A Highly Efficient Remote Access Trojan Detection Method
Periodicals(2019)
摘要
AbstractNowadays, machine learning is popular in remote access Trojan (RAT) detection which can create patterns for decision-making. However, most research focus on improving the detection rate and reducing the false negative rate, therefore they ignore the result of abnormal samples. In addition, most classifiers select several proprietary applications and RATs as their training set, which makes them difficult to adapt to the real environment. In this article, the authors address the issue of imbalance dataset between normal and RAT samples, and propose a highly efficient method of detecting RATs in real traffic. In the authors method, they generate eight features by combining the size, the inter-arrival and the flag from one packet sequence. Then, they preprocess the imbalance dataset and implement a classifier by XGBoost algorithm. The classifier achieves a false negative rate of less than 0.18%. Moreover, the authors demonstrate that their classifier is capable of detecting unknown RAT.
更多查看译文
关键词
Feature Extraction, Machine Learning, Network Behavior, Remote Access Trojan, Traffic
AI 理解论文
溯源树
样例
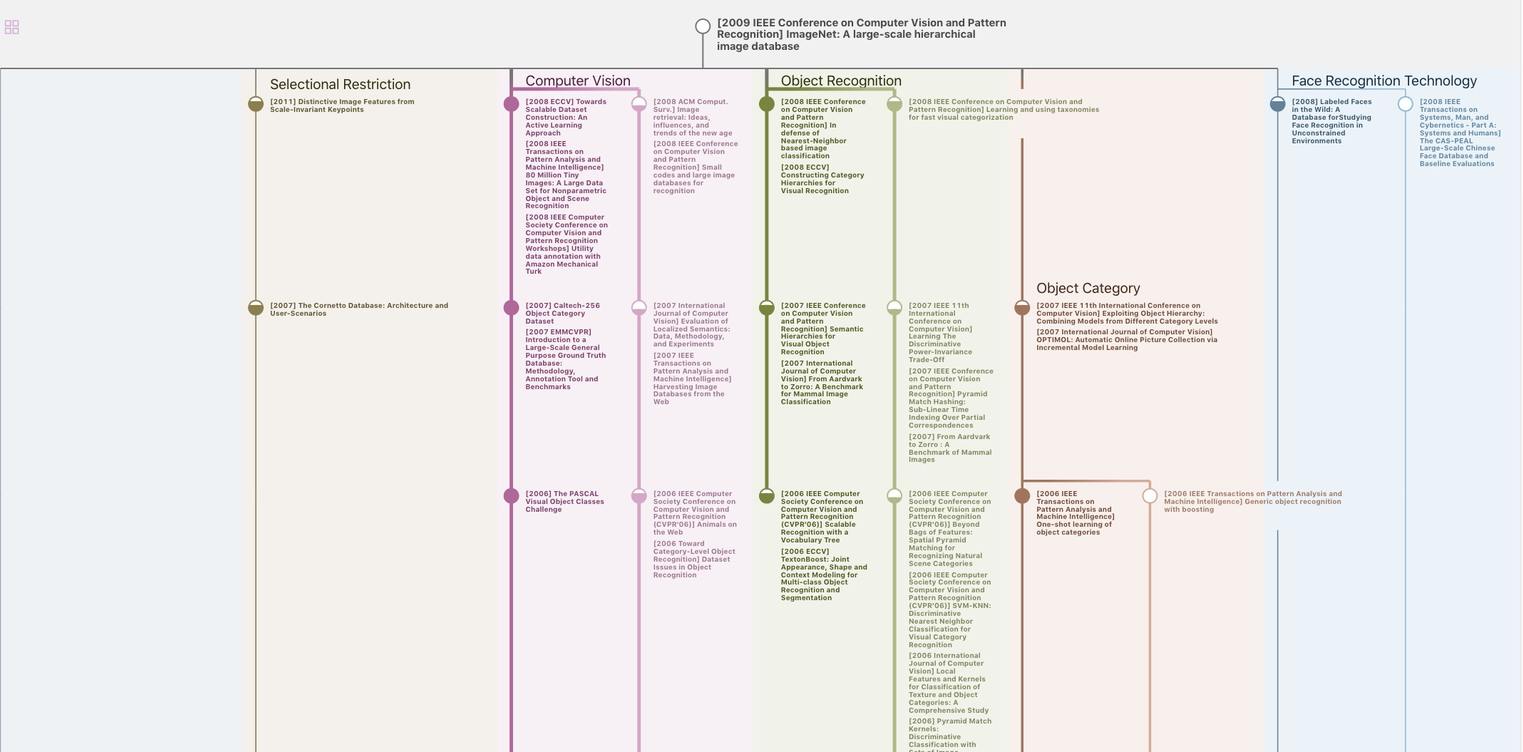
生成溯源树,研究论文发展脉络
Chat Paper
正在生成论文摘要