Applying a machine learning interatomic potential to unravel the effects of local lattice distortion on the elastic properties of multi-principal element alloys
JOURNAL OF ALLOYS AND COMPOUNDS(2019)
摘要
The concept of local lattice distortion (LLD) is of fundamental importance in the understanding of properties of high-entropy alloys and, more generally, of multi-principal element alloys (MPEAs). Despite previous experimental and computational efforts, the unambiguous evaluation of the static (due to atomic size difference) and dynamic (due to thermal fluctuation) LLD is still elusive. Here, as a first step, we develop a machine learning interatomic potential based on an efficient "learning-on-the-fly" scheme for CoFeNi, a prototypical ternary MPEA. Using this potential, we perform molecular dynamics simulations to calculate the elastic moduli of single- and polycrystalline CoFeNi. The results are in excellent agreement with theoretical and experimental data. As a second step, we design a simulation framework allowing the determination of the effects of static and dynamic LLD, thermal expansion, and chemical short-range order on the elastic properties of our prototypical MPEA. The results indicate that not only the average value of LLD, but also its probability distribution affect the elastic properties of MPEAs. In addition, we show that a variety of commonly used LLD indicators, e.g., atomic strain, pair distribution function, and bond-length distribution, correlate with each other. Our results not only shed light on the of LLD in MPEAs, but also demonstrate the capabilities of our machine learning potential as a powerful tool for the development and characterization of novel alloys with designed properties. (C) 2019 Published by Elsevier B.V.
更多查看译文
关键词
Multi-principal element alloys,High-entropy alloys,Elastic properties,Atomistic simulations,Machine learning
AI 理解论文
溯源树
样例
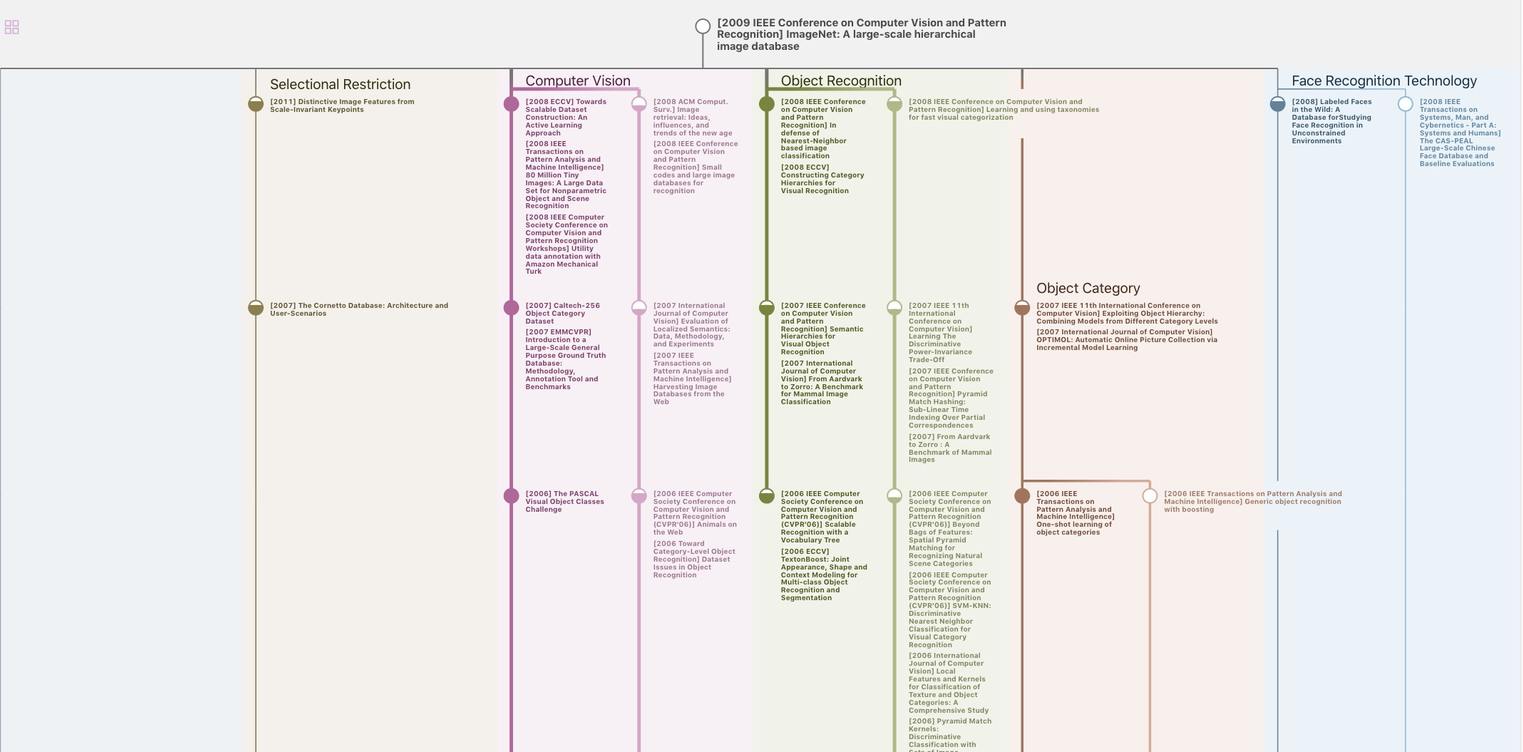
生成溯源树,研究论文发展脉络
Chat Paper
正在生成论文摘要