Old Dog Learns New Tricks: Randomized Ucb For Bandit Problems
INTERNATIONAL CONFERENCE ON ARTIFICIAL INTELLIGENCE AND STATISTICS, VOL 108(2020)
摘要
We propose RandUCB, a bandit strategy that builds on theoretically derived confidence intervals similar to upper confidence bound (UCB) algorithms, but akin to Thompson sampling (TS), it uses randomization to trade off exploration and exploitation. In the Karmed bandit setting, we show that there are infinitely many variants of RandUCB, all of which achieve the minimax-optimal 0( \/KT) regret after T rounds. Moreover, for a specific multi-armed bandit setting, we show that both UCB and TS can be recovered as special cases of RandUCB. For structured bandits, where each arm is associated with a d-dimensional feature vector and rewards are distributed according to a linear or generalized linear model, we prove that RandUCB achieves the minimax-optimal O(dVT) regret even in the case of infinitely many arms. Through experiments in both the multi-armed and structured bandit settings, we demonstrate that RandUCB matches or outperforms TS and other randomized exploration strategies. Our theoretical and empirical results together imply that RandUCB achieves the best of both worlds.
更多查看译文
AI 理解论文
溯源树
样例
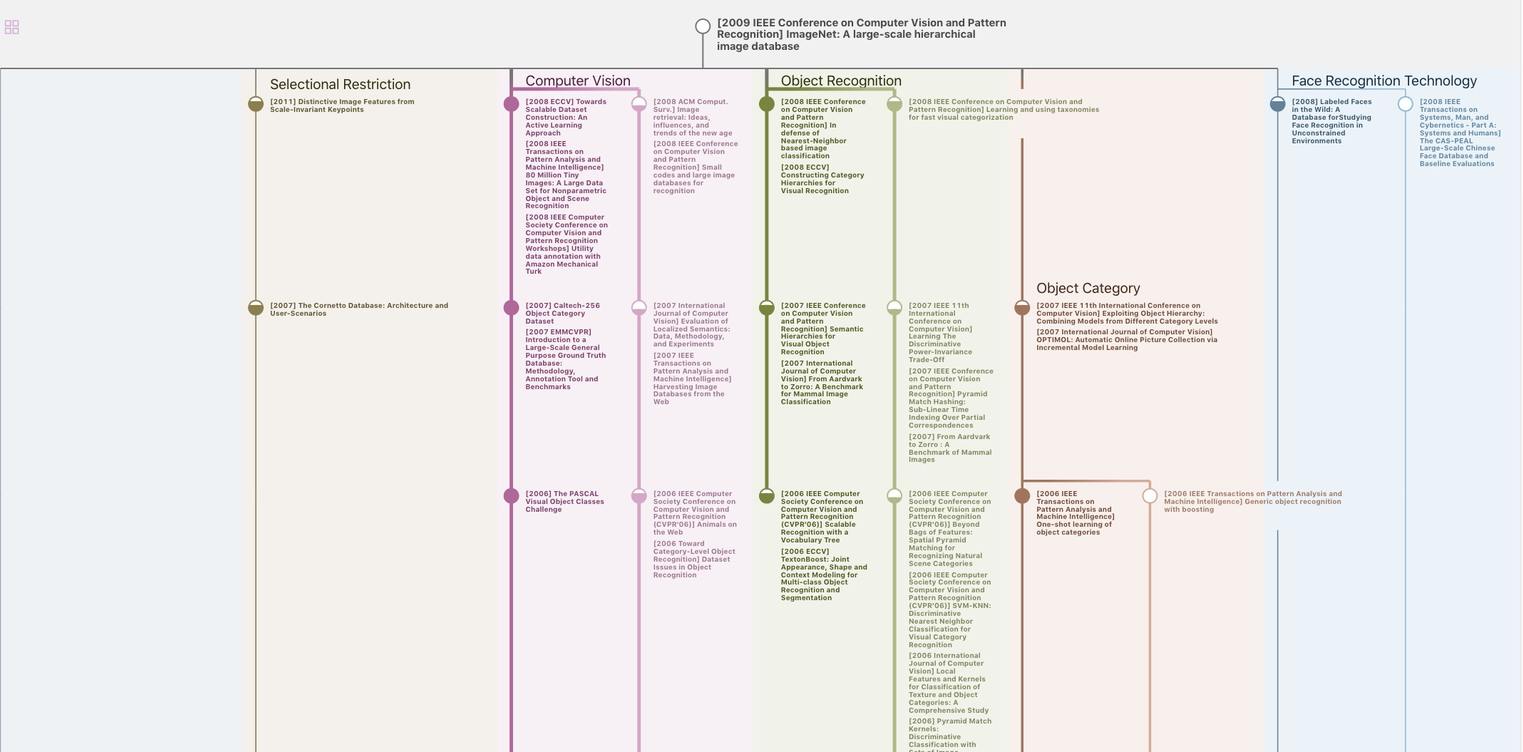
生成溯源树,研究论文发展脉络
Chat Paper
正在生成论文摘要