Deep Sketch-Shape Hashing With Segmented 3D Stochastic Viewing
2019 IEEE/CVF Conference on Computer Vision and Pattern Recognition (CVPR)(2019)
摘要
Sketch-based 3D shape retrieval has been extensively studied in recent works, most of which focus on improving the retrieval accuracy, whilst neglecting the efficiency. In this paper, we propose a novel framework for efficient sketch-based 3D shape retrieval, i.e., Deep Sketch-Shape Hashing (DSSH), which tackles the challenging problem from two perspectives. Firstly, we propose an intuitive 3D shape representation method to deal with unaligned shapes with arbitrary poses. Specifically, the proposed Segmented Stochastic-viewing Shape Network models discriminative 3D representations by a set of 2D images rendered from multiple views, which are stochastically selected from non-overlapping spatial segments of a 3D sphere. Secondly, Batch-Hard Binary Coding (BHBC) is developed to learn semantics-preserving compact binary codes by mining the hardest samples. The overall framework is jointly learned by developing an alternating iteration algorithm. Extensive experimental results on three benchmarks show that DSSH improves both the retrieval efficiency and accuracy remarkably, compared to the state-of-the-art methods.
更多查看译文
关键词
Recognition: Detection,Categorization,Retrieval,3D from Multiview and Sensors,Deep Learning
AI 理解论文
溯源树
样例
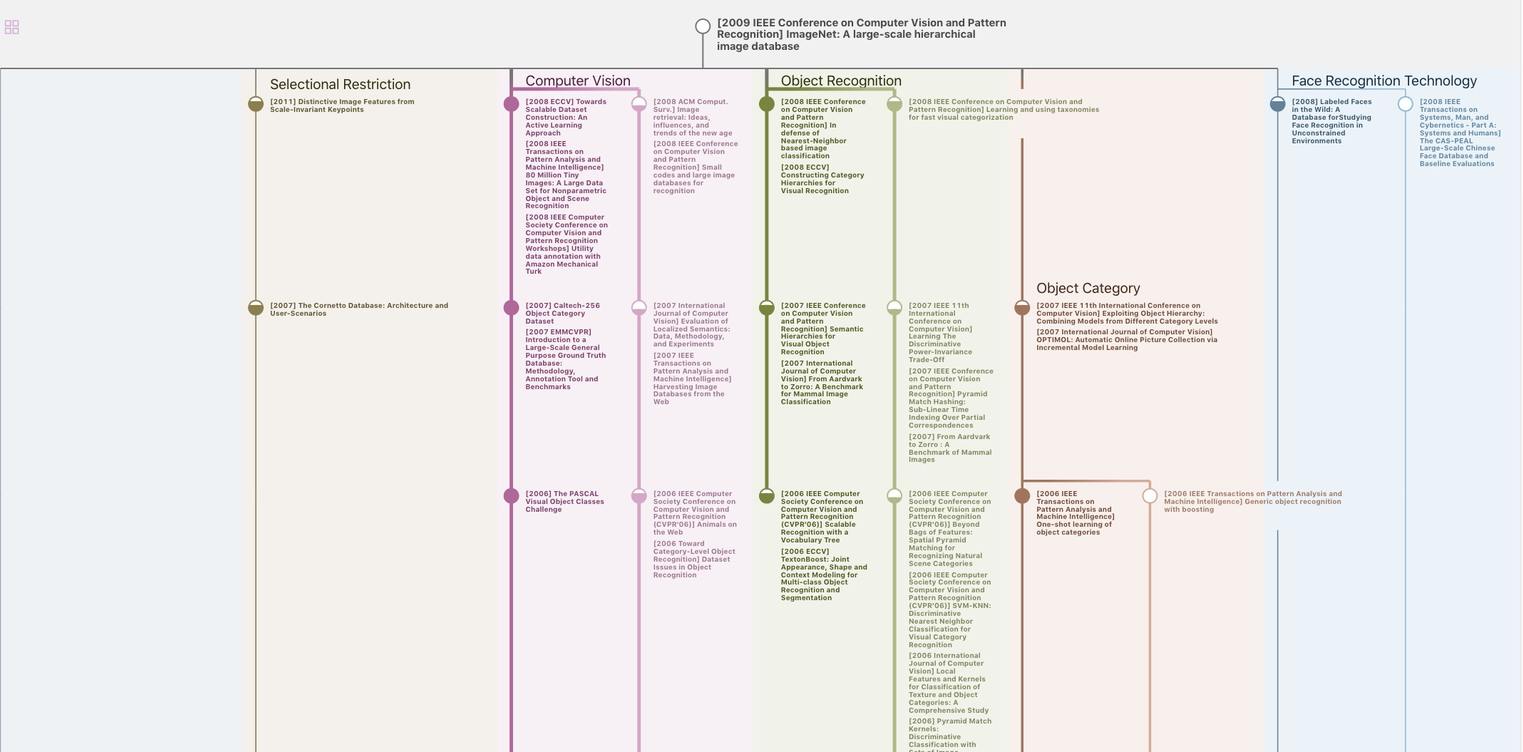
生成溯源树,研究论文发展脉络
Chat Paper
正在生成论文摘要