Achieving shrinkage in a time-varying parameter model framework
Journal of Econometrics(2019)
摘要
Shrinkage for time-varying parameter (TVP) models is investigated within a Bayesian framework, with the aim to automatically reduce time-varying parameters to static ones, if the model is overfitting. This is achieved through placing the double gamma shrinkage prior on the process variances. An efficient Markov chain Monte Carlo scheme is developed, exploiting boosting based on the ancillarity-sufficiency interweaving strategy. The method is applicable both to TVP models for univariate as well as multivariate time series. Applications include a TVP generalized Phillips curve for EU area inflation modeling and a multivariate TVP Cholesky stochastic volatility model for joint modeling of the returns from the DAX-30 index.
更多查看译文
关键词
Bayesian inference,Bayesian Lasso,Double gamma prior,Hierarchical priors,Kalman filter,Log predictive density scores,Normal–gamma prior,Sparsity,State space model
AI 理解论文
溯源树
样例
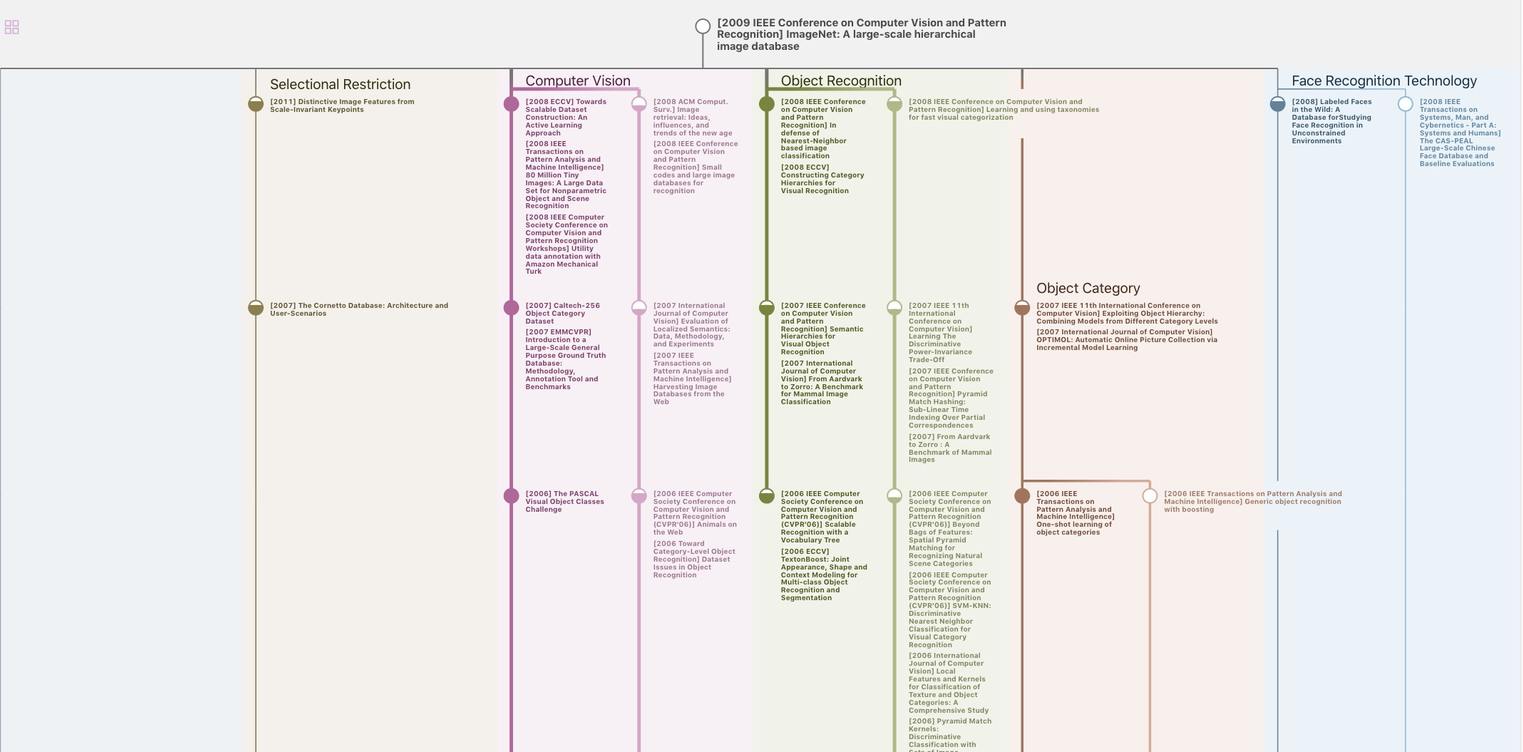
生成溯源树,研究论文发展脉络
Chat Paper
正在生成论文摘要