Predicting Partisan Responsiveness: A Probabilistic Text Mining Time-Series Approach
POLITICAL ANALYSIS(2020)
摘要
When do parties respond to their political rivals and when do they ignore them? This article presents a new computational framework to detect, analyze and predict partisan responsiveness by showing when parties on opposite poles of the political spectrum react to each other's agendas and thereby contribute to polarization. Once spikes in responsiveness are detected and categorized using latent Dirichlet allocation, we utilize the terms that comprise the topics, together with a gradient descent solver, to assess the classifier's predictive accuracy. Using 10,597 documents from the official websites of radical right and ethnic political parties in Slovakia (2004-2014), the analysis predicts which political issues will elicit partisan reactions, and which will be ignored, with an accuracy of 83% (F-measure) and outperforms both Random Forest and Naive Bayes classifiers. Subject matter experts validate the approach and interpret the results.
更多查看译文
关键词
forecasting,automated content analysis,natural language processing,probabilistic topic modeling,sparse learning,political parties,polarization
AI 理解论文
溯源树
样例
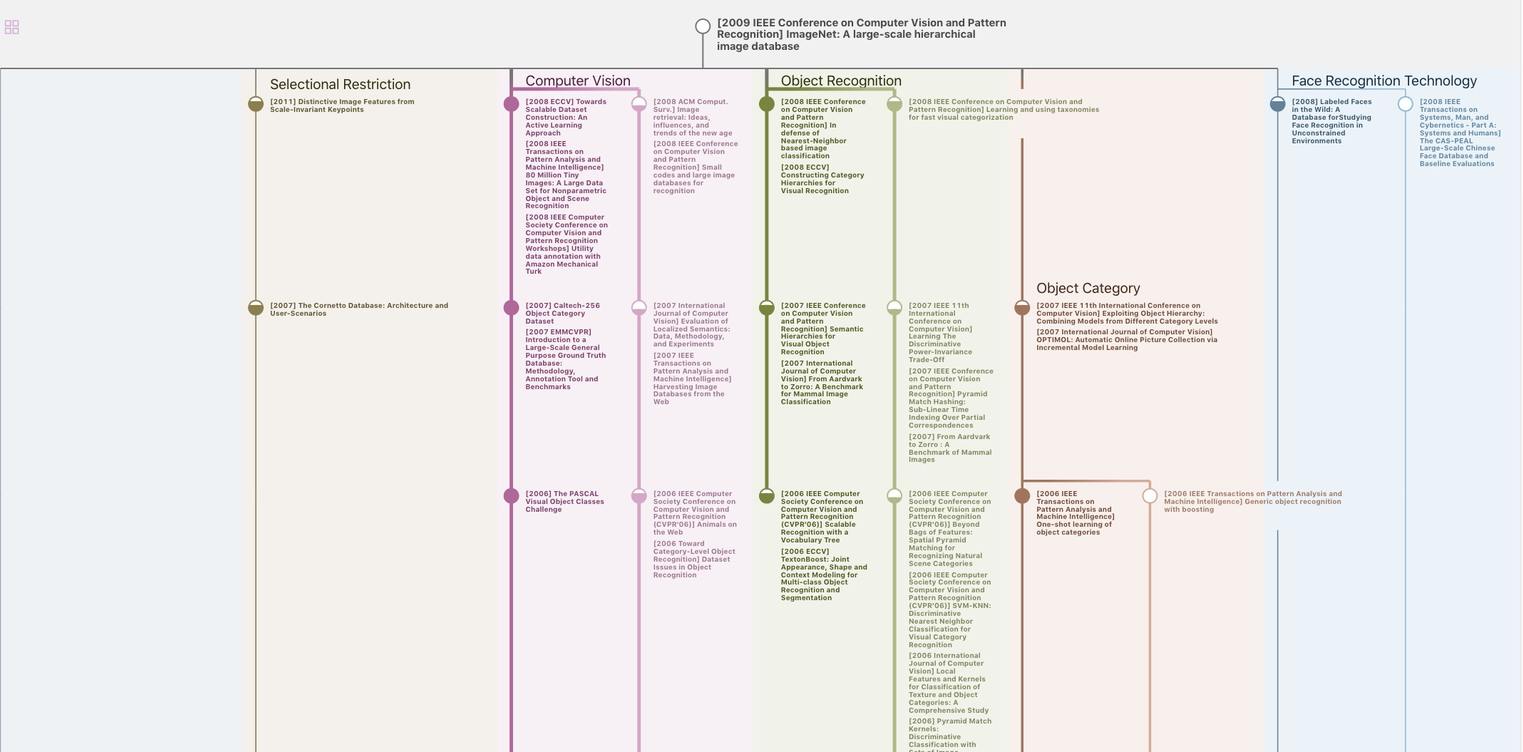
生成溯源树,研究论文发展脉络
Chat Paper
正在生成论文摘要