A Transparent Framework For Evaluating Unintended Demographic Bias In Word Embeddings
57TH ANNUAL MEETING OF THE ASSOCIATION FOR COMPUTATIONAL LINGUISTICS (ACL 2019)(2019)
摘要
Word embedding models have gained a lot of traction in the Natural Language Processing community, however, they suffer from unintended demographic biases. Most approaches to evaluate these biases rely on vector space based metrics like the Word Embedding Association Test (WEAT). While these approaches offer great geometric insights into unintended biases in the embedding vector space, they fail to offer an interpretable meaning for how the embeddings could cause discrimination in downstream NLP applications. In this work, we present a transparent framework and metric for evaluating discrimination across protected groups with respect to their word embedding bias. Our metric (Relative Negative Sentiment Bias, RNSH) measures fairness in word embeddings via the relative negative sentiment associated with demographic identity terms from various protected groups. We show that our framework and metric enable useful analysis into the bias in word embeddings.
更多查看译文
关键词
Natural Language Processing,Language Modeling,Word Representation,Named Entity Recognition,Topic Modeling
AI 理解论文
溯源树
样例
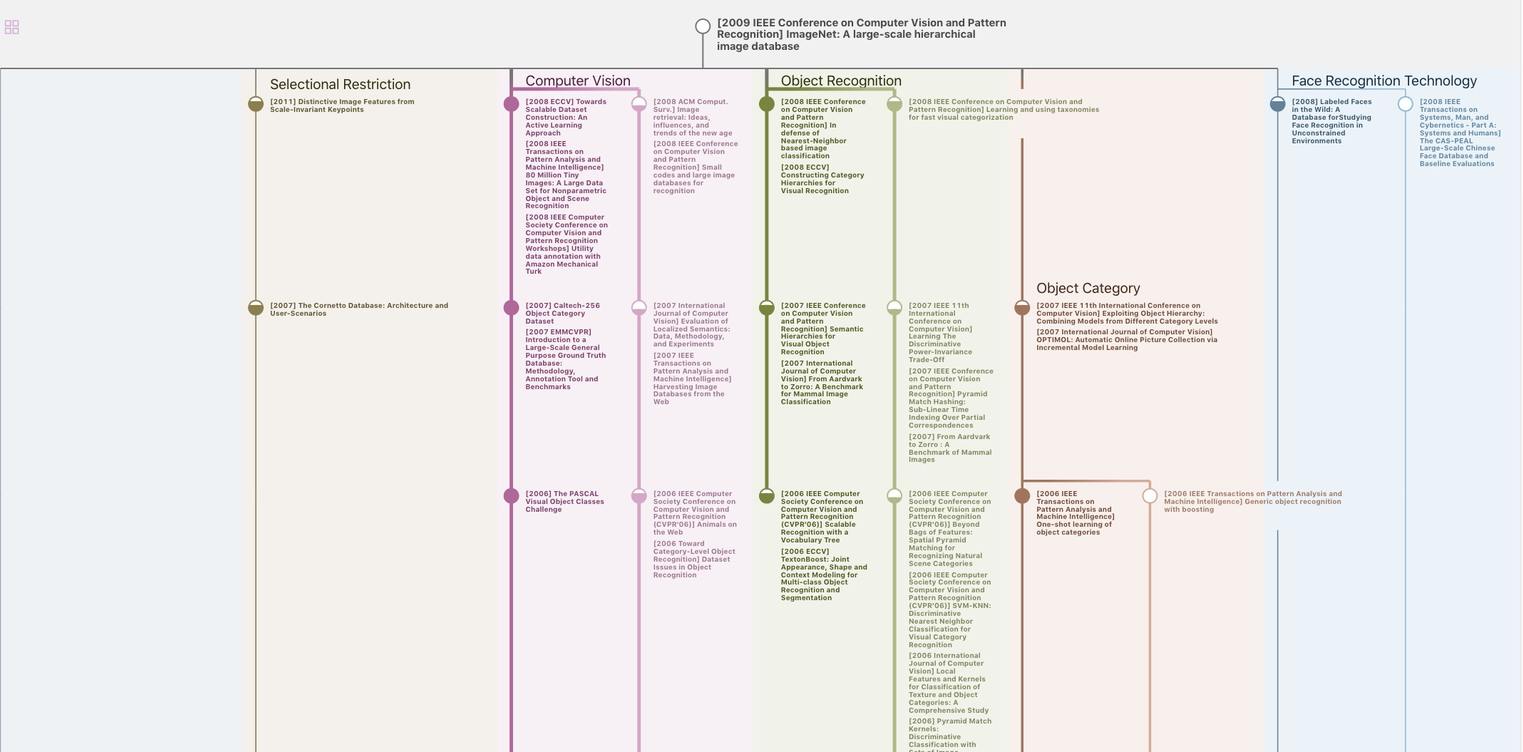
生成溯源树,研究论文发展脉络
Chat Paper
正在生成论文摘要