Infinite-horizon Off-Policy Policy Evaluation with Multiple Behavior Policies
arXiv (Cornell University)(2019)
摘要
We consider off-policy policy evaluation when the trajectory data are generated by multiple behavior policies. Recent work has shown the key role played by the state or state-action stationary distribution corrections in the infinite horizon context for off-policy policy evaluation. We propose estimated mixture policy (EMP), a novel class of partially policy-agnostic methods to accurately estimate those quantities. With careful analysis, we show that EMP gives rise to estimates with reduced variance for estimating the state stationary distribution correction while it also offers a useful induction bias for estimating the state-action stationary distribution correction. In extensive experiments with both continuous and discrete environments, we demonstrate that our algorithm offers significantly improved accuracy compared to the state-of-the-art methods.
更多查看译文
关键词
Mixed-Effects Models,Observational Studies
AI 理解论文
溯源树
样例
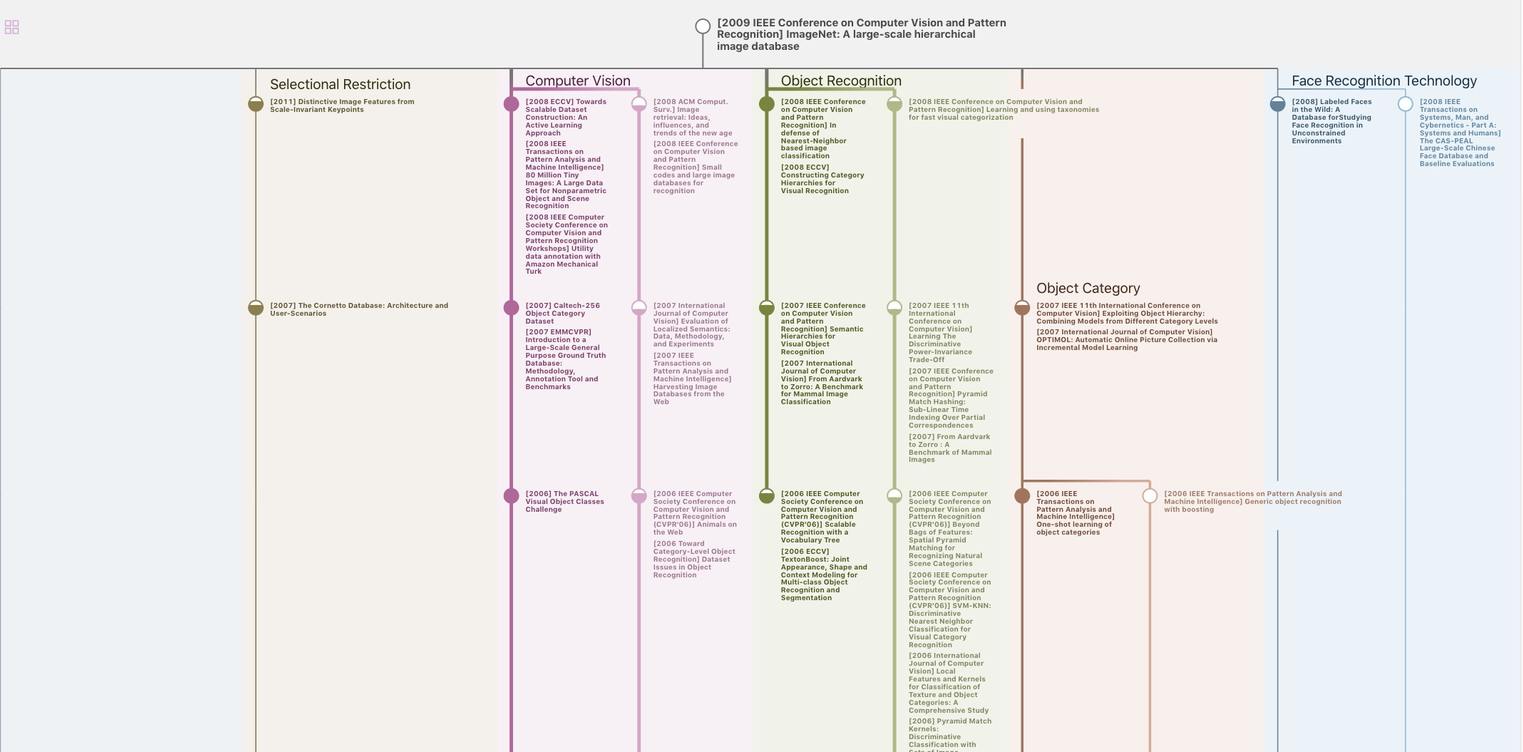
生成溯源树,研究论文发展脉络
Chat Paper
正在生成论文摘要