Continual Learning Using Bayesian Neural Networks
IEEE Transactions on Neural Networks and Learning Systems(2021)
摘要
Continual learning models allow them to learn and adapt to new changes and tasks over time. However, in continual and sequential learning scenarios, in which the models are trained using different data with various distributions, neural networks (NNs) tend to forget the previously learned knowledge. This phenomenon is often referred to as catastrophic forgetting. The catastrophic forgetting is an inevitable problem in continual learning models for dynamic environments. To address this issue, we propose a method, called continual Bayesian learning networks (CBLNs), which enables the networks to allocate additional resources to adapt to new tasks without forgetting the previously learned tasks. Using a Bayesian NN, CBLN maintains a mixture of Gaussian posterior distributions that are associated with different tasks. The proposed method tries to optimize the number of resources that are needed to learn each task and avoids an exponential increase in the number of resources that are involved in learning multiple tasks. The proposed method does not need to access the past training data and can choose suitable weights to classify the data points during the test time automatically based on an uncertainty criterion. We have evaluated the method on the MNIST and UCR time-series data sets. The evaluation results show that the method can address the catastrophic forgetting problem at a promising rate compared to the state-of-the-art models.
更多查看译文
关键词
Bayesian neural networks (BNNs),catastrophic forgetting,continual learning,incremental learning,uncertainty
AI 理解论文
溯源树
样例
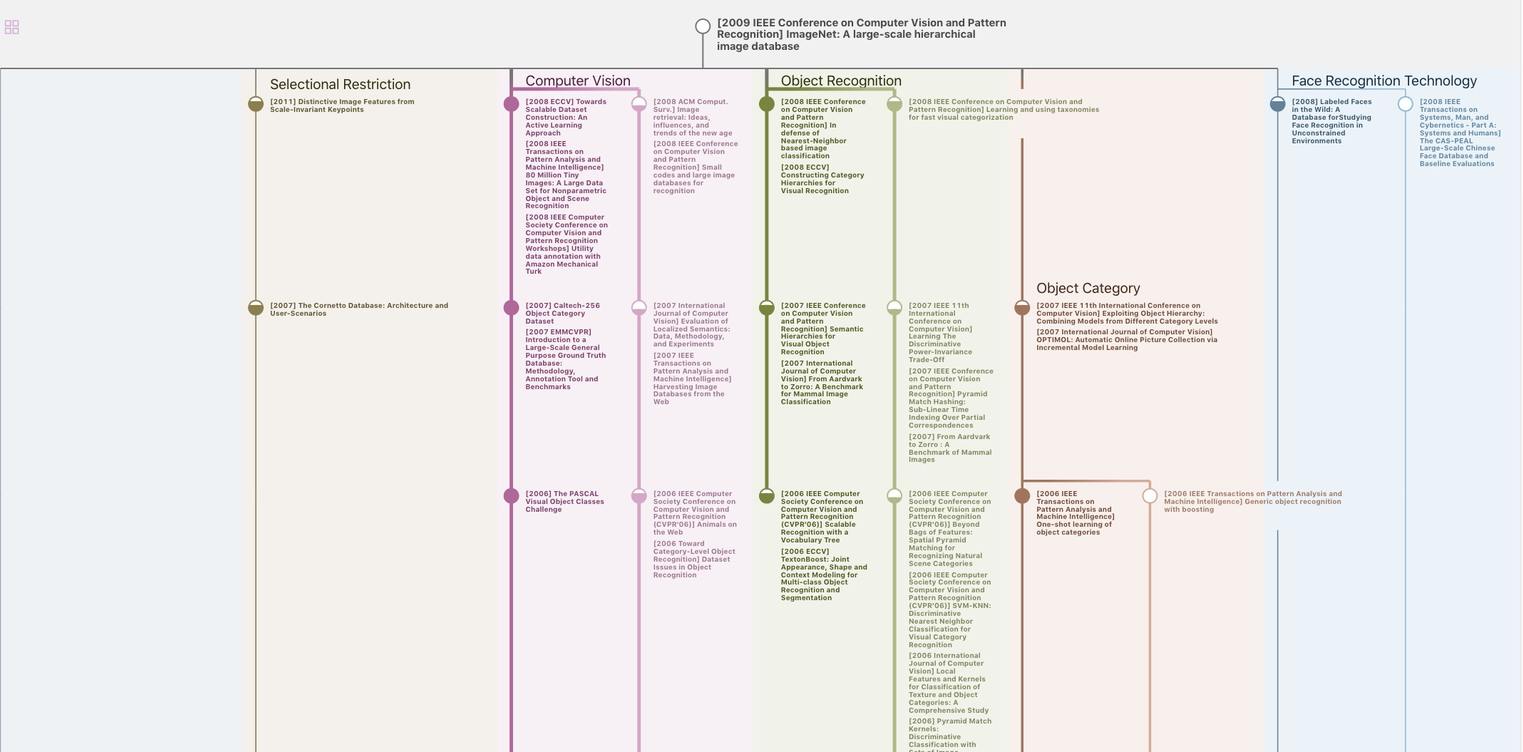
生成溯源树,研究论文发展脉络
Chat Paper
正在生成论文摘要