Spectral embedding of weighted graphs
arxiv(2023)
摘要
When analyzing weighted networks using spectral embedding, a judicious transformation of the edge weights may produce better results. To formalize this idea, we consider the asymptotic behavior of spectral embedding for different edge-weight representations, under a generic low rank model. We measure the quality of different embeddings -- which can be on entirely different scales -- by how easy it is to distinguish communities, in an information-theoretic sense. For common types of weighted graphs, such as count networks or p-value networks, we find that transformations such as tempering or thresholding can be highly beneficial, both in theory and in practice.
更多查看译文
关键词
Chernoff information, Gaussian mixture model, Matrix factorization, Network, Stochastic block model
AI 理解论文
溯源树
样例
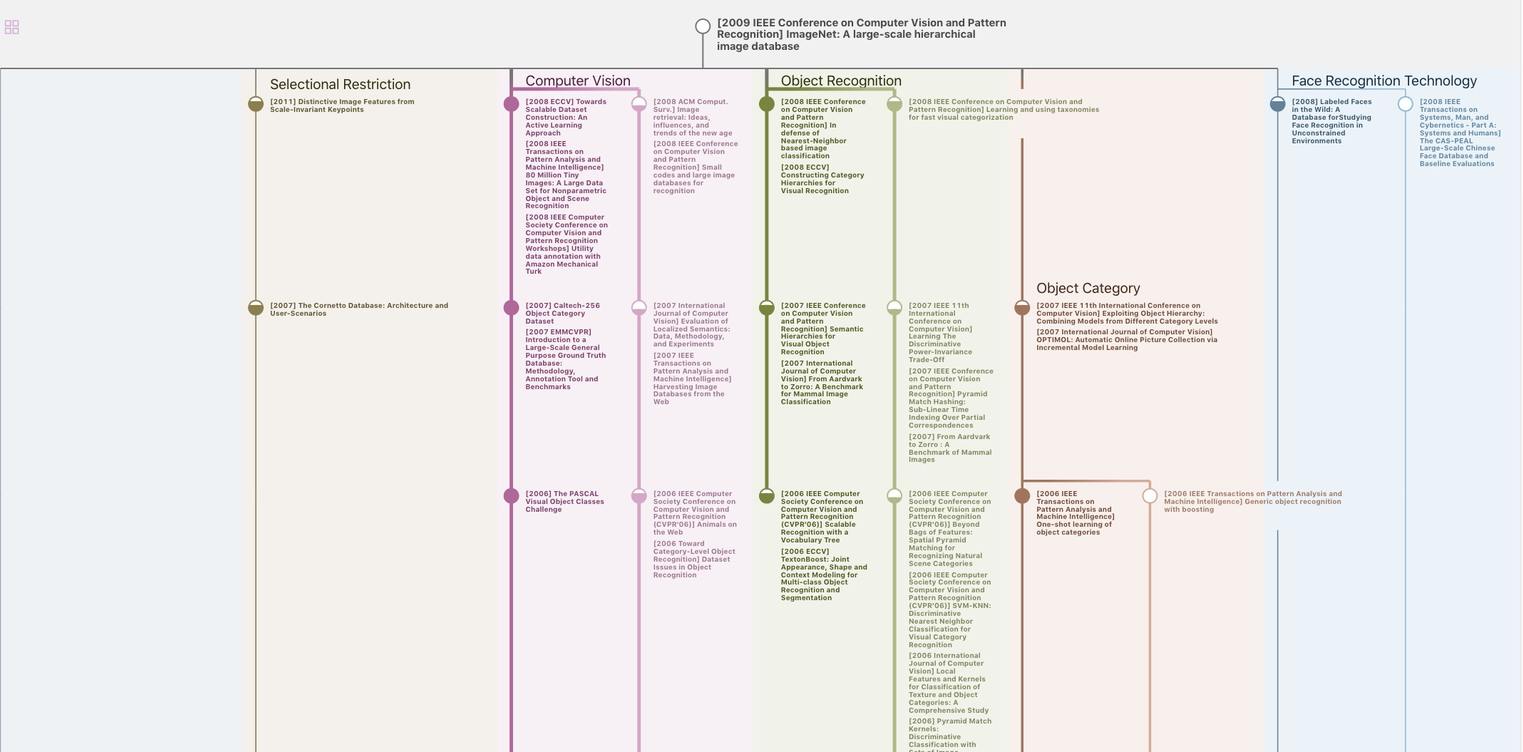
生成溯源树,研究论文发展脉络
Chat Paper
正在生成论文摘要