Empowering graph segmentation methods with SOMs and CONN similarity for clustering large and complex data
NEURAL COMPUTING & APPLICATIONS(2019)
摘要
High-dimensional, large, and noisy data with complex structure challenge the limits of many clustering algorithms including modern graph segmentation methods. SOM-based clustering has been shown capable of capturing many clusters of widely varying statistical properties in such data. However, to date the best discovery results are produced by interactive extraction of clusters from informative SOM visualizations. This does not scale for Big Data, large archives, or near-real-time analyses. We approach this challenge by infusing SOM knowledge into leading automatic graph segmentation algorithms, which produce extremely poor results when segmenting the SOM prototypes without this information, and which would take a prohibitively long time to segment the input data sets. The knowledge translation occurs by casting the SOM prototypes as vertices and the CONN similarity measure as edge weightings of a graph which is then presented to graph segmentation algorithms. The resulting performance closely approximates the precision of the interactive SOM segmentation for complicated data and, at the same time, is extremely fast and memory-efficient. We demonstrate the effectiveness on a simple synthetic data set and on a very realistic fully labeled synthetic hyperspectral image. We also examine performance dependence on available parametrizations of the graph segmentation algorithms, in combination with parametrizations of the CONN similarity measure.
更多查看译文
关键词
SOM clustering, Graph segmentation, CONN similarity, Big Data, Automation
AI 理解论文
溯源树
样例
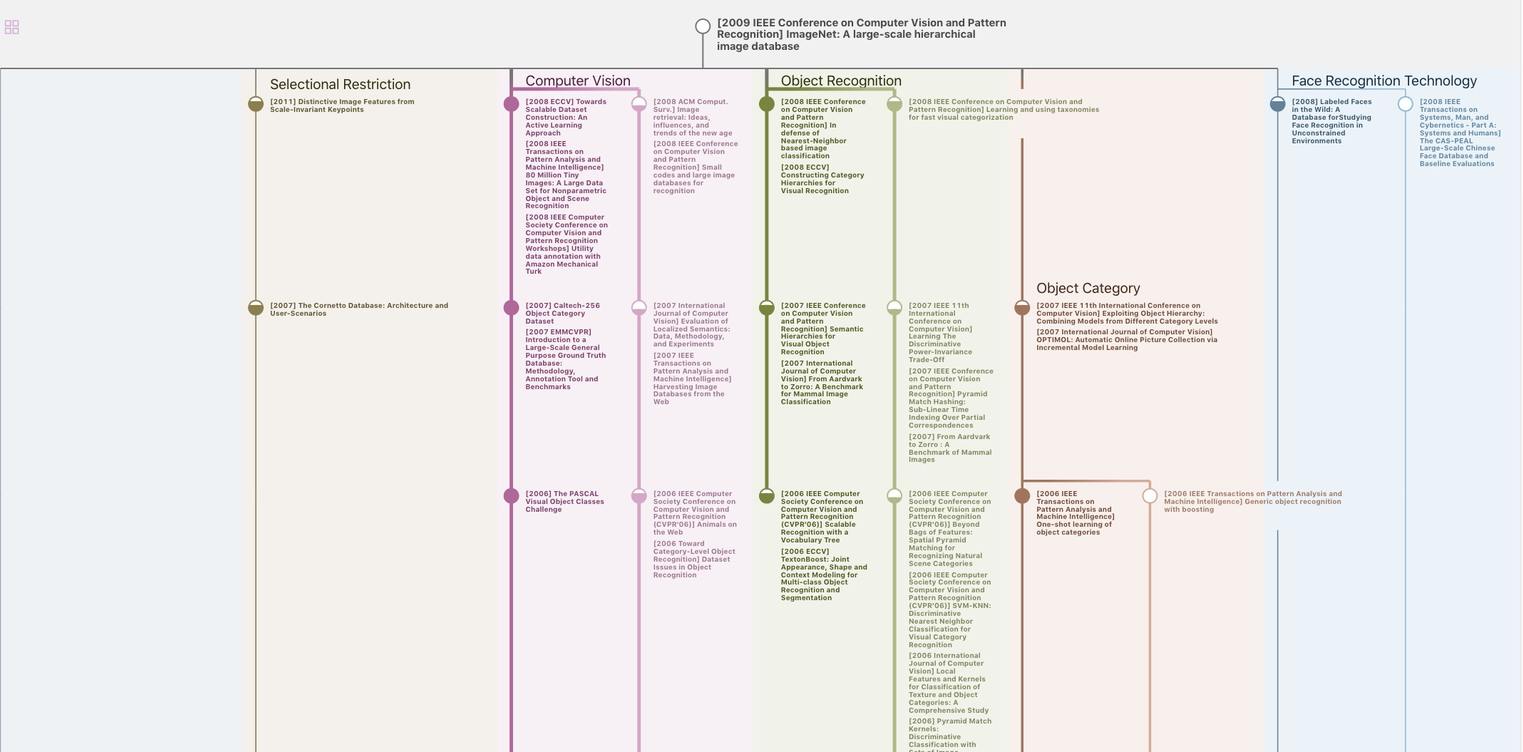
生成溯源树,研究论文发展脉络
Chat Paper
正在生成论文摘要