Semiparametric Bayesian Causal Inference
ANNALS OF STATISTICS(2020)
摘要
We develop a semiparametric Bayesian approach for estimating the mean response in a missing data model with binary outcomes and a nonparametrically modelled propensity score. Equivalently, we estimate the causal effect of a treatment, correcting nonparametrically for confounding. We show that standard Gaussian process priors satisfy a semiparametric Bernsteinvon Mises theorem under smoothness conditions. We further propose a novel propensity score-dependent prior that provides efficient inference under strictly weaker conditions. We also show that it is theoretically preferable to model the covariate distribution with a Dirichlet process or Bayesian bootstrap, rather than modelling its density.
更多查看译文
关键词
Bernstein-von Mises, Gaussian processes, propensity score-dependent priors, causal inference, Dirichlet process
AI 理解论文
溯源树
样例
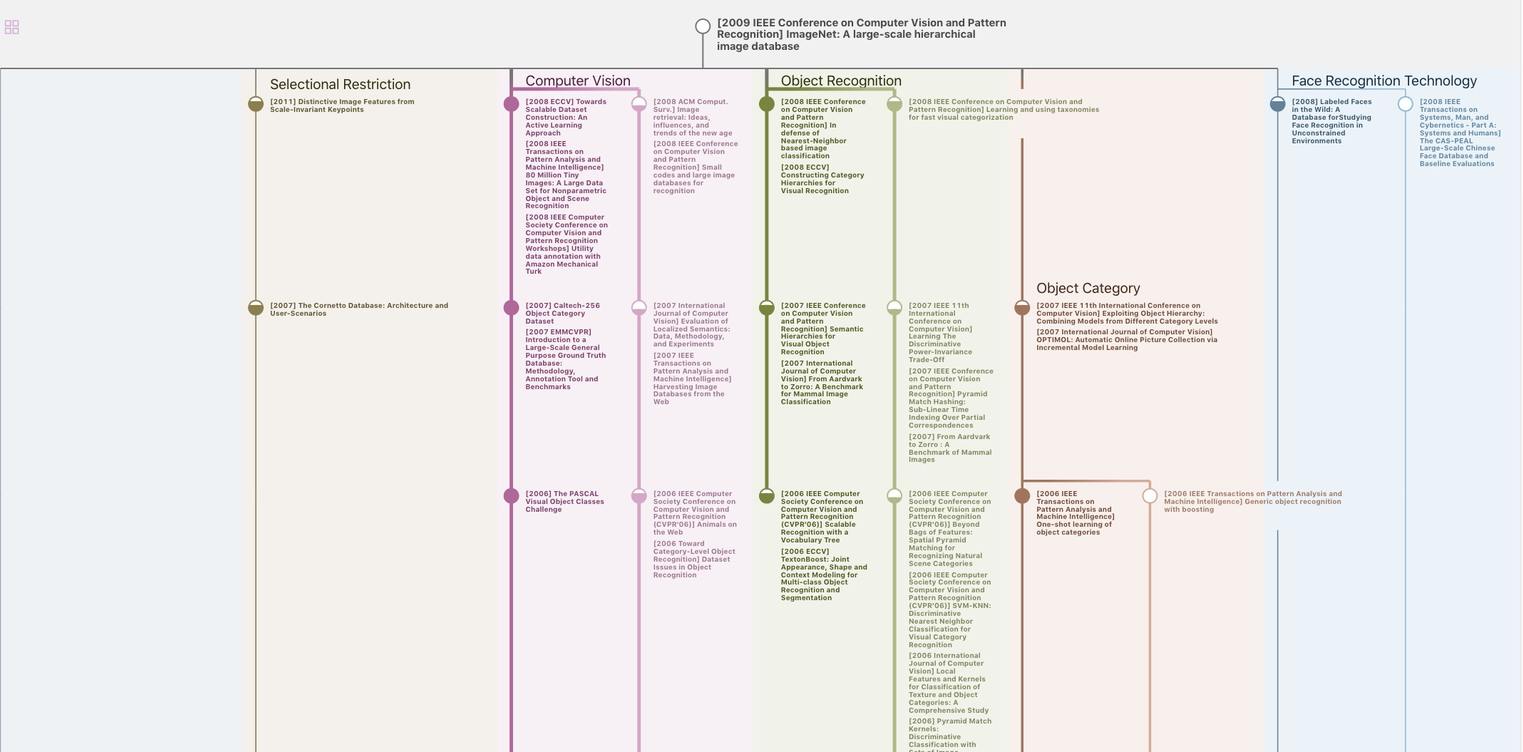
生成溯源树,研究论文发展脉络
Chat Paper
正在生成论文摘要