Deep Kernel Transfer in Gaussian Processes for Few-shot Learning
arxiv(2019)
摘要
Humans tackle new problems by making inferences that go far beyond the information available, reusing what they have previously learned, and weighing different alternatives in the face of uncertainty. Incorporating these abilities in an artificial system is a major objective in machine learning. Towards this goal, we introduce a Bayesian method based on Gaussian Processes (GPs) that can learn efficiently from a limited amount of data and generalize across new tasks and domains. We frame few-shot learning as a model selection problem by learning a deep kernel across tasks, and then using this kernel as a covariance function in a GP prior for Bayesian inference. This probabilistic treatment allows for cross-domain flexibility, and uncertainty quantification. We provide substantial experimental evidence, showing that the proposed method is better than several state-of-the-art algorithms in few-shot regression and cross-domain classification.
更多查看译文
AI 理解论文
溯源树
样例
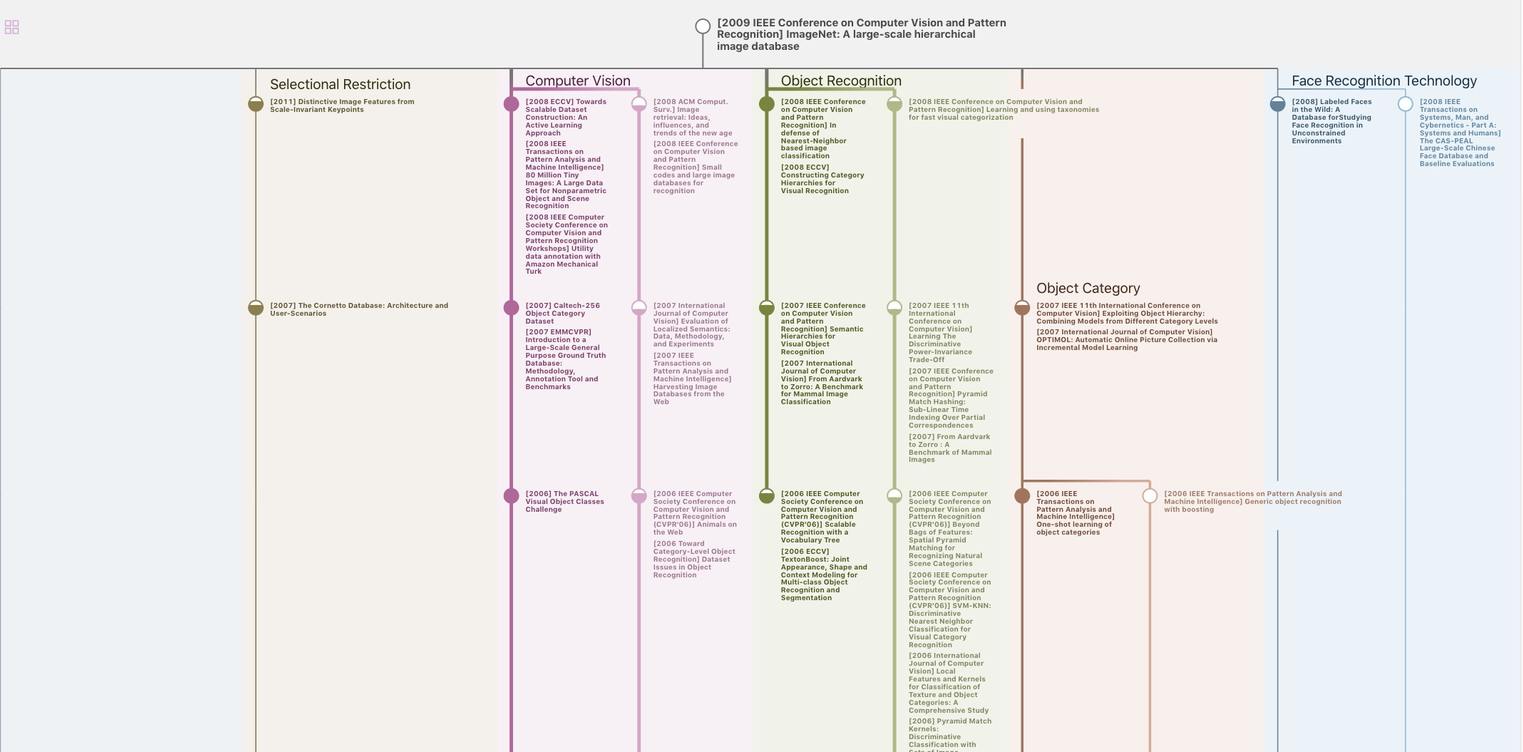
生成溯源树,研究论文发展脉络
Chat Paper
正在生成论文摘要