Adaptive neural dynamic surface control of MIMO uncertain nonlinear systems with time-varying full state constraints and disturbances.
Neurocomputing(2019)
摘要
In order to stabilize multiple-input–multiple-output uncertain strict feedback nonlinear systems with time-varying full-state constraints and external disturbances, an adaptive neural dynamic surface control method is investigated in this paper. A new compensator is proposed, which combines approximation error based adaptive radial basis function neural networks (RBFNNs) and nonlinear disturbance observer (NDOB) to improve the compensate performance of unmodeled dynamics and external disturbances. To prevent that the time-varying full state constraints are violated, the controller is designed via asymmetric time-varying barrier Lyapunov function (ATBLF). Meanwhile, the dynamic surface control is established to avoid the problem of “explosion of complexity” in traditional backstepping design. All closed-loop signals are proved to be semiglobally uniformly ultimate bounded through stability analysis. Finally, the simulation for six degrees of freedom (6-DOF) model unmanned airship are given to illustrate the effectiveness of the proposed control method.
更多查看译文
关键词
Dynamic surface control,Asymmetric time-varying barrier Lyapunov function,Nonlinear disturbance observer (NDOB),Feedback nonlinear systems,RBF neural networks
AI 理解论文
溯源树
样例
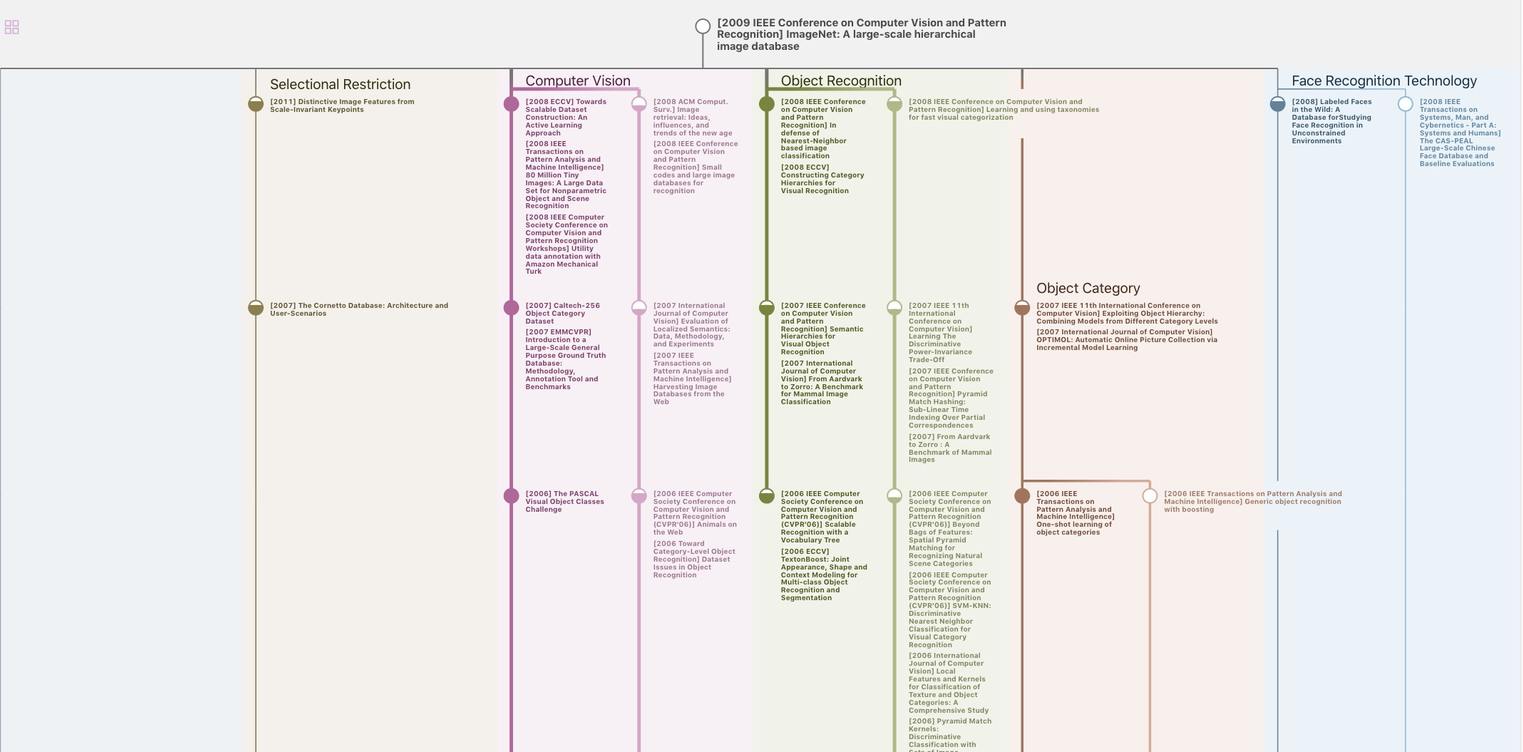
生成溯源树,研究论文发展脉络
Chat Paper
正在生成论文摘要