Abstract 4059: Identification of Pre-Existing Neoantigen-Specific T Cells in Patients Receiving Checkpoint Inhibitor Therapy Using a Deep Learning Antigen Prediction Model
Clinical Research (Excluding Clinical Trials)(2019)
摘要
Immune Checkpoint Blockade (ICB) has revolutionized the treatment of patients with cancer but provides durable clinical benefit to only a minority of patients. Neoantigens, antigens derived from tumor mutations, are emerging as key targets of T cells in patients with clinical responses to ICB whose tumors exhibit high mutational burden. T cells specific to some neoantigens appear to be naturally generated in these patients and can be further expanded upon ICB. Accurate neoantigen identification may fuel therapeutic advances by driving potent anti-neoantigen immune responses through therapeutic immunization or adoptive cell therapy. However, identification of neoantigens and neoantigen-reactive T cells remains a significant challenge. Current methods are time consuming and labor intensive and require non-routine clinical specimen collections. Here, we apply deep learning to a large HLA peptide and genomic dataset from NSCLC patients to create a computational model of minimal epitope presentation for neoantigen prediction. We demonstrate that our model allows rapid identification of neoantigens and neoantigen reactive T cells using routine clinical biopsies and blood specimens. This methodology does not require HLA-specific reagents and enables identification of T cell responses for every patient. To identify neoantigen-specific T cells, selected peptides, prioritized by our prediction model, were synthesized as minimal epitopes and added to short-term in vitro stimulation (IVS) cultures to expand neoantigen-reactive T cells. The presence of antigen-specific T cells was assessed two weeks later using IFN-gamma (IFN-g) ELISpot against pools of the prioritized neoantigens. Patient-specific peptide pools and IFN-g ELISpot enabled identification of neoantigen-reactive T cells in 5/9 (56%) patients on ICB. Deconvolution yielded an average of 2 recognized neoantigens per patient, with increased responses over time observed in one patient where multiple time points were available. Neoantigen responses were confirmed in repeat IVS cultures where additional samples were available. Importantly, expansion of HLA-matched healthy controls in the presence of patient peptide pools did not result in any responses by IFN-g ELISpot. In addition, antigen-reactive T cells were sorted for single cell sequencing on the 10x platform using CD137 as an HLA-independent T-cell activation marker. In summary, pre-existing neoantigen specific T cells and TCR clones can be rapidly identified utilizing routine clinical specimens, through (i) a powerful neoantigen prediction model, (ii) short-term in vitro T-cell expansion followed by IFN-gamma ELISpot, and (iii) HLA-independent selection and single cell sequencing. This methodology may allow rapid design of neoantigen-targeting vaccines or selection of TCRs for cell therapeutic approaches. Citation Format: Christine D. Palmer, Brendan Bulik, Aaron Yang, Meghan Hart, Matthew Davis, Joshua Francis, Fujiko Duke, Rita Zhou, Jennifer Busby, Tyler Murphy, Andrew Clark, Lauren Young, Mike Zhong, Kamilah Caldwell, Jesse Abhyankar, Alison Cook, Tommy Boucher, Raphaël Rousseau, Cynthia Voong, Naiyer Rizvi, Mark Frattini, Roman Yelensky, Karin Jooss, Mojca Skoberne. Identification of pre-existing neoantigen-specific T cells in patients receiving checkpoint inhibitor therapy using a deep learning antigen prediction model [abstract]. In: Proceedings of the American Association for Cancer Research Annual Meeting 2019; 2019 Mar 29-Apr 3; Atlanta, GA. Philadelphia (PA): AACR; Cancer Res 2019;79(13 Suppl):Abstract nr 4059.
更多查看译文
关键词
Tumor Antigen,Antigen Presentation,Cancer Immunotherapy,T Cell Immunity,Antibody Engineering
AI 理解论文
溯源树
样例
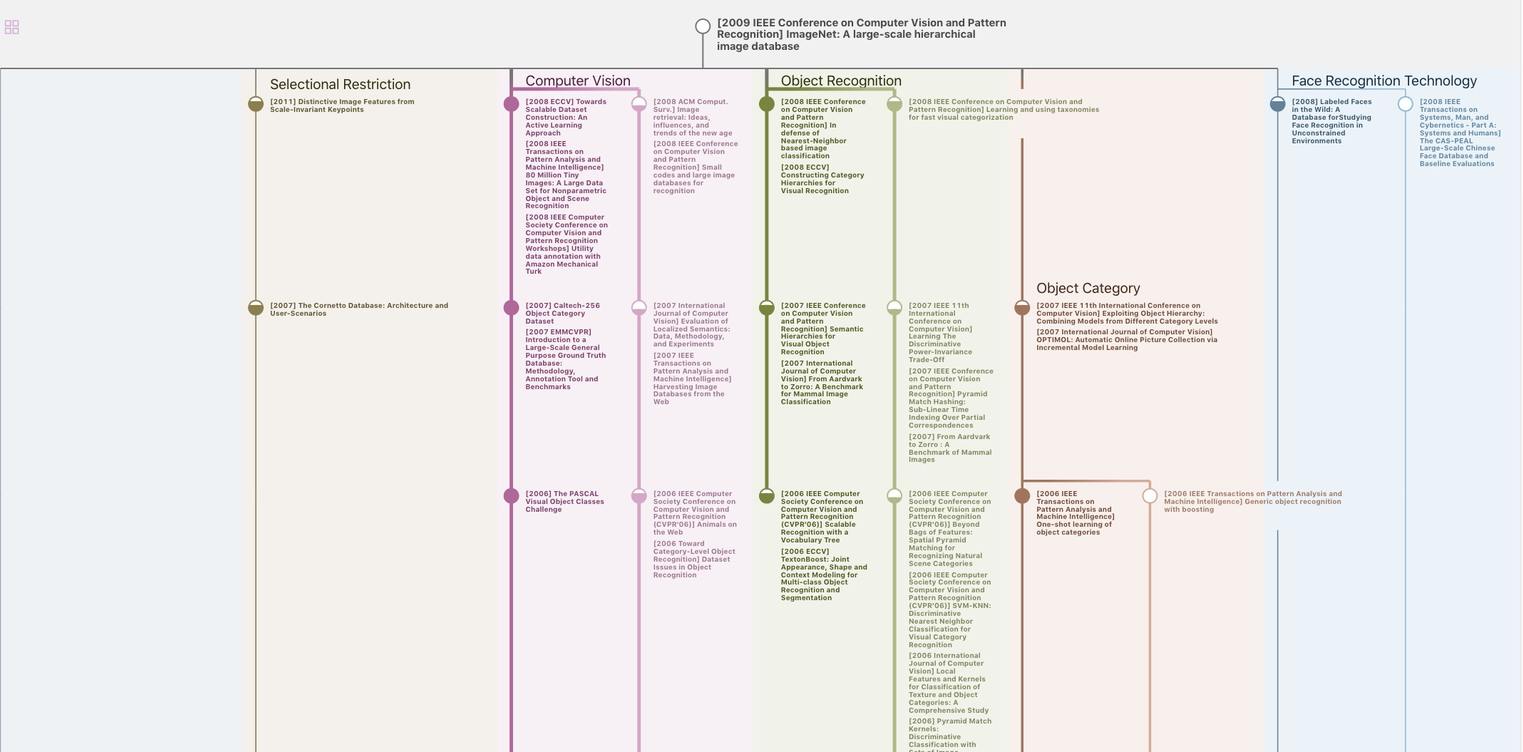
生成溯源树,研究论文发展脉络
Chat Paper
正在生成论文摘要