On the Convergence of the SINDy Algorithm
MULTISCALE MODELING & SIMULATION(2019)
摘要
One way to understand time-series data is to identify the underlying dynamical system which generates it. This task can be done by selecting an appropriate model and a set of parameters which best fits the dynamics while providing the simplest representation (i.e., the smallest amount of terms). One such approach is the sparse identification of nonlinear dynamics framework [6], which uses a sparsity-promoting algorithm that iterates between a partial least-squares fit and a thresholding (sparsity-promoting) step. In this work, we provide some theoretical results on the behavior and convergence of the algorithm proposed in [S. L. Brunton, J. L. Proctor, and J. N. Kutz, Proc. Nat. Acad. Sci. USA, 113 (2016), pp. 3932-3937]. In particular, we prove that the algorithm approximates local minimizers of an unconstrained l(0)-penalized least-squares problem. From this, we provide sufficient conditions for general convergence, rate of convergence, conditions for one-step recovery, and a recovery result with respect to the condition number and noise. Examples illustrate that the rates of convergence are sharp. In addition, our results extend to other algorithms related to the algorithm in [S. L. Brunton, J. L. Proctor, and J. N. Kutz, Proc. Nat. Acad. Sci. USA, 113 (2016), pp. 3932-3937], and provide theoretical verification of several observed phenomena.
更多查看译文
关键词
sparse approximation,convergence analysis,model selection
AI 理解论文
溯源树
样例
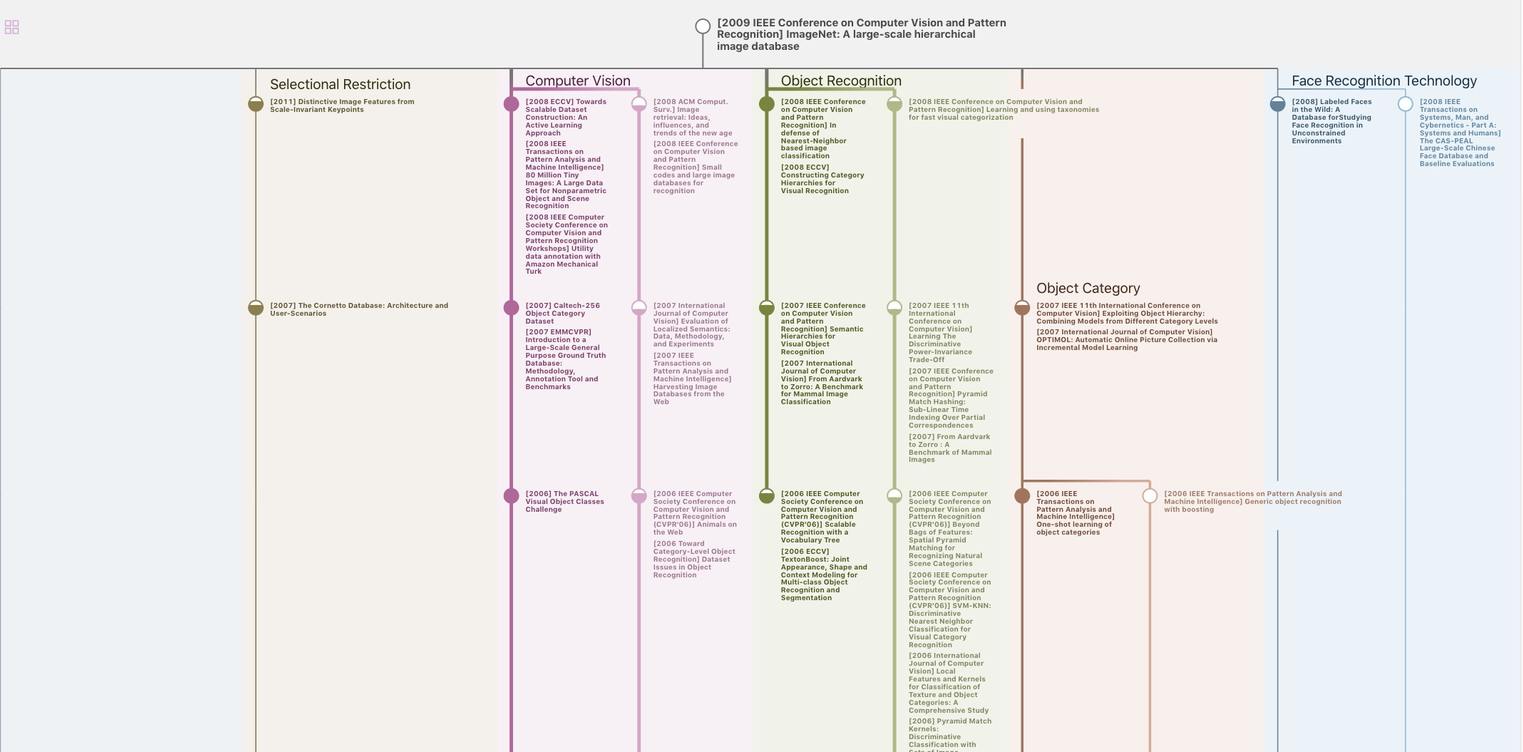
生成溯源树,研究论文发展脉络
Chat Paper
正在生成论文摘要