A Screening Mechanism Fast-Aggregation-Based Takagi-Sugeno-Kang Fuzzy Classification for Epileptic Electroencephalograms Signal
JOURNAL OF MEDICAL IMAGING AND HEALTH INFORMATICS(2019)
摘要
The classification of epileptic electroencephalograms signals (EEG) is of great importance for medical diagnosis. Takagi-Sugeno-Kang (TSK) fuzzy system is one of very important research hotspots in the field of artificial intelligence (AI). Because of its wide success in many fields, TSK fuzzy system has attracted more and more researchers' attention. How to further improve its classification performance is still a challenging task for machine learning. Based on the negative correlation learning theory, a multi-layer TSK fuzzy system with fast aggregation training (ML-TSK-FA) is proposed. The classifier ML-TSK-FA is also similar to deep learning hierarchy. The hidden layer in each base training block of ML-TSK-FA is represented by interpretable fuzzy rules. Based on the cascade structure theory, the source data is mapped into each independent base training block as the same input space. Unlike traditional constructions, this study proposes a screening mechanism for selecting TSK fuzzy classifiers with better classification performance in a training block, while discarding those with poor performance. The advantage of this method is that it greatly saves the training cost and improves the approximation performance of the training model. Experiments show that the proposed classifier ML-TSK-FA is very suitable for EEG signal classification. This also indirectly indicates that ML-TSK-FA is a promising classification system.
更多查看译文
关键词
Takagi-Sugeno-Kang (TSK),Screening Mechanism,Fast Aggregation,Fuzzy Classification,EEG
AI 理解论文
溯源树
样例
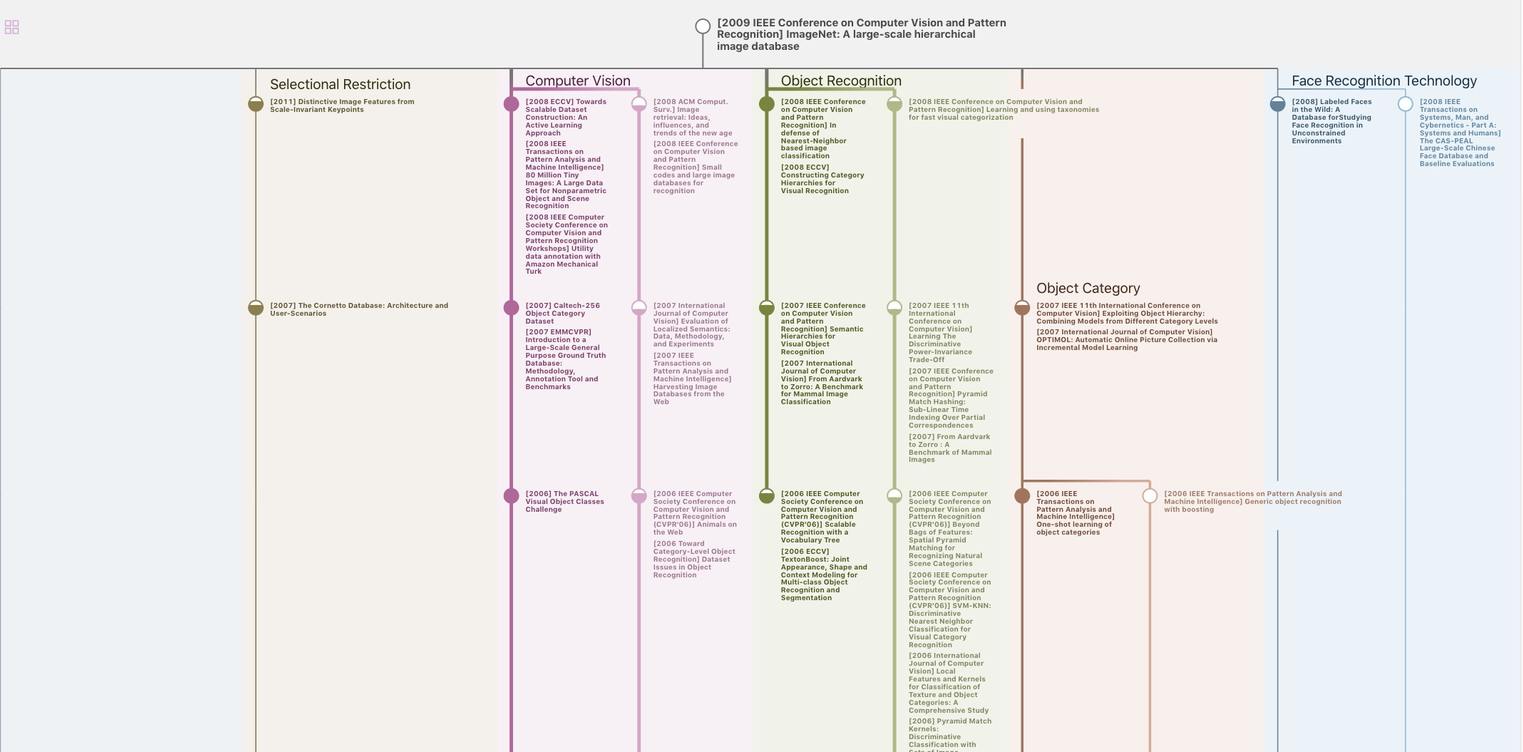
生成溯源树,研究论文发展脉络
Chat Paper
正在生成论文摘要