Forecast Aggregation via Peer Prediction.
AAAI Conference on Human Computation & Crowdsourcing (HCOMP)(2021)
摘要
Crowdsourcing is a popular paradigm for soliciting forecasts on future events. As people may have different forecasts, how to aggregate solicited forecasts into a single accurate prediction remains to be an important challenge, especially when no historical accuracy information is available for identifying experts. In this paper, we borrow ideas from the peer prediction literature and assess the prediction accuracy of participants using solely the collected forecasts. This approach leverages the correlations among peer reports to cross-validate each participant's forecasts and allows us to assign a "peer assessment score (PAS)" for each agent as a proxy for the agent's prediction accuracy. We identify several empirically effective methods to generate PAS and propose an aggregation framework that uses PAS to identify experts and to boost existing aggregators' prediction accuracy. We evaluate our methods over 14 real-world datasets and show that i) PAS generated from peer prediction methods can approximately reflect the prediction accuracy of agents, and ii) our aggregation framework demonstrates consistent and significant improvement in the prediction accuracy over existing aggregators for both binary and multi-choice questions under three popular accuracy measures: Brier score (mean square error), log score (cross-entropy loss) and AUC-ROC.
更多查看译文
关键词
forecast aggregation,peer prediction
AI 理解论文
溯源树
样例
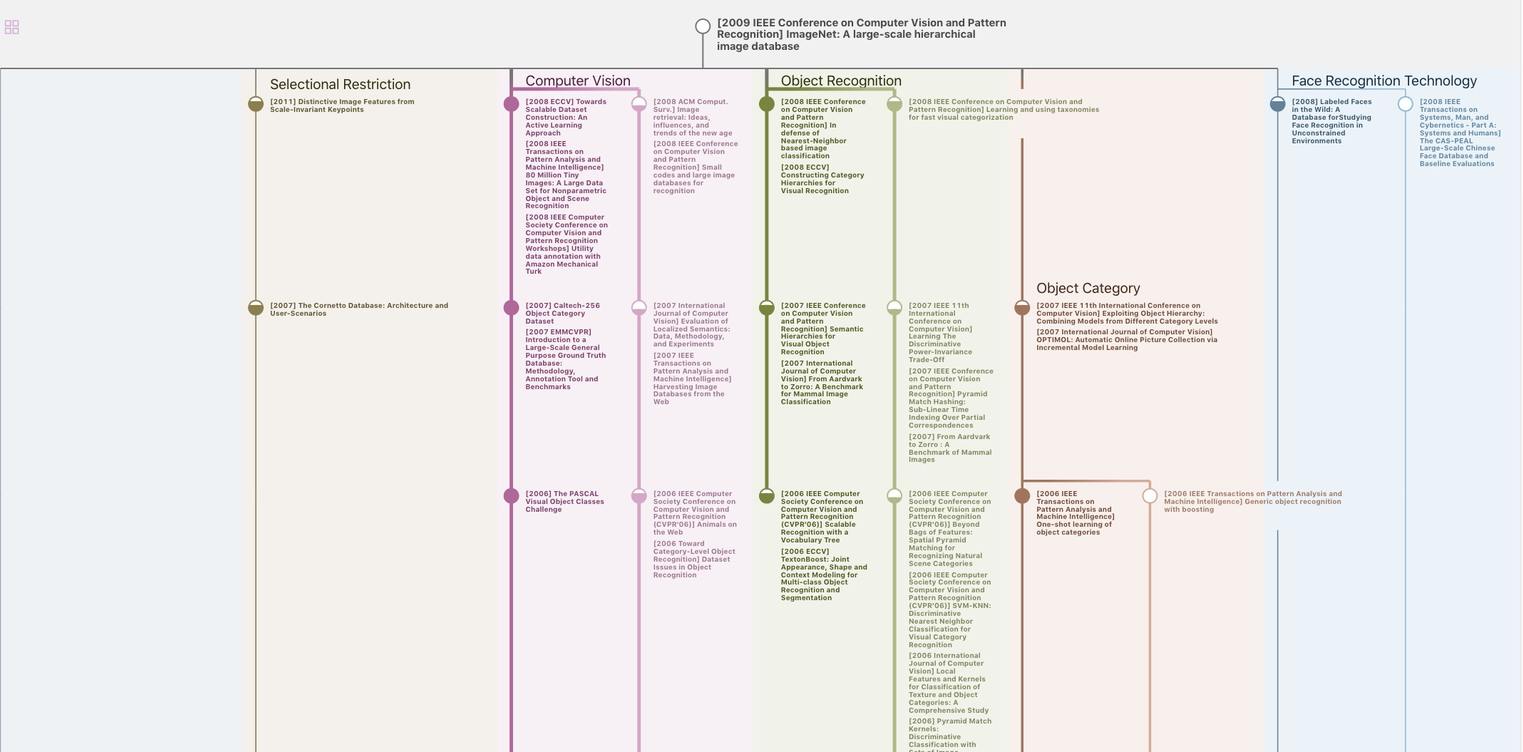
生成溯源树,研究论文发展脉络
Chat Paper
正在生成论文摘要