Learning fashion compatibility across categories with deep multimodal neural networks
Neurocomputing(2020)
摘要
Fashion compatibility is a subjective sense of human for relationships between fashion items, which is essential for fashion recommendation. Recently, it increasingly attracts more and more attentions and has become a very hot research topic. Learning fashion compatibility is a challenging task, since it needs to consider plenty of factors about fashion items, such as color, texture, style and functionality. Unlike low-level visual compatibility (e.g., color, texture), high-level semantic compatibility (e.g., style, functionality) cannot be handled purely based on fashion images. In this paper, we propose a novel multimodal framework to learn fashion compatibility, which simultaneously integrates both semantic and visual embeddings into a unified deep learning model. For semantic embeddings, a multilayered Long Short-Term Memory (LSTM) is employed for discriminative semantic representation learning, while a deep Convolutional Neural Network (CNN) is used for visual embeddings. A fusion module is then constructed to combine semantic and visual information of fashion items, which equivalently transforms semantic and visual spaces into a latent feature space. Furthermore, a new triplet ranking loss with compatible weights is introduced to measure fine-grained relationships between fashion items, which is more consistent with human feelings on fashion compatibility in reality. Extensive experiments conducted on Amazon fashion dataset demonstrate the effectiveness of the proposed method for learning fashion compatibility, which outperforms the state-of-the-art approaches.
更多查看译文
关键词
Fashion compatibility,Deep learning,Neural networks,Multimodal
AI 理解论文
溯源树
样例
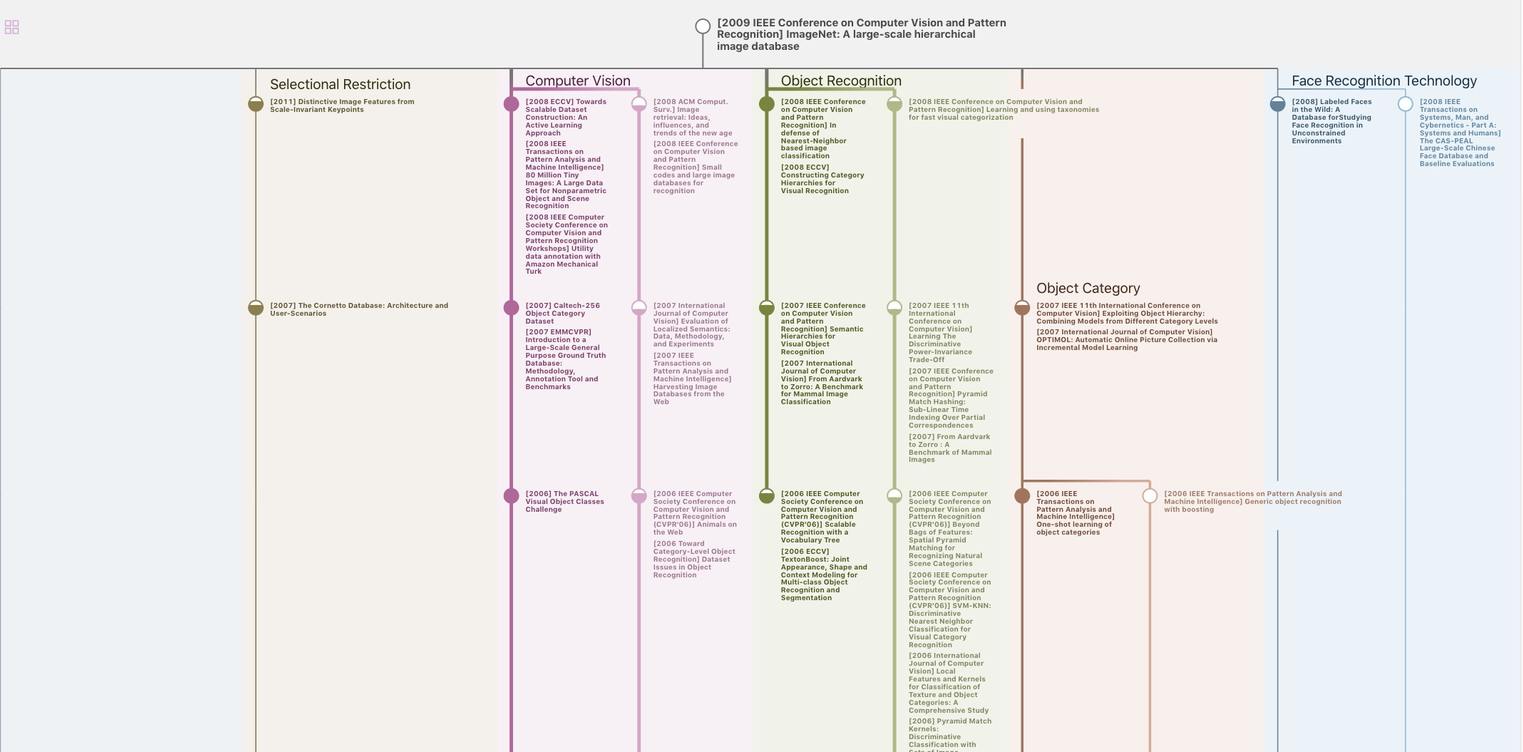
生成溯源树,研究论文发展脉络
Chat Paper
正在生成论文摘要