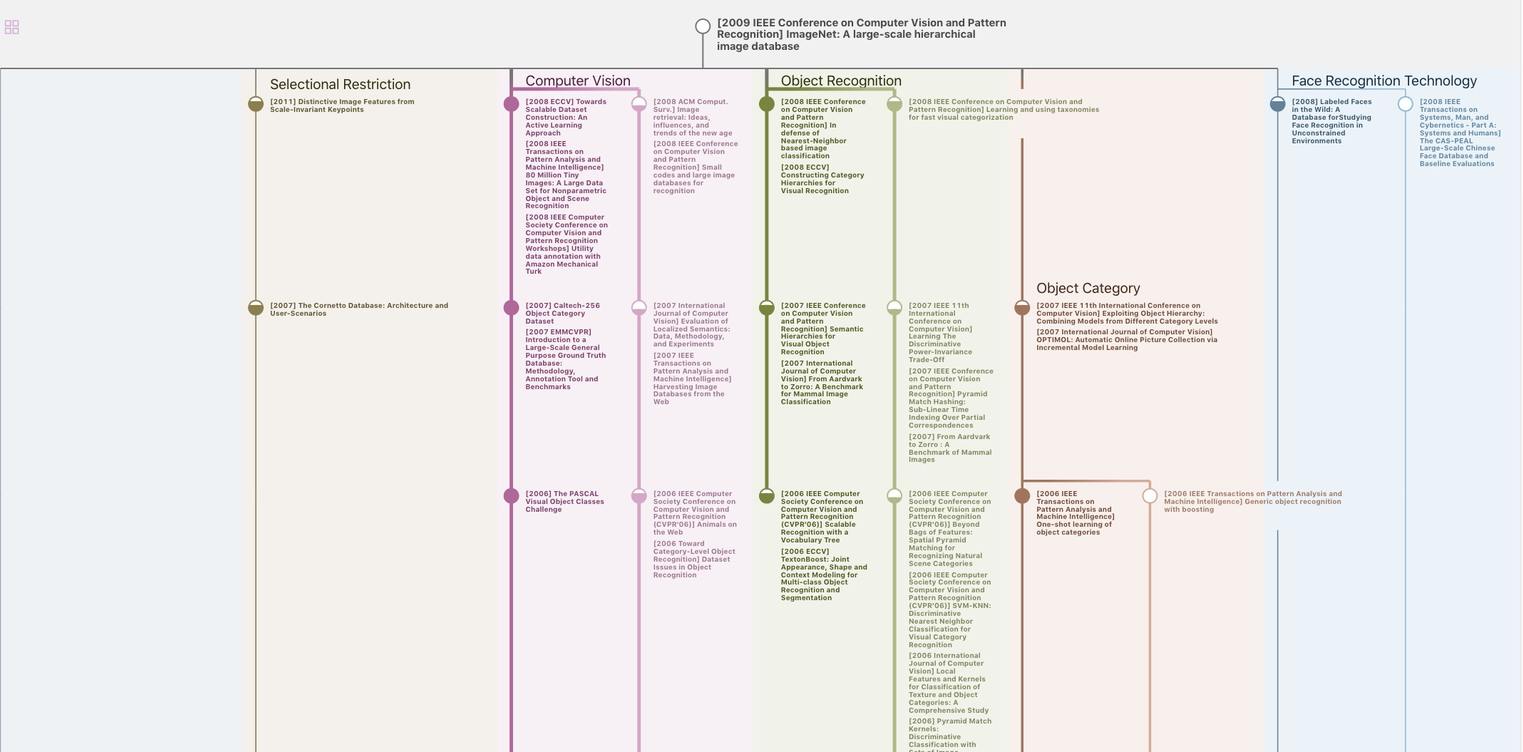
生成溯源树,研究论文发展脉络
Chat Paper
正在生成论文摘要