Barrage Of Random Transforms For Adversarially Robust Defense
2019 IEEE/CVF CONFERENCE ON COMPUTER VISION AND PATTERN RECOGNITION (CVPR 2019)(2019)
摘要
Defenses against adversarial examples, when using the ImageNet dataset, are historically easy to defeat. The common understanding is that a combination of simple image transformations and other various defenses are insufficient to provide the necessary protection when the obfuscated gradient is taken into account. In this paper, we explore the idea of stochastically combining a large number of individually weak defenses into a single barrage of randomized transformations to build a strong defense against adversarial attacks. We show that, even after accounting for obfuscated gradients, the Barrage of Random Transforms (BaRT) is a resilient defense against even the most difficult attacks, such as PGD. BaRT achieves up to a 24x improvement in accuracy compared to previous work, and has even extended effectiveness out to a previously untested maximum adversarial perturbation of epsilon = 32.
更多查看译文
关键词
Recognition: Detection,Categorization,Retrieval,Big Data,Large Scale Methods,Deep Learning
AI 理解论文
溯源树
样例
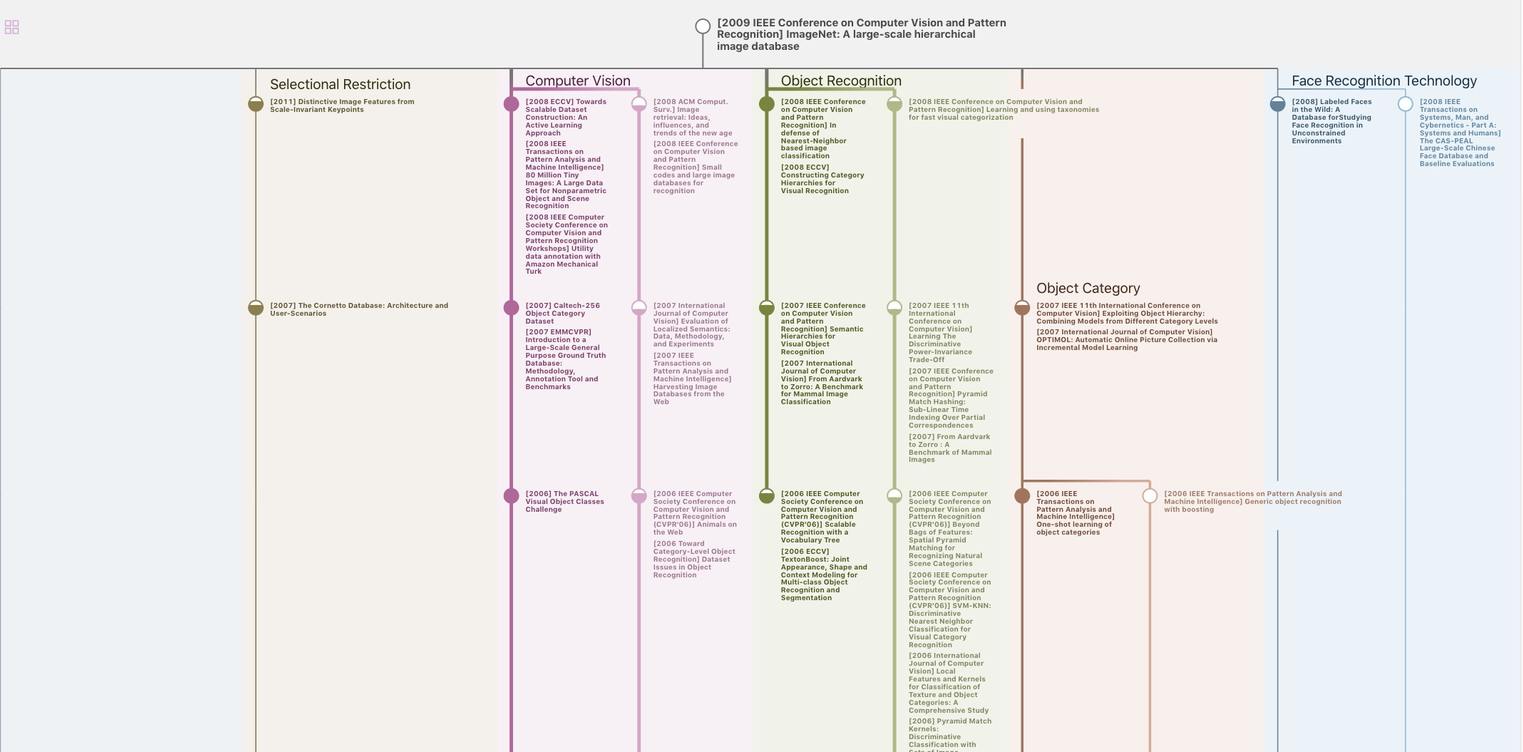
生成溯源树,研究论文发展脉络
Chat Paper
正在生成论文摘要