Retrieval-Augmented Convolutional Neural Networks Against Adversarial Examples
2019 IEEE/CVF CONFERENCE ON COMPUTER VISION AND PATTERN RECOGNITION (CVPR 2019)(2019)
摘要
We propose a retrieval-augmented convolutional network (RaCNN) and propose to train it with local mixup, a novel variant of the recently proposed mixup algorithm. The proposed hybrid architecture combining a convolutional network and an off-the-shelf retrieval engine was designed to mitigate the adverse effect of off-manifold adversarial examples, while the proposed local mixup addresses on-manifold ones by explicitly encouraging the classifier to locally behave linearly on the data manifold. Our evaluation of the proposed approach against seven readily available adversarial attacks on three datasets CIFAR-10, SVHN and ImageNet demonstrate the improved robustness compared to a vanilla convolutional network, and comparable performance with the state-of-the-art reactive defense approaches.
更多查看译文
关键词
Recognition: Detection,Categorization,Retrieval,Deep Learning
AI 理解论文
溯源树
样例
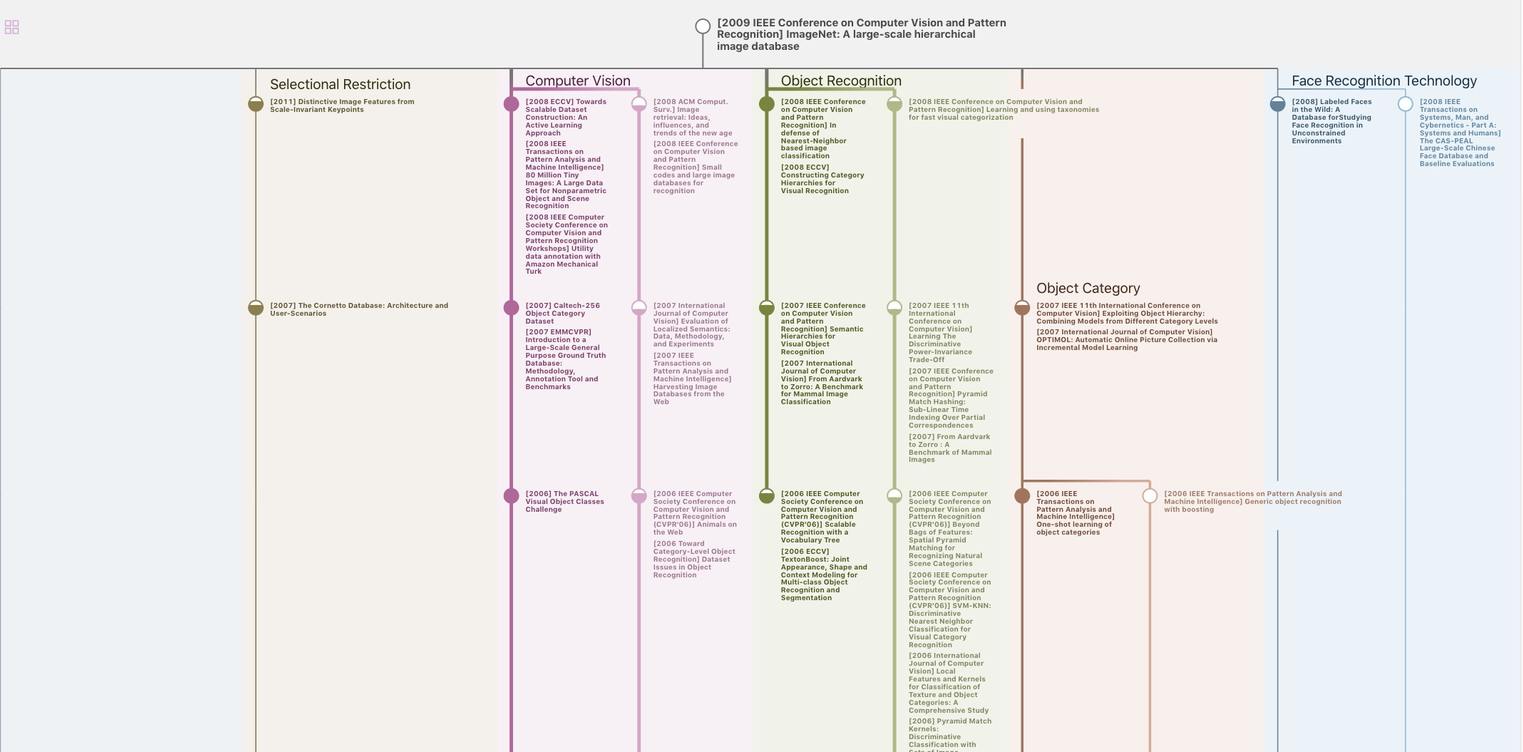
生成溯源树,研究论文发展脉络
Chat Paper
正在生成论文摘要