Learning Nonlinear State-Space Models Using Smooth Particle-Filter-Based Likelihood Approximations
IFAC PAPERSONLINE(2018)
摘要
When classical particle filtering algorithms are used for maximum likelihood parameter estimation in nonlinear state-space models, a key challenge is that estimates of the likelihood function and its derivatives are inherently noisy. The key idea in this paper is to run a particle filter based on a current parameter estimate, but then use the output from this particle filter to re-evaluate the likelihood function approximation also for other parameter values. This results in a (local) deterministic approximation of the likelihood and any standard optimization routine can be applied to find the maximum of this approximation. By iterating this procedure we eventually arrive at a final parameter estimate. (C) 2018, IFAC (International Federation of Automatic Control) Hosting by Elsevier Ltd. All rights reserved.
更多查看译文
关键词
System identification, parameter estimation, nonlinear state-space models, particle filter, maximum likelihood, optimization
AI 理解论文
溯源树
样例
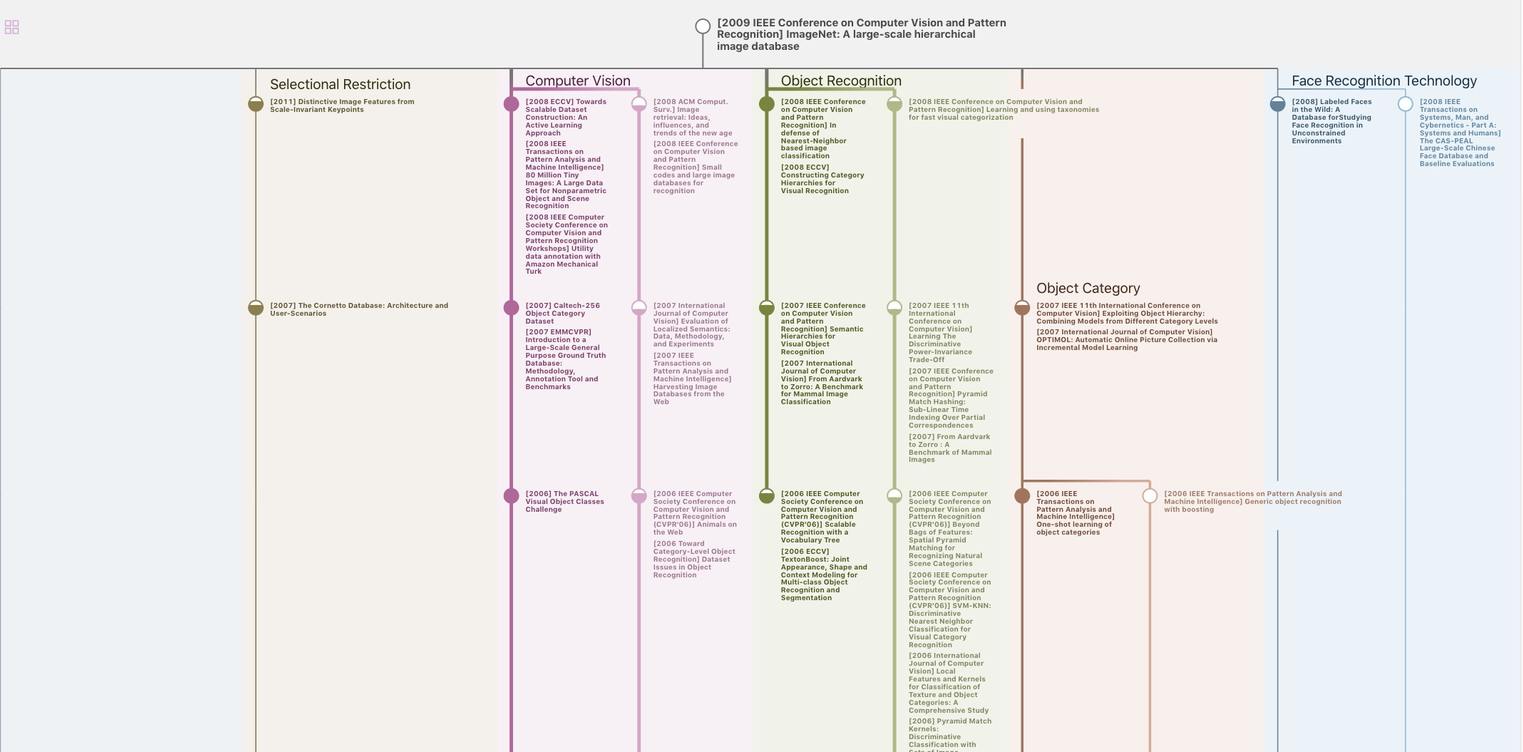
生成溯源树,研究论文发展脉络
Chat Paper
正在生成论文摘要