Analysis of Factors Affecting Real-Time Ridesharing Vehicle Crash Severity
SUSTAINABILITY(2019)
摘要
The popular real-time ridesharing service has promoted social and environmental sustainability in various ways. Meanwhile, it also brings some traffic safety concerns. This paper aims to analyze factors affecting real-time ridesharing vehicle crash severity based on the classification and regression tree (CART) model. The Chicago police-reported crash data from January to December 2018 is collected. Crash severity in the original dataset is highly imbalanced: only 60 out of 2624 crashes are severe injury crashes. To fix the data imbalance problem, a hybrid data preprocessing approach which combines the over- and under-sampling is applied. Model results indicate that, by resampling the crash data, the successfully predicted severe crashes are increased from 0 to 40. Besides, the G-mean is increased from 0% to 73%, and the AUC (area under the receiver operating characteristics curve) is increased from 0.73 to 0.82. The classification tree reveals that following variables are the primary indicators of real-time ridesharing vehicle crash severity: pedestrian/pedalcyclist involvement, number of passengers, weather condition, trafficway type, vehicle manufacture year, traffic control device, driver gender, lighting condition, vehicle type, driver age and crash time. The current study could provide some valuable insights for the sustainable development of real-time ridesharing services and urban transportation.
更多查看译文
关键词
real-time ridesharing,crash severity,data imbalance,SMOTE plus ENN,decision tree
AI 理解论文
溯源树
样例
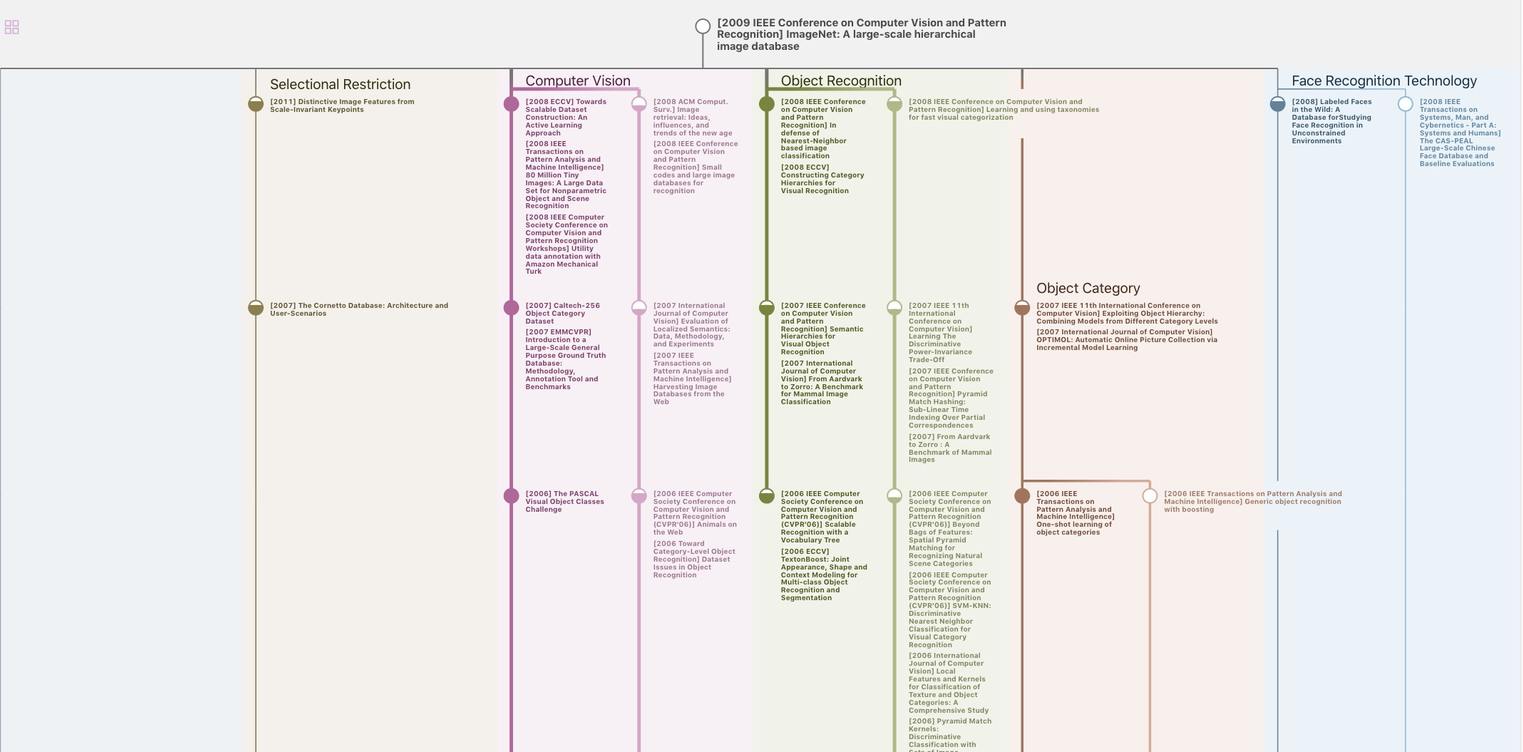
生成溯源树,研究论文发展脉络
Chat Paper
正在生成论文摘要