Semantics of the Black-Box: Can Knowledge Graphs Help Make Deep Learning Systems More Interpretable and Explainable?
IEEE Internet Computing(2021)
摘要
The recent series of innovations in deep learning (DL) have shown enormous potential to impact individuals and society, both positively and negatively. DL models utilizing massive computing power and enormous datasets have significantly outperformed prior historical benchmarks on increasingly difficult, well-defined research tasks across technology domains such as computer vision, natural language processing, and human-computer interactions. However, DL's black-box nature and over-reliance on massive amounts of data condensed into labels and dense representations pose challenges for interpretability and explainability. Furthermore, DLs have not proven their ability to effectively utilize relevant domain knowledge critical to human understanding. This aspect was missing in early data-focused approaches and necessitated knowledge-infused learning (K-iL) to incorporate computational knowledge. This article demonstrates how knowledge, provided as a knowledge graph, is incorporated into DL using K-iL. Through examples from natural language processing applications in healthcare and education, we discuss the utility of K-iL towards interpretability and explainability.
更多查看译文
关键词
DL models utilizing massive computing power,deep learning,semantics,natural language processing applications,knowledge graph,computational knowledge,necessitated knowledge-infused learning,early data-focused approaches,human understanding,relevant domain knowledge,explainability,dense representations,black-box nature,human-computer interactions,computer vision,well-defined research tasks,prior historical benchmarks,enormous datasets
AI 理解论文
溯源树
样例
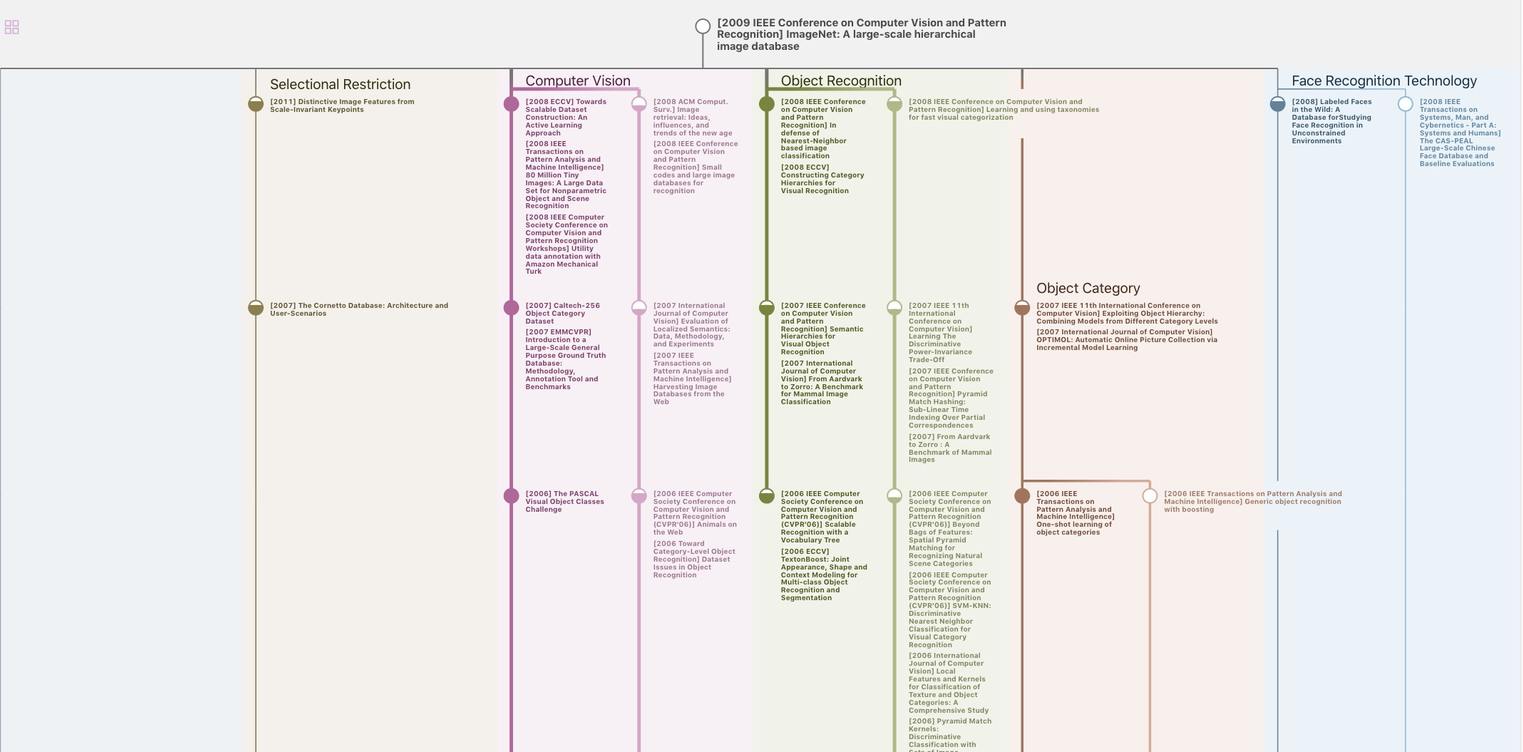
生成溯源树,研究论文发展脉络
Chat Paper
正在生成论文摘要