Learning Only from Relevant Keywords and Unlabeled Documents
2019 CONFERENCE ON EMPIRICAL METHODS IN NATURAL LANGUAGE PROCESSING AND THE 9TH INTERNATIONAL JOINT CONFERENCE ON NATURAL LANGUAGE PROCESSING (EMNLP-IJCNLP 2019): PROCEEDINGS OF THE CONFERENCE(2019)
摘要
We consider a document classification problem where document labels are absent but only relevant keywords of a target class and unlabeled documents are given. Although heuristic methods based on pseudo-labeling have been considered, theoretical understanding of this problem has still been limited. Moreover, previous methods cannot easily incorporate welldeveloped techniques in supervised text classification. In this paper, we propose a theoretically guaranteed learning framework that is simple to implement and has flexible choices of models, e.g., linear models or neural networks. We demonstrate how to optimize the area under the receiver operating characteristic curve (AUC) effectively and also discuss how to adjust it to optimize other well-known evaluation metrics such as the accuracy and F-1-measure. Finally, we show the effectiveness of our framework using benchmark datasets.
更多查看译文
关键词
relevant keywords,learning
AI 理解论文
溯源树
样例
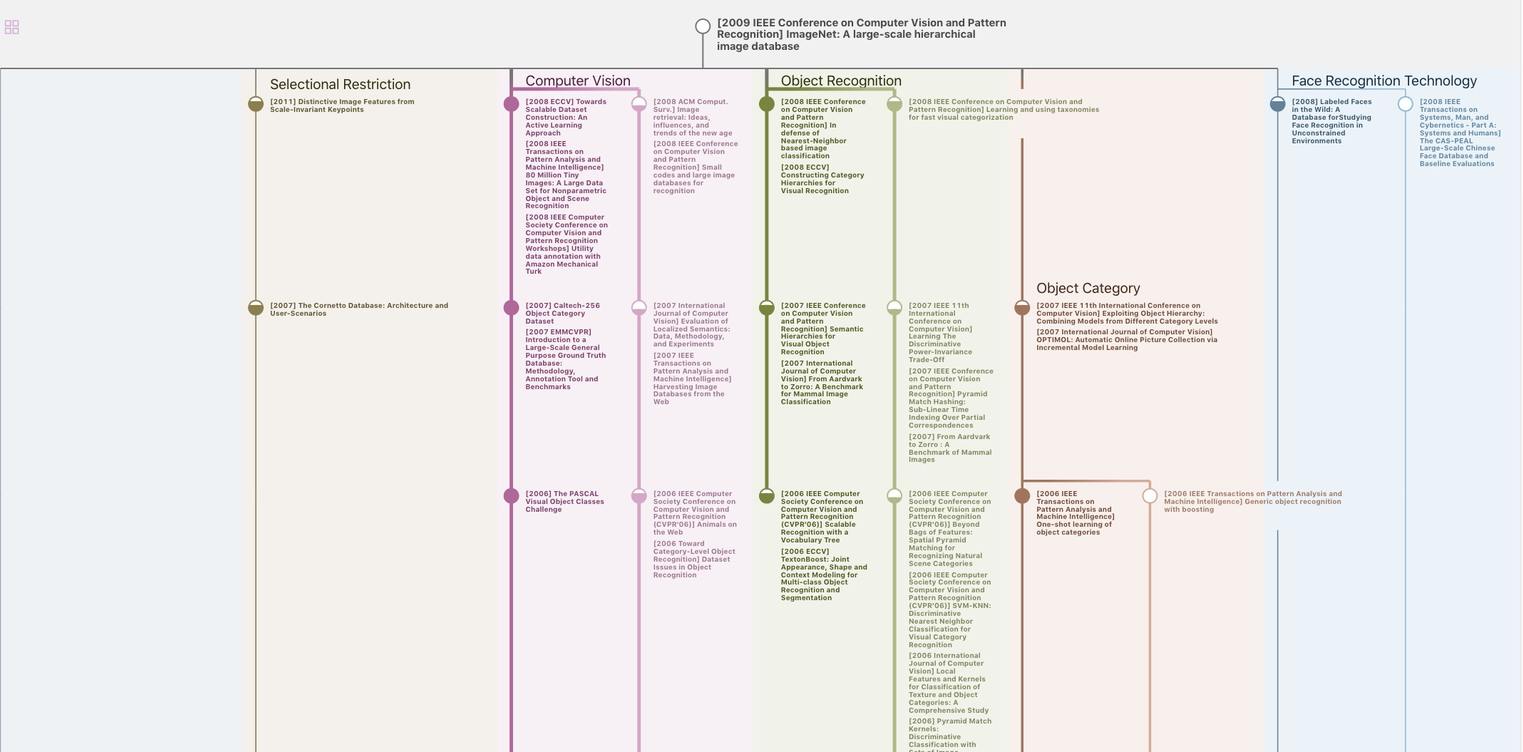
生成溯源树,研究论文发展脉络
Chat Paper
正在生成论文摘要