SmoothFool: An Efficient Framework for Computing Smooth Adversarial Perturbations
2020 IEEE WINTER CONFERENCE ON APPLICATIONS OF COMPUTER VISION (WACV)(2019)
摘要
Deep neural networks are susceptible to adversarial manipulations in the input domain. The extent of vulnerability has been explored intensively in cases of $\ell_p$-bounded and $\ell_p$-minimal adversarial perturbations. However, the vulnerability of DNNs to adversarial perturbations with specific statistical properties or frequency-domain characteristics has not been sufficiently explored. In this paper, we study the smoothness of perturbations and propose SmoothFool, a general and computationally efficient framework for computing smooth adversarial perturbations. Through extensive experiments, we validate the efficacy of the proposed method for both the white-box and black-box attack scenarios. In particular, we demonstrate that: (i) there exist extremely smooth adversarial perturbations for well-established and widely used network architectures, (ii) smoothness significantly enhances the robustness of perturbations against state-of-the-art defense mechanisms, (iii) smoothness improves the transferability of adversarial perturbations across both data points and network architectures, and (iv) class categories exhibit a variable range of susceptibility to smooth perturbations. Our results suggest that smooth APs can play a significant role in exploring the vulnerability extent of DNNs to adversarial examples.
更多查看译文
关键词
white-box attack scenarios,black-box attack scenarios,network architectures,adversarial examples,SmoothFool,deep neural networks,adversarial manipulations,adversarial perturbations,computer vision
AI 理解论文
溯源树
样例
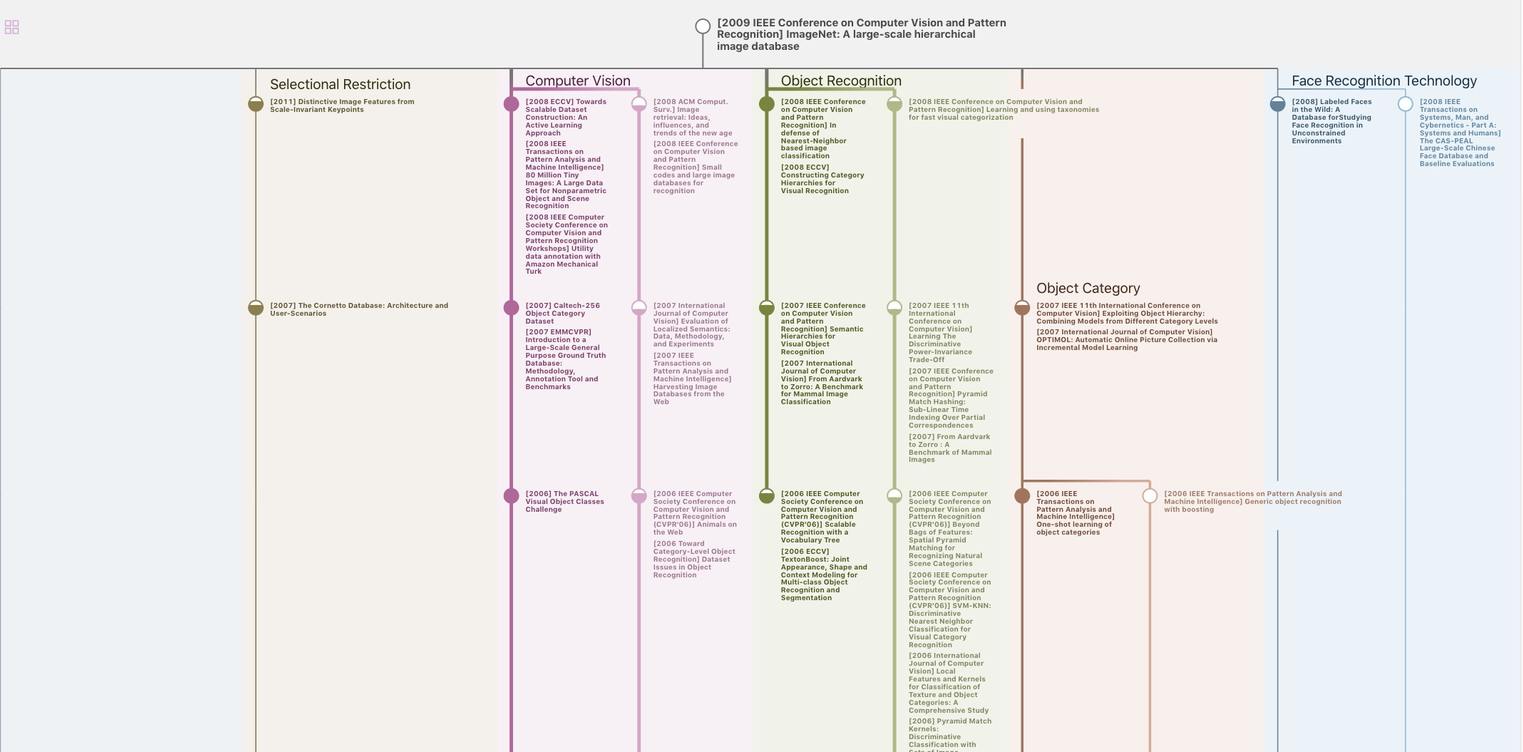
生成溯源树,研究论文发展脉络
Chat Paper
正在生成论文摘要