Anisotropic functional deconvolution with long-memory noise: the case of a multi-parameter fractional Wiener sheet
JOURNAL OF NONPARAMETRIC STATISTICS(2019)
摘要
We look into the minimax results for the anisotropic two-dimensional functional deconvolution model with the two-parameter fractional Gaussian noise. We derive the lower bounds for the -risk, , and taking advantage of the Riesz poly-potential, we apply a wavelet-vaguelette expansion to de-correlate the anisotropic fractional Gaussian noise. We construct an adaptive wavelet hard-thresholding estimator that attains asymptotically optimal or quasi-optimal convergence rates in a wide range of Besov balls. Such convergence rates depend on a delicate balance between the parameters of the Besov balls, the degree of ill-posedness of the convolution operator and the parameters of the fractional Gaussian noise under regular-smooth convolution, whereas the rates are not affected by long-memory under super-smooth convolution. A limited simulations study confirms the theoretical claims of the paper. The proposed approach is extended to the general r-dimensional case, with r>2, and the corresponding convergence rates do not suffer from the curse of dimensionality.
更多查看译文
关键词
Anisotropic functional deconvolution,Besov space,anisotropic fractional Brownian sheet,minimax convergence rate
AI 理解论文
溯源树
样例
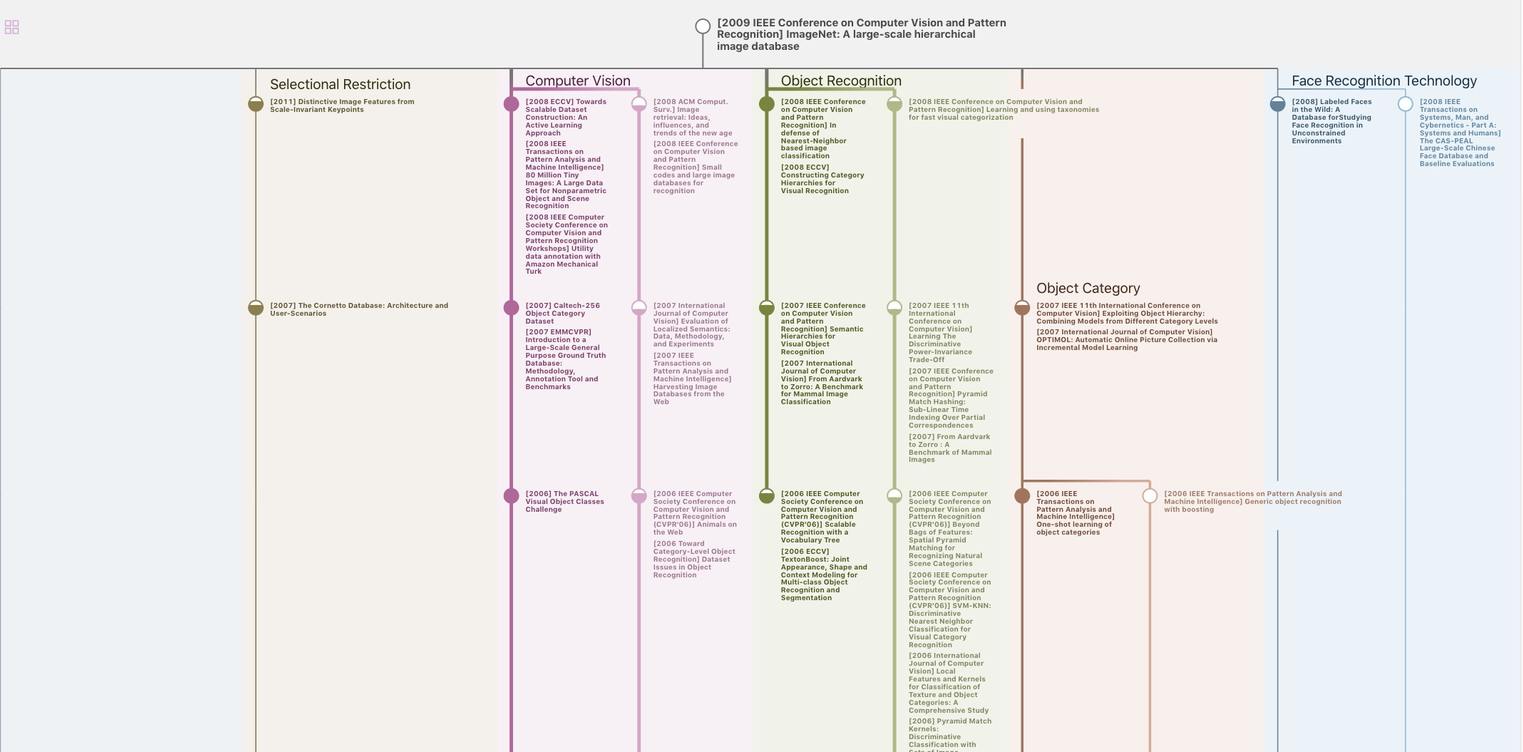
生成溯源树,研究论文发展脉络
Chat Paper
正在生成论文摘要