Siamese-Network-Based Learning To Rank For No-Reference 2d And 3d Image Quality Assessment
IEEE ACCESS(2019)
摘要
2D image quality assessment (IQA) and stereoscopic 3D IQA are considered as two different tasks in the literature. In this paper, we present an index for both no-reference 2D and 3D IQA. We propose to transform the IQA task into a task of quality comparison between images. By generating image pairs, the amount of training data reaches the square of the original amount of data, effectively solving the lacking of training samples. We also propose a learning to rank model using Siamese convolutional neural networks (LRSN) for quality comparison. The presented LRSN has two branches that have the same structure, share weights with each other, and take two image patches as inputs. The goal of LRSN is learning to rank the quality scores between the two input image patches. The relative quality score of a test image is obtained by first comparing its image patches with many image patches of other images and counts the number of times that its image patches are ranked superior to other patches. The experimental results on three 2D (LIVE, CSIQ, and TID2013) and three 3D (LIVE 3D Phase-I, LIVE 3D Phase-II, and NBU) IQA databases demonstrate that the proposed LRSN model works well for both 2D and 3D IQA and outperforms the state-of-the-art no-reference 2D and 3D IQA metrics.
更多查看译文
关键词
No-reference image quality assessment,stereoscopic image quality assessment,Siamese convolutional neural networks,learning to rank
AI 理解论文
溯源树
样例
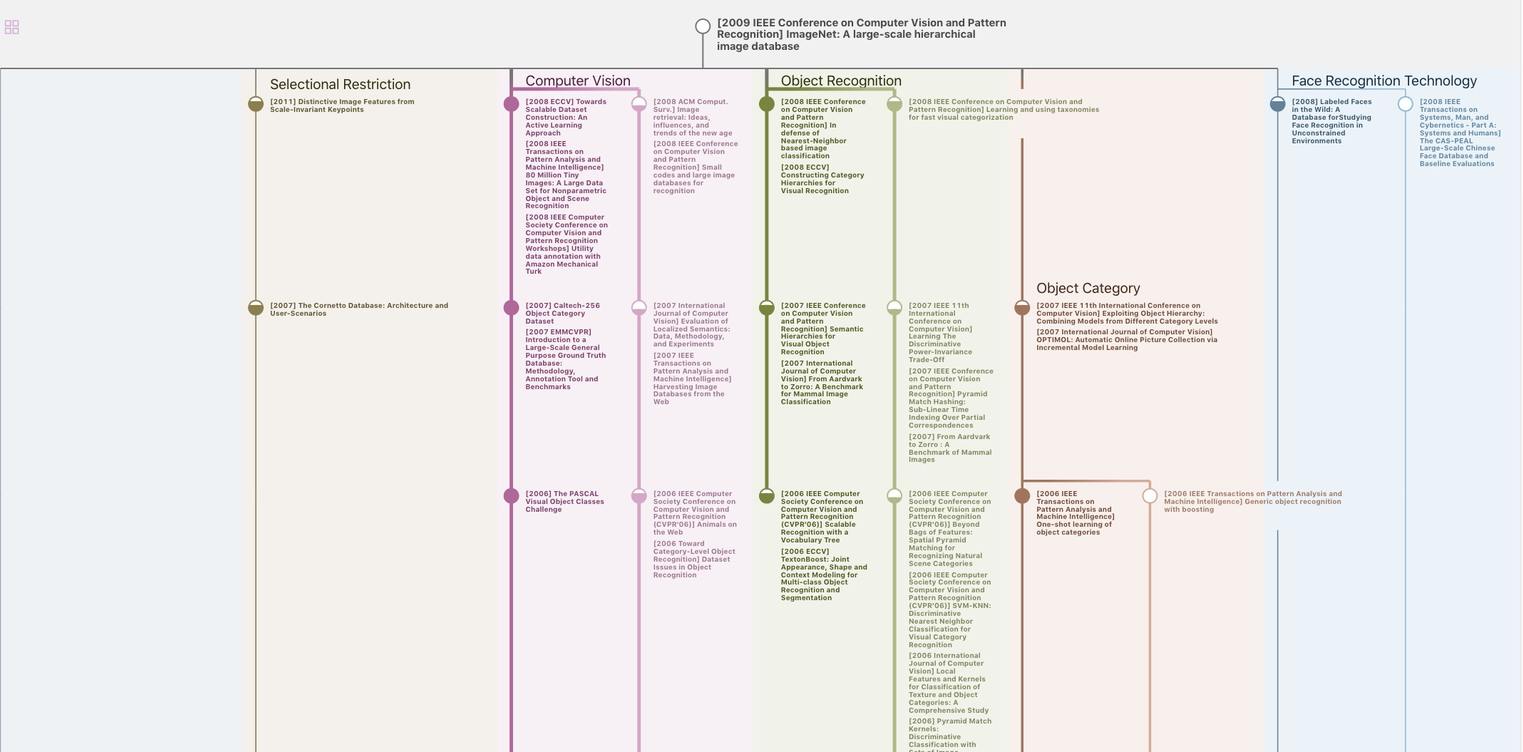
生成溯源树,研究论文发展脉络
Chat Paper
正在生成论文摘要