Gaussian Processes with Errors in Variables: Theory and Computation
JOURNAL OF MACHINE LEARNING RESEARCH(2023)
摘要
Covariate measurement error in nonparametric regression is a common problem in nutritional epidemiology and geostatistics, and other fields. Over the last two decades, this problem has received substantial attention in the frequentist literature. Bayesian approaches for handling measurement error have only been explored recently and are surprisingly successful, although there still is a lack of a proper theoretical justification regarding the asymptotic performance of the estimators. By specifying a Gaussian process prior on the regression function and a Dirichlet process Gaussian mixture prior on the unknown distribution of the unobserved covariates, we show that the posterior distribution of the regression function and the unknown covariate density attain optimal rates of contraction adaptively over a range of Ho center dot lder classes, up to logarithmic terms. We also develop a novel surrogate prior for approximating the Gaussian process prior that leads to efficient computation and preserves the covariance structure, thereby facilitating easy prior elicitation. We demon strate the empirical performance of our approach and compare it with competitors in a wide range of simulation experiments and a real data example.
更多查看译文
关键词
Approximated Gaussian processes,measurement error model,nonparametric Bayes,smoothing and nonparametric regression,supersmooth errors
AI 理解论文
溯源树
样例
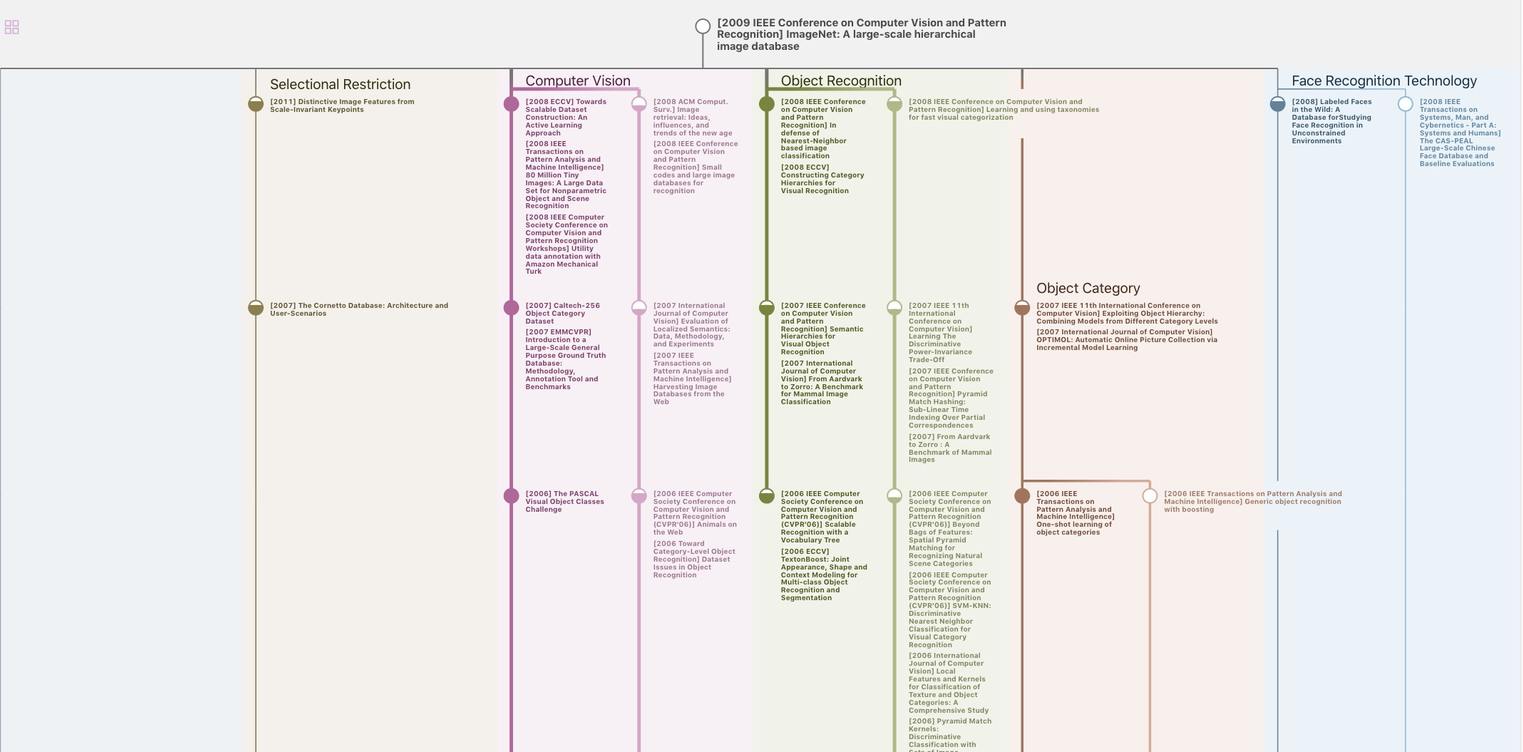
生成溯源树,研究论文发展脉络
Chat Paper
正在生成论文摘要