A Computational Framework for Multivariate Convex Regression and Its Variants
Journal of the American Statistical Association(2018)
摘要
We study the nonparametric least squares estimator (LSE) of a multivariate convex regression function. The LSE, given as the solution to a quadratic program with O(n(2)) linear constraints (n being the sample size), is difficult to compute for large problems. Exploiting problem specific structure, we propose a scalable algorithmic framework based on the augmented Lagrangian method to compute the LSE. We develop a novel approach to obtain smooth convex approximations to the fitted (piecewise affine) convex LSE and provide formal bounds on the quality of approximation. When the number of samples is not too large compared to the dimension of the predictor, we propose a regularization schemeLipschitz convex regressionwhere we constrain the norm of the subgradients, and study the rates of convergence of the obtained LSE. Our algorithmic framework is simple and flexible and can be easily adapted to handle variants: estimation of a nondecreasing/nonincreasing convex/concave (with or without a Lipschitz bound) function. We perform numerical studies illustrating the scalability of the proposed algorithmon some instances our proposal leads to more than a 10,000-fold improvement in runtime when compared to off-the-shelf interior point solvers for problems with n = 500.
更多查看译文
关键词
Augmented Lagrangian method,Lipschitz convex regression,Nonparametric least squares estimator,Scalable quadratic programming,Smooth convex regression
AI 理解论文
溯源树
样例
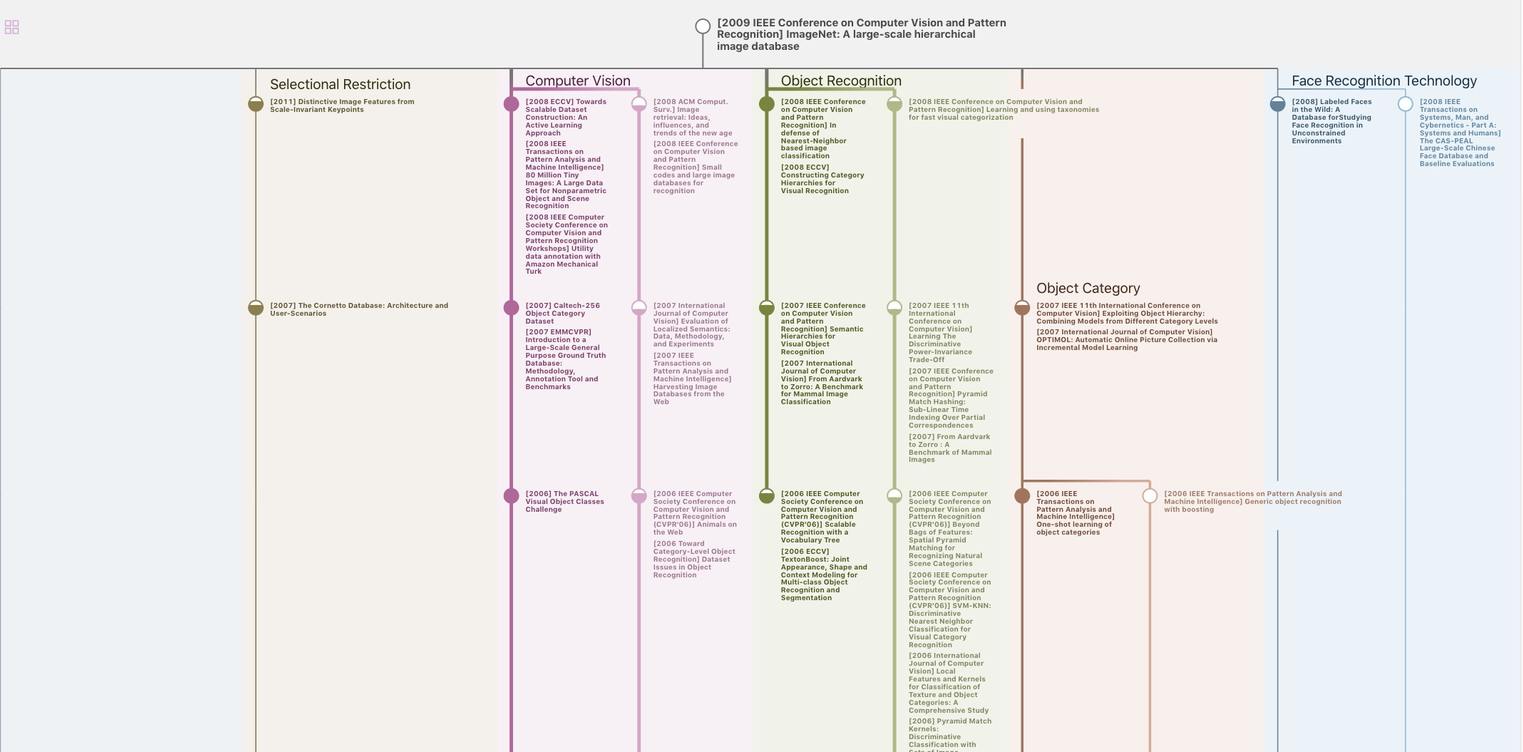
生成溯源树,研究论文发展脉络
Chat Paper
正在生成论文摘要