Shape Constrained Network for Eye Segmentation in the Wild
WACV(2020)
摘要
Semantic segmentation of eyes has long been a vital pre-processing step in many biometric applications. Majority of the works focus only on high resolution eye images, while little has been done to segment the eyes from low quality images in the wild. However, this is a particularly interesting and meaningful topic, as eyes play a crucial role in conveying the emotional state and mental well-being of a person. In this work, we take two steps toward solving this problem: (1) We collect and annotate a challenging eye segmentation dataset containing 8882 eye patches from 4461 facial images of different resolutions, illumination conditions and head poses; (2) We develop a novel eye segmentation method, Shape Constrained Network (SCN), that incorporates shape prior into the segmentation network training procedure. Specifically, we learn the shape prior from our dataset using VAE-GAN, and leverage the pre-trained encoder and discriminator to regularise the training of SegNet. To improve the accuracy and quality of predicted masks, we replace the loss of SegNet with three new losses: Intersection-over-Union (IoU) loss, shape discriminator loss and shape embedding loss. Extensive experiments shows that our method outperforms state-of-the-art segmentation and landmark detection methods in terms of mean IoU (mIoU) accuracy and the quality of segmentation masks. The eye segmentation database is available at https://www.dropbox.com/s/yvveouvxsvti08x/Eye_Segmentation_Database.zip?dl=0.
更多查看译文
关键词
eye segmentation method,Shape Constrained Network,segmentation network training procedure,Intersection-over-Union loss,landmark detection methods,semantic segmentation,vital pre-processing step,high resolution eye images,low quality images,VAE-GAN
AI 理解论文
溯源树
样例
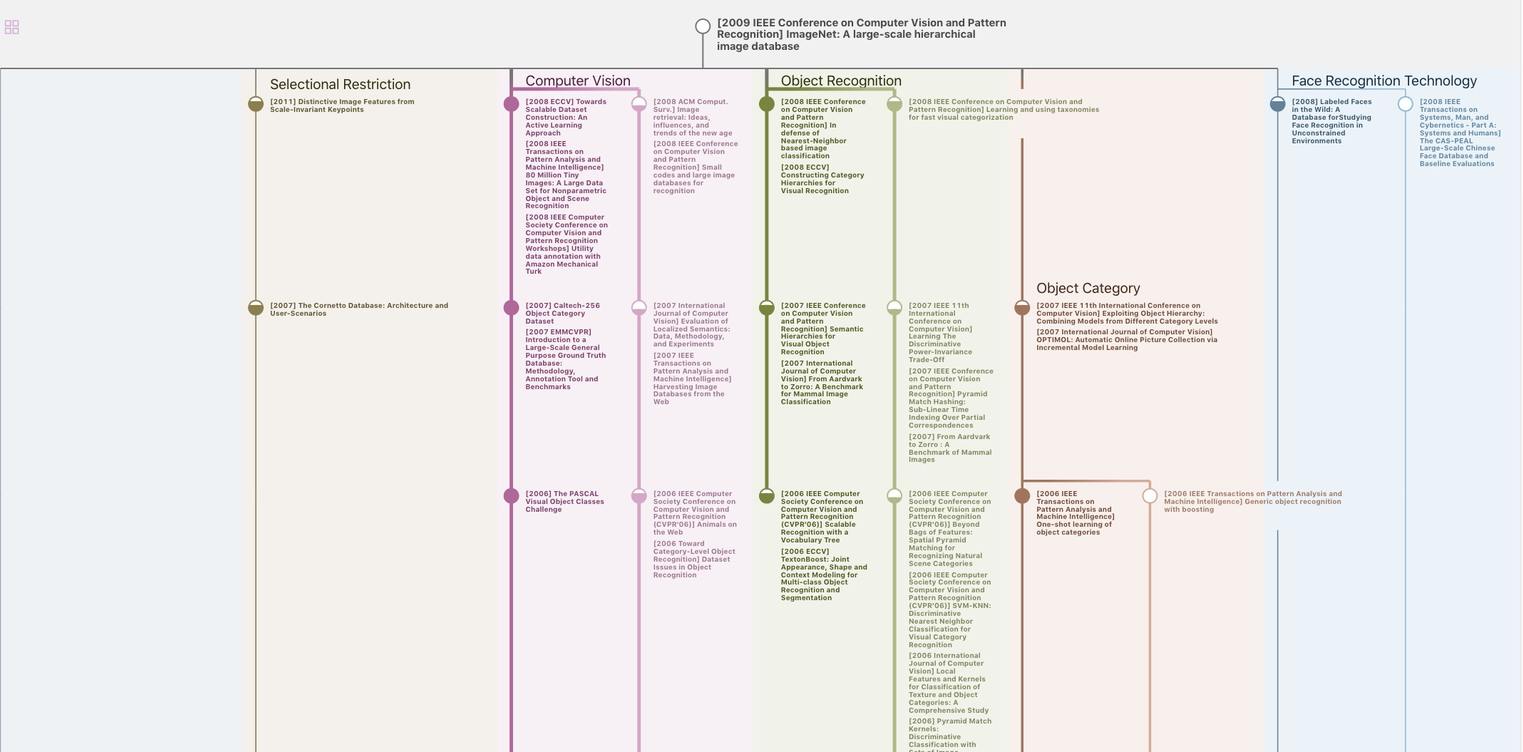
生成溯源树,研究论文发展脉络
Chat Paper
正在生成论文摘要