Preoperative Planning For Guidewires Employing Shape-Regularized Segmentation And Optimized Trajectories
OR 2.0 CONTEXT-AWARE OPERATING THEATERS AND MACHINE LEARNING IN CLINICAL NEUROIMAGING(2019)
摘要
Upcoming robotic interventions for endovascular procedures can significantly reduce the high radiation exposure currently endured by surgeons. Robotically driven guidewires replace manual insertion and leave the surgeon the task of planning optimal trajectories based on segmentation of associated risk structures. However, such a pipeline brings new challenges. While Deep learning based segmentation such as U-Net can achieve outstanding Dice scores, it fails to provide suitable results for trajectory planning in annotation scarce environments. We propose a preoperative pipeline featuring a shape regularized U-Net that extracts coherent anatomies from pixelwise predictions. It uses Rapidly-exploring Random Trees together with convex optimization for locally optimal planning. Our experiments on two publicly available data sets evaluate the complete pipeline. We show the benefits of our approach in a functional evaluation including both segmentation and planning metrics: While we achieve comparable Dice, Hausdorff distances and planning metrics such as success rate of motion planning algorithms are significantly better than U-Net.
更多查看译文
关键词
Preoperative planning, Shape regularization, Functional evaluation, Endovascular procedures
AI 理解论文
溯源树
样例
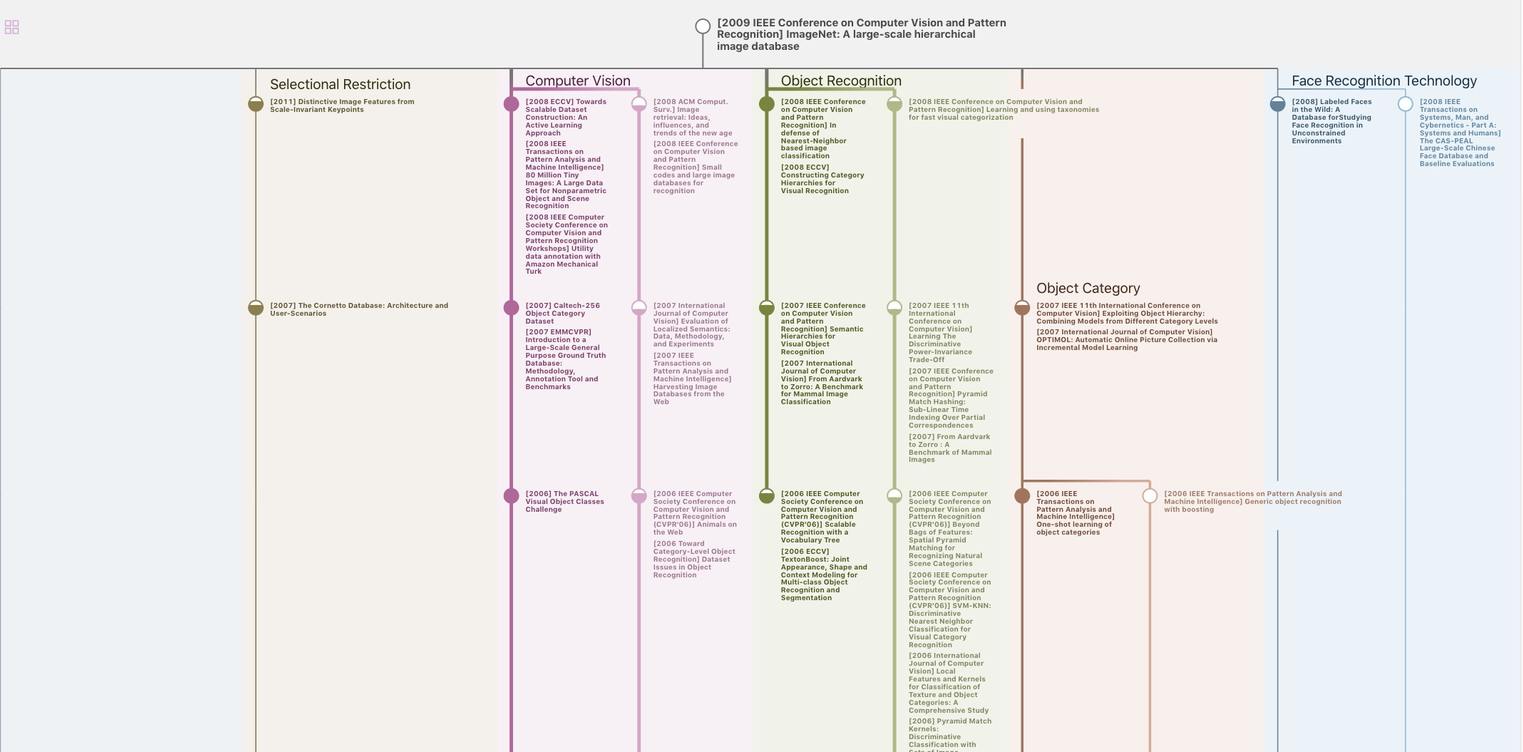
生成溯源树,研究论文发展脉络
Chat Paper
正在生成论文摘要