Accelerate Stochastic Subgradient Method by Leveraging Local Growth Condition
ANALYSIS AND APPLICATIONS(2019)
摘要
In this paper, a new theory is developed for first-order stochastic convex optimization, showing that the global convergence rate is sufficiently quantified by a local growth rate of the objective function in a neighborhood of the optimal solutions. In particular, if the objective function F(w) in the c-sublevel set grows as fast as parallel to w - w(*)parallel to(1/theta)(2), where w(*) represents the closest optimal solution to w and theta is an element of (0, 1] quantifies the local growth rate, the iteration complexity of first-order stochastic optimization for achieving an epsilon-optimal solution can be (O) over tilde (1/epsilon(2(1-theta))), which is optimal at most up to a logarithmic factor. To achieve the faster global convergence, we develop two different accelerated stochastic subgradient methods by iteratively solving the original problem approximately in a local region around a historical solution with the size of the local region gradually decreasing as the solution approaches the optimal set. Besides the theoretical improvements, this work also includes new contributions toward making the proposed algorithms practical: (i) we present practical variants of accelerated stochastic subgradient methods that can run without the knowledge of multiplicative growth constant and even the growth rate theta; (ii) we consider a broad family of problems in machine learning to demonstrate that the proposed algorithms enjoy faster convergence than traditional stochastic subgradient method. We also characterize the complexity of the proposed algorithms for ensuring the gradient is small without the smoothness assumption.
更多查看译文
关键词
Convex optimization,stochastic subgradient,local growth condition
AI 理解论文
溯源树
样例
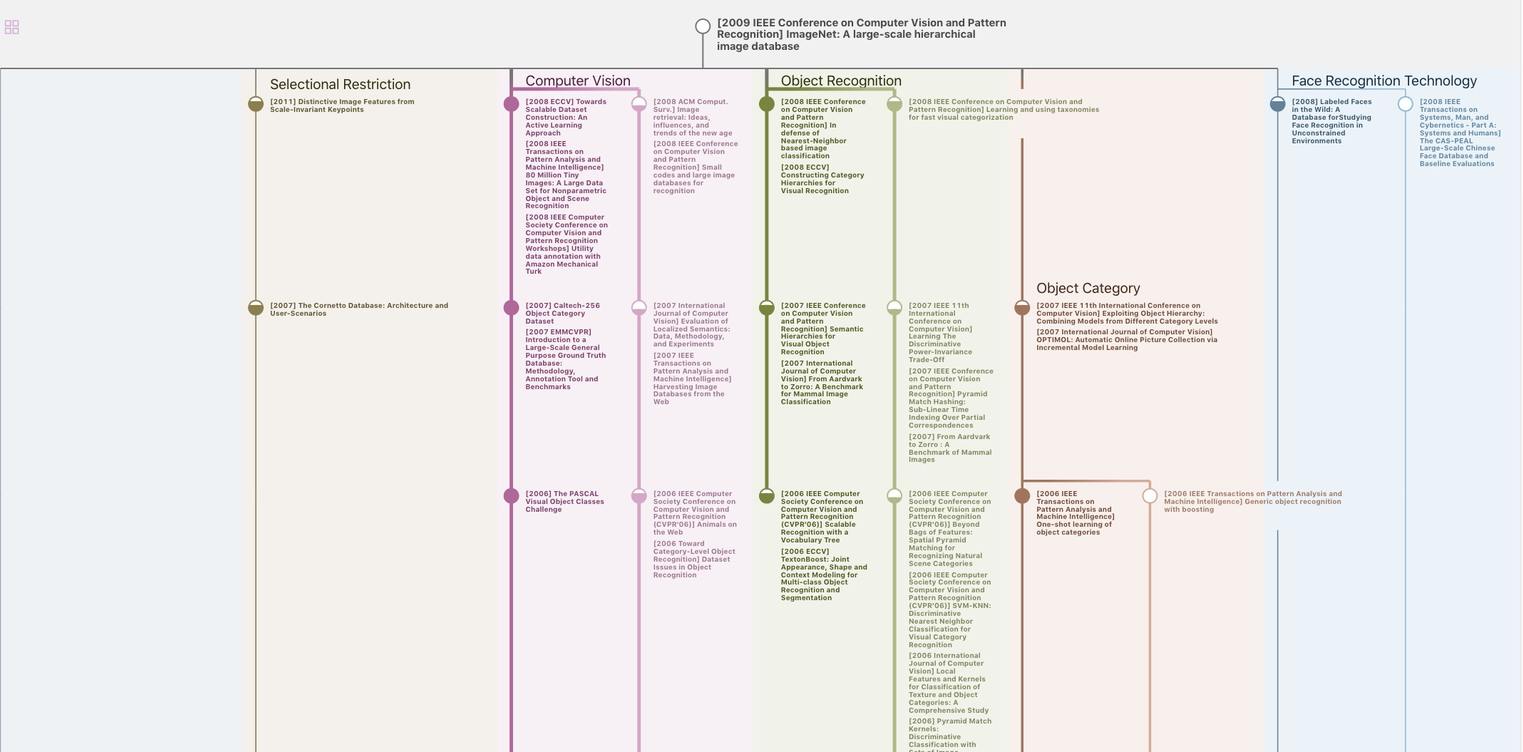
生成溯源树,研究论文发展脉络
Chat Paper
正在生成论文摘要