Locally Balanced Inductive Matrix Completion for Demand-Supply Inference in Stationless Bike-Sharing Systems
IEEE Transactions on Knowledge and Data Engineering(2020)
摘要
Stationless bike-sharing systems such as Mobike are currently becoming extremely popular in China as well as some other big cities in the world. Compared to traditional bicycle-sharing systems, stationless bike-sharing systems do not need bike stations. Users can rent and return bikes at arbitrary locations through an App installed on their smart phones. Such a convenient and flexible bike-sharing mode greatly solves the last mile issue of the commuters, and better meets their real bike usage demand. However, it also poses new challenges for operators to manage the system. The first primary challenge is how to accurately estimate the real bike usage demand in different areas of a city and in different time intervals, which is crucial for the system planning and operation. This paper for the first time proposes a data driven approach for bike usage demand inference in stationless bike-sharing systems. The idea is that we first estimate the demands in some regions and time intervals from a small number of observed bike check-out/in data directly, and then use them as seeds to infer the region-level bike usage demands of an entire city. Specifically, we formulate this problem as a matrix completion task by modeling the bike usage demand as a matrix whose two dimensions are time intervals of a day and regions of a city, respectively. With the observation that POI distribution of a region is an important indicator to bike demand, we propose to utilize inductive matrix factorization by considering POIs as side information. As the bike usage data are highly correlated in both spatial and temporal dimensions, we also incorporate the spatial-temporal correlations as well as the balanced bike usage constraint into a joint optimization framework. We evaluate the proposed model on a large Mobike trip dataset collected from Beijing, and the experimental results show its superior performance by comparison with various baseline methods.
更多查看译文
关键词
Bike-sharing system,demand inference,matrix completion,optimization
AI 理解论文
溯源树
样例
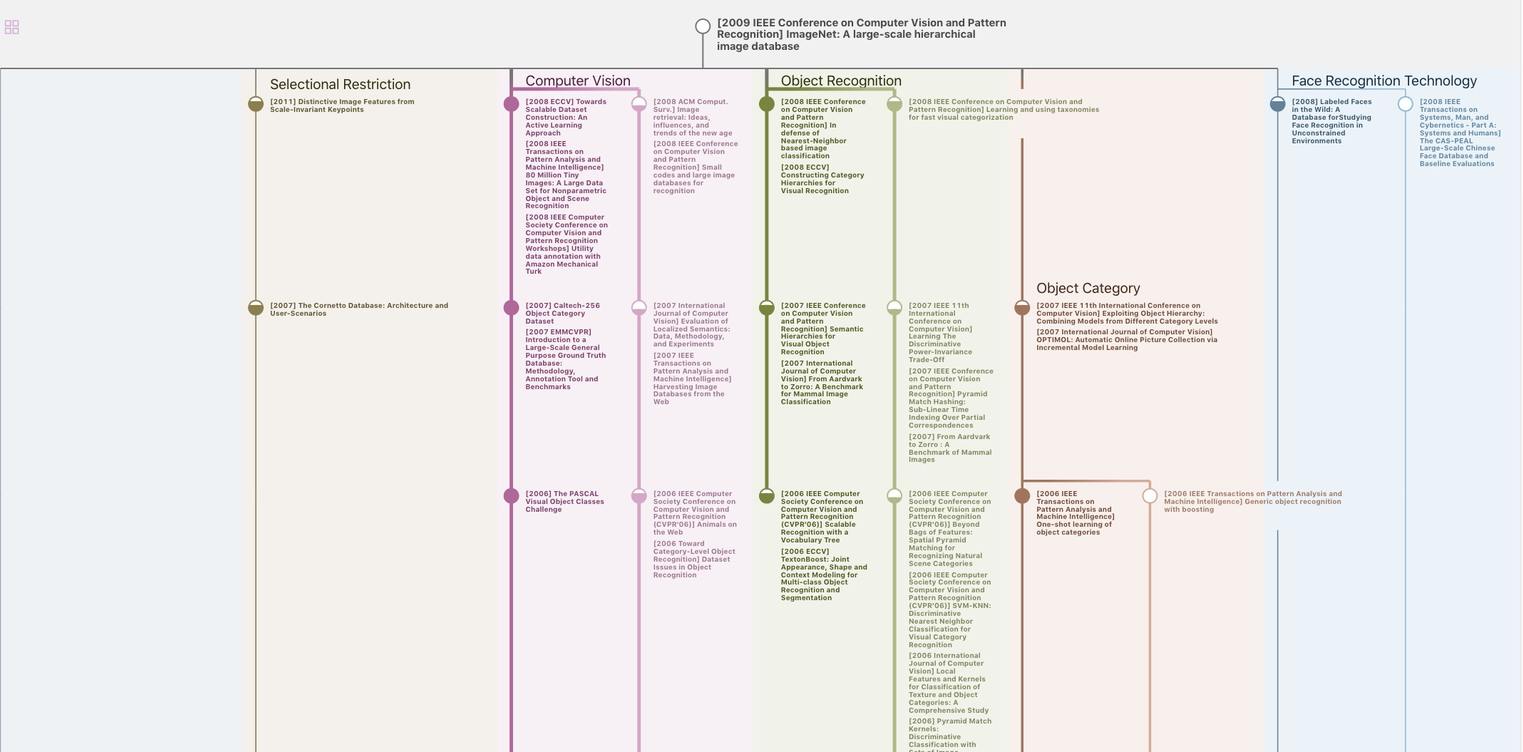
生成溯源树,研究论文发展脉络
Chat Paper
正在生成论文摘要