Volumetric Data Exploration with Machine Learning-Aided Visualization in Neutron Science
ADVANCES IN COMPUTER VISION, CVC, VOL 1(2020)
摘要
Recent advancements in neutron and X-ray sources, instrumentation and data collection modes have significantly increased the experimental data size (which could easily contain 108-1010 data points), so that conventional volumetric visualization approaches become inefficient for both still imaging and interactive OpenGL rendition in a 3D setting. We introduce a new approach based on the unsupervised machine learning algorithm, Density-Based Spatial Clustering of Applications with Noise (DBSCAN), to efficiently analyze and visualize large volumetric datasets. Here we present two examples of analyzing and visualizing datasets from the diffuse scattering experiment of a single crystal sample and the tomographic reconstruction of a neutron scanning of a turbine blade. We found that by using the intensity as the weighting factor in the clustering process, DBSCAN becomes very effective in de-noising and feature/boundary detection, and thus enables better visualization of the hierarchical internal structures of the neutron scattering data.
更多查看译文
关键词
Scientific visualization,Feature extraction,Unsupervised learning and clustering,Volumetric dataset
AI 理解论文
溯源树
样例
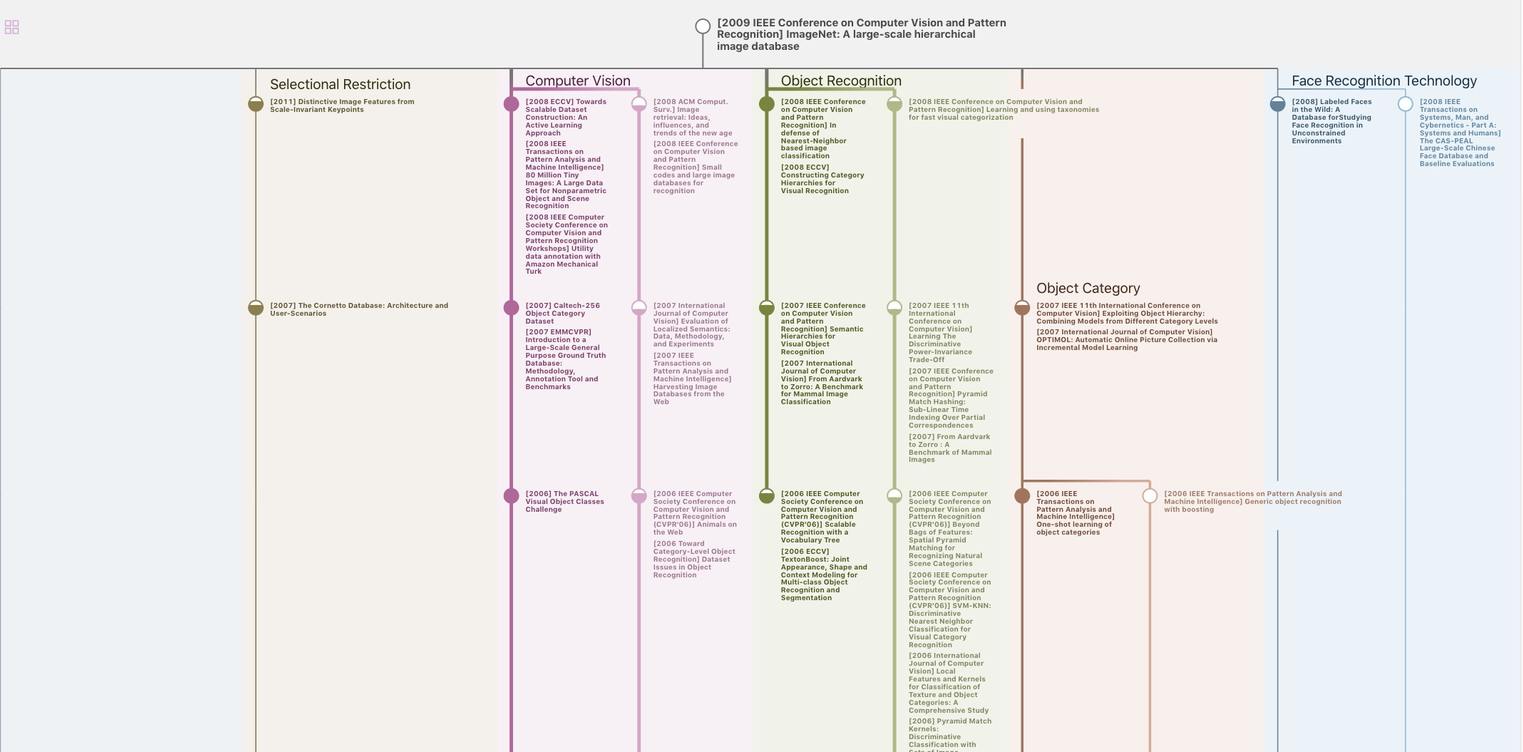
生成溯源树,研究论文发展脉络
Chat Paper
正在生成论文摘要