Abstract 1643: Discovery Pipeline of Cancer Transcriptomic Biomarkers Using Machine Learning
Bioinformatics, Convergence Science, and Systems Biology(2019)
摘要
Introduction: Cancer affects millions of people and causes nearly 1 in 6 deaths worldwide. Precision oncology holds potential in guiding cancer treatment on a molecular basis and elucidating targetable cancer pathways. This study aims to create a discovery pipeline that uses cancer patients’ transcriptomes and machine learning algorithms to predict cancer patient survival. Methods: Transcriptomic and phenotypic data from cancer patients were downloaded from The Cancer Genome Atlas (TCGA). Initial genes were selected using univariate cox proportional hazard models to predict overall survival across all cancer types. Co-expressed selected genes were clustered, creating gene sets per cancer subtype. Then, an amalgam of LASSO and elastic-net regularized generalized linear models (GLMNET), gene bootstrapping, random forest without/with shadow features (Boruta), and recursive feature elimination were used. Monte Carlo iterations were performed for GLMNET to assess model stability and over-/underfitting. Results: The highest hazard ratios (HRs) and lowest p-values resulted from the univariate cox proportional hazards model (e.g. for the RASGEF1A gene in a uterine serous carcinoma model, HR=2.8e8, p=0.005) and subsequent GLMNET models (e.g. 392 genes for uterine endometrioid carcinoma, HR=2.89e8, p=0.00873). Boruta before GLMNET yielded lower HRs and higher p-values (e.g. 11 genes for uterine serous carcinoma, HR=2, p=0.0517). Bootstrapping genes before GLMNET generally yielded much lower HRs and higher p-values. Conclusions: We were able to model survival risk in cancer patients with a variety of methods. The best method so far is univariate gene selection and GLMNET, although random forest is promising. Next steps include exploring random forest models further, investigating the biology of prognostic models, using disease-specific survival, analyzing possible confounders, using Breslow-Wilcoxon or accelerated failure time models, replacing histological with molecular-signature-based cancer subtypes, and exploring alternative methods for prognosis prediction. Supported by the Medical Scholars Program, Medical College of Georgia, Augusta, GA. Citation Format: Eileen Kim. Discovery pipeline of cancer transcriptomic biomarkers using machine learning [abstract]. In: Proceedings of the American Association for Cancer Research Annual Meeting 2019; 2019 Mar 29-Apr 3; Atlanta, GA. Philadelphia (PA): AACR; Cancer Res 2019;79(13 Suppl):Abstract nr 1643.
更多查看译文
关键词
Cancer Imaging,Cancer,Tumor Heterogeneity,Predictive Modeling,Transcriptional Landscape
AI 理解论文
溯源树
样例
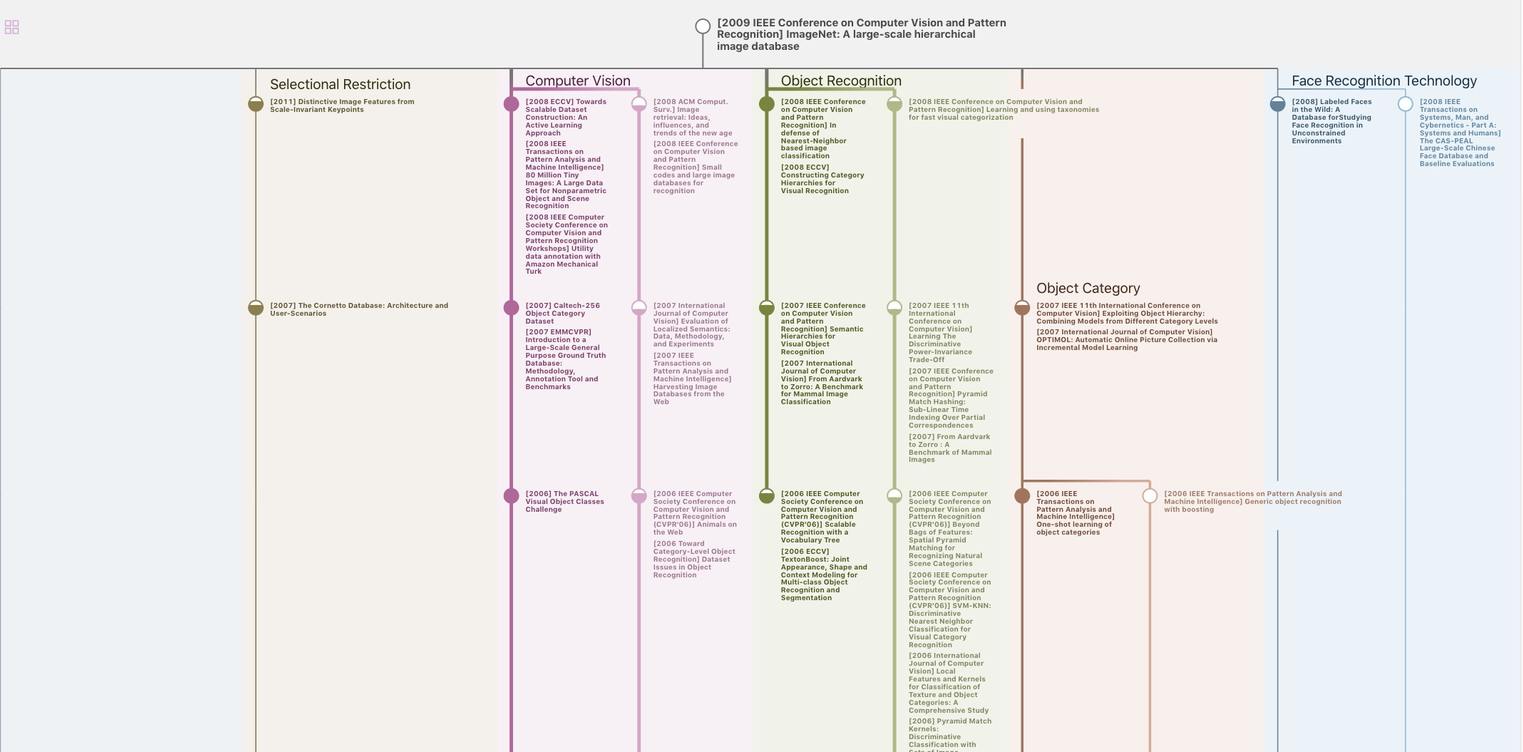
生成溯源树,研究论文发展脉络
Chat Paper
正在生成论文摘要