More Powerful Selective Kernel Tests For Feature Selection
INTERNATIONAL CONFERENCE ON ARTIFICIAL INTELLIGENCE AND STATISTICS, VOL 108(2020)
摘要
Refining one's hypotheses in the light of data is a common scientific practice; however, the dependency on the data introduces selection bias and can lead to specious statistical analysis. An approach for addressing this is via conditioning on the selection procedure to account for how we have used the data to generate our hypotheses, and prevent information to be used again after selection. Many selective inference (a.k.a. post-selection inference) algorithms typically take this approach but will "over-condition" for sake of tractability. While this practice yields well calibrated statistic tests with controlled false positive rates (FPR), it can incur a major loss in power. In our work, we extend two recent proposals for selecting features using the Maximum Mean Discrepancy and Hilbert Schmidt Independence Criterion to condition on the minimal conditioning event. We show how recent advances in multiscale bootstrap makes conditioning on the minimal selection event possible and demonstrate our proposal over a range of synthetic and real world experiments. Our results show that our proposed test is indeed more powerful in most scenarios.
更多查看译文
AI 理解论文
溯源树
样例
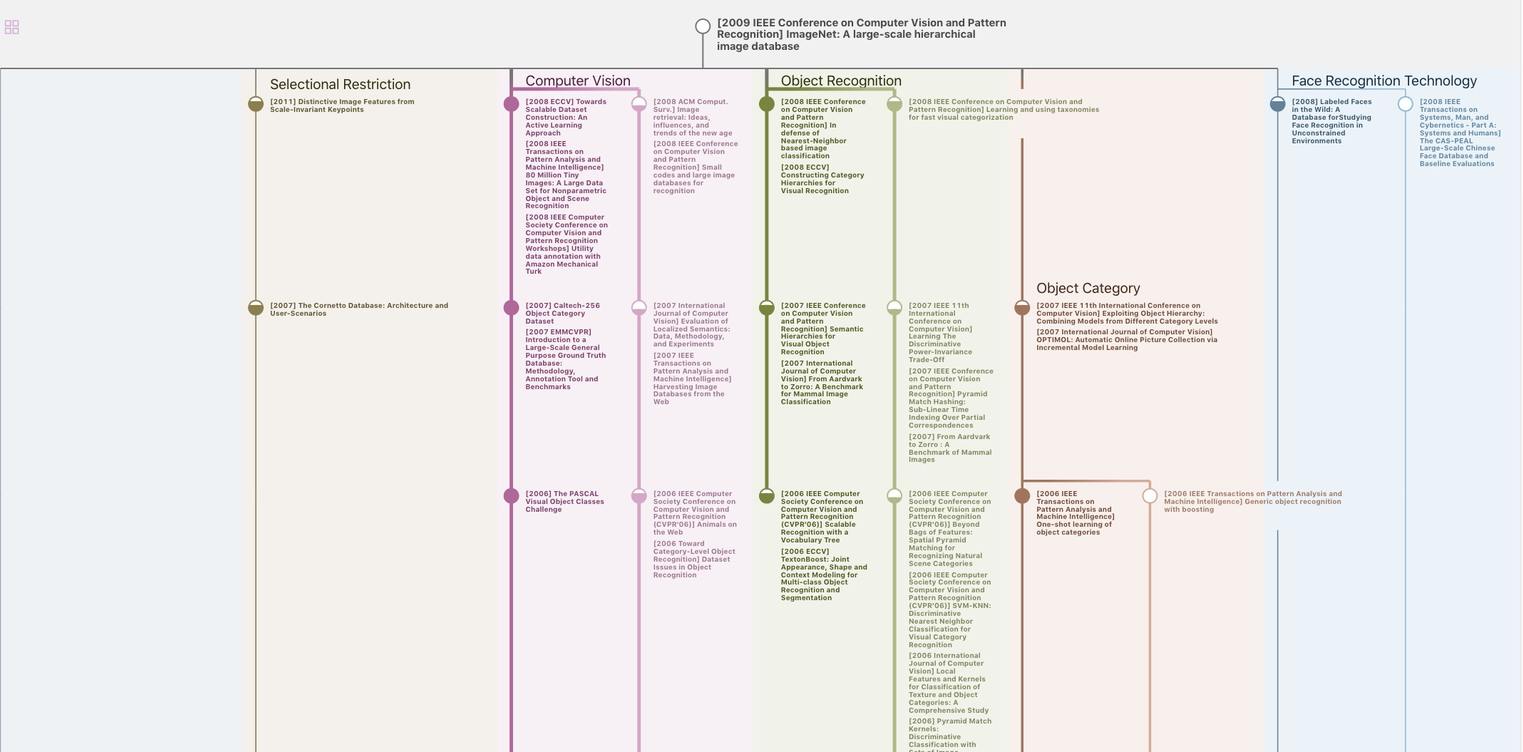
生成溯源树,研究论文发展脉络
Chat Paper
正在生成论文摘要