Tuning Parameters Via A New Rapid, Accurate And Parameter-Less Method Using Meta-Learning
INTERNATIONAL JOURNAL OF DATA MINING MODELLING AND MANAGEMENT(2019)
摘要
Dealing with a large parameter space in data mining tasks is extremely time-consuming, and the tuning method itself needs to be tuned since methods themselves have at least one parameter. Here, a new rapid and parameter-less method is presented to tune algorithms on diverse datasets to achieve high quality results in a short consumed time. The method presented here uses a pre-knowledge by using meta-features to guess closer point to optimal point in parameter space of target algorithms (here, support vector machine algorithm is used). For preparing the pre-knowledge, 282 meta-features are introduced and then genetic algorithm is applied to determine best meta-features for the target algorithm. Then the best meta-features are used to tune the target algorithm on unseen datasets. The results show in less than 0.19 minute in average, the method obtains approximately the same classification rates in comparison with others, but the consumed time is dramatically declined.
更多查看译文
关键词
parameter tuning, meta learning, meta feature, SVM tuning, genetic algorithm
AI 理解论文
溯源树
样例
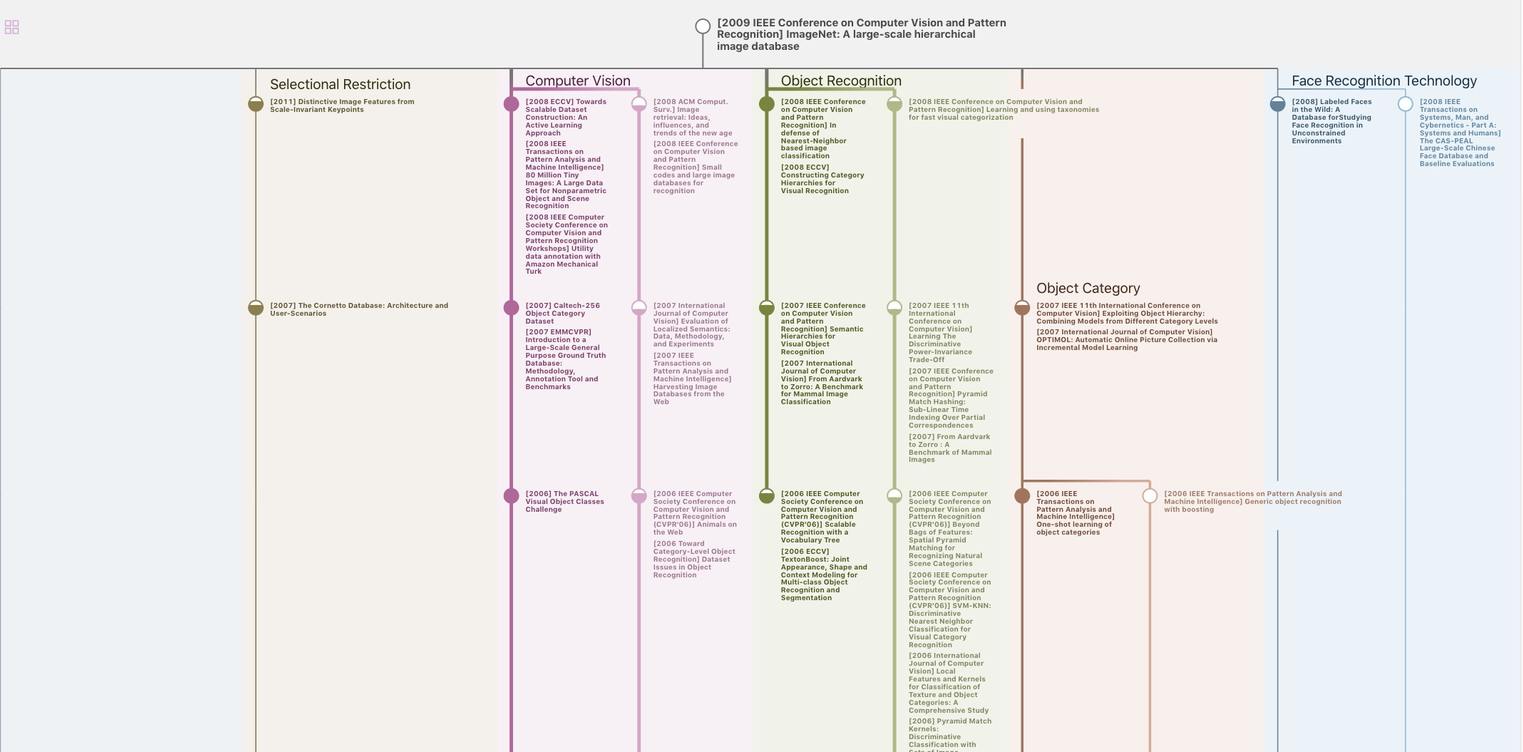
生成溯源树,研究论文发展脉络
Chat Paper
正在生成论文摘要