Hypothesis Testing for Multiple Mean and Correlation Curves with Functional Data
STATISTICA SINICA(2020)
摘要
Existing statistical methods for functional data analyses tend to use local smoothing estimators or some known basis approximations. In many applications with functional observations, the main objectives of statistical inferences are to test (a) the equivalence of a set of unknown mean curves, and (b) the correlation curves of the unknown stochastic processes. However, the unknown curves of the functional data might not be approximated by a set of known basis functions. We propose a class of simple test statistics for comparing the mean and correlation curves of functional data, without relying on an estimation using local smoothing or basis approximations, and study their basic asymptotic properties. Then, we apply the proposed method to functional gene expression data, showing that it yields practical and meaningful results with minimal assumptions. Numerical justifications of our testing method are provided by a simulation study.
更多查看译文
关键词
Comparing mean curves,correlation curve,functional data,gene expression profile,testing functional equivalence,uniform normed convergence
AI 理解论文
溯源树
样例
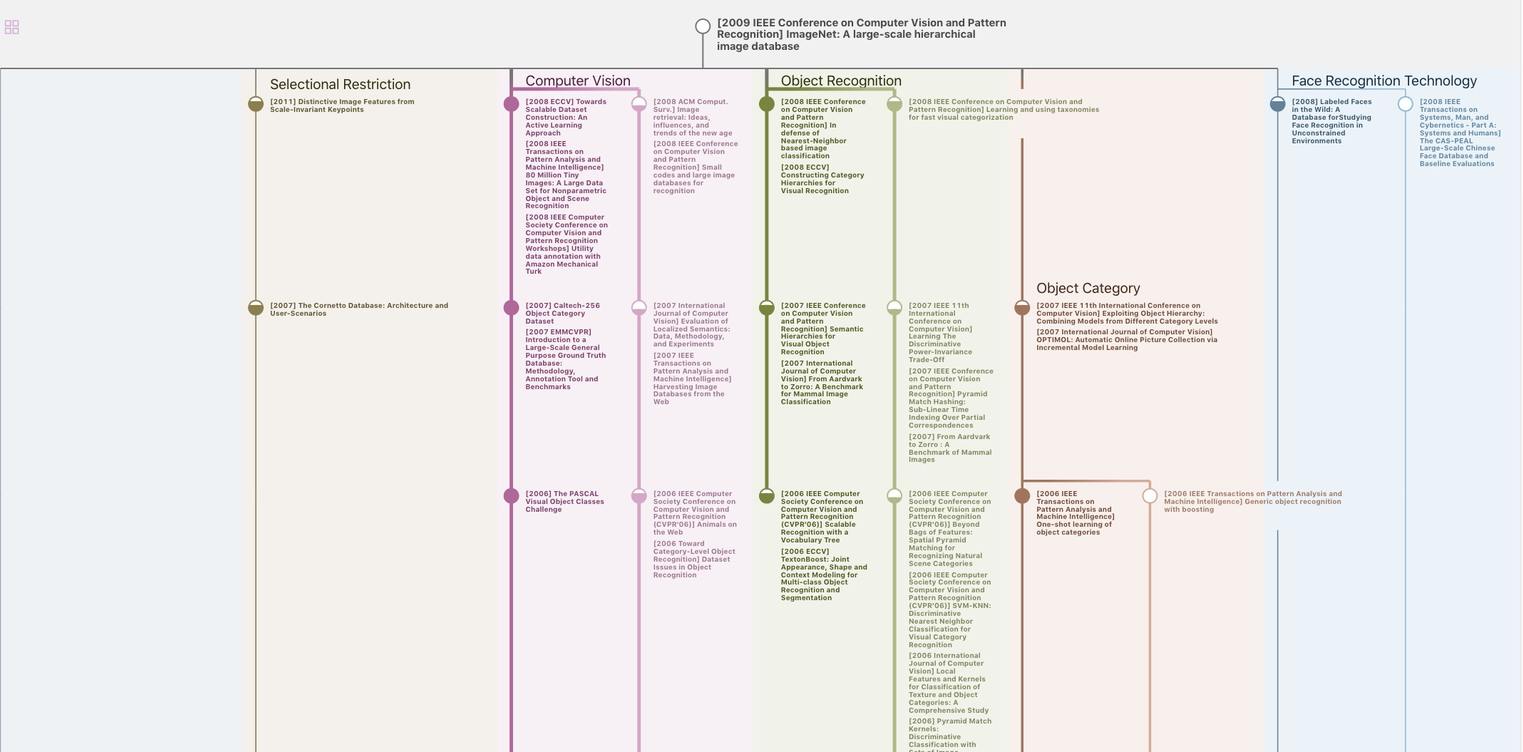
生成溯源树,研究论文发展脉络
Chat Paper
正在生成论文摘要