Transformer with Gaussian weighted self-attention for speech enhancement
arxiv(2019)
摘要
The Transformer architecture recently replaced recurrent neural networks such as LSTM or GRU on many natural language processing (NLP) tasks by presenting new state of the art performance. Self-attention is a core building block for Transformer, which not only enables parallelization of sequence computation but also provides the constant path length between symbols that is essential to learn long-range dependencies. However, Transformer did not perform well for speech enhancement due to the lack of consideration for speech and noise physical characteristics. In this paper, we propose Gaussian weighted self-attention that attenuates attention weights according to the distance between target and context symbols. The experimental results showed that the proposed attention scheme significantly improved over the original Transformer as well as recurrent networks.
更多查看译文
AI 理解论文
溯源树
样例
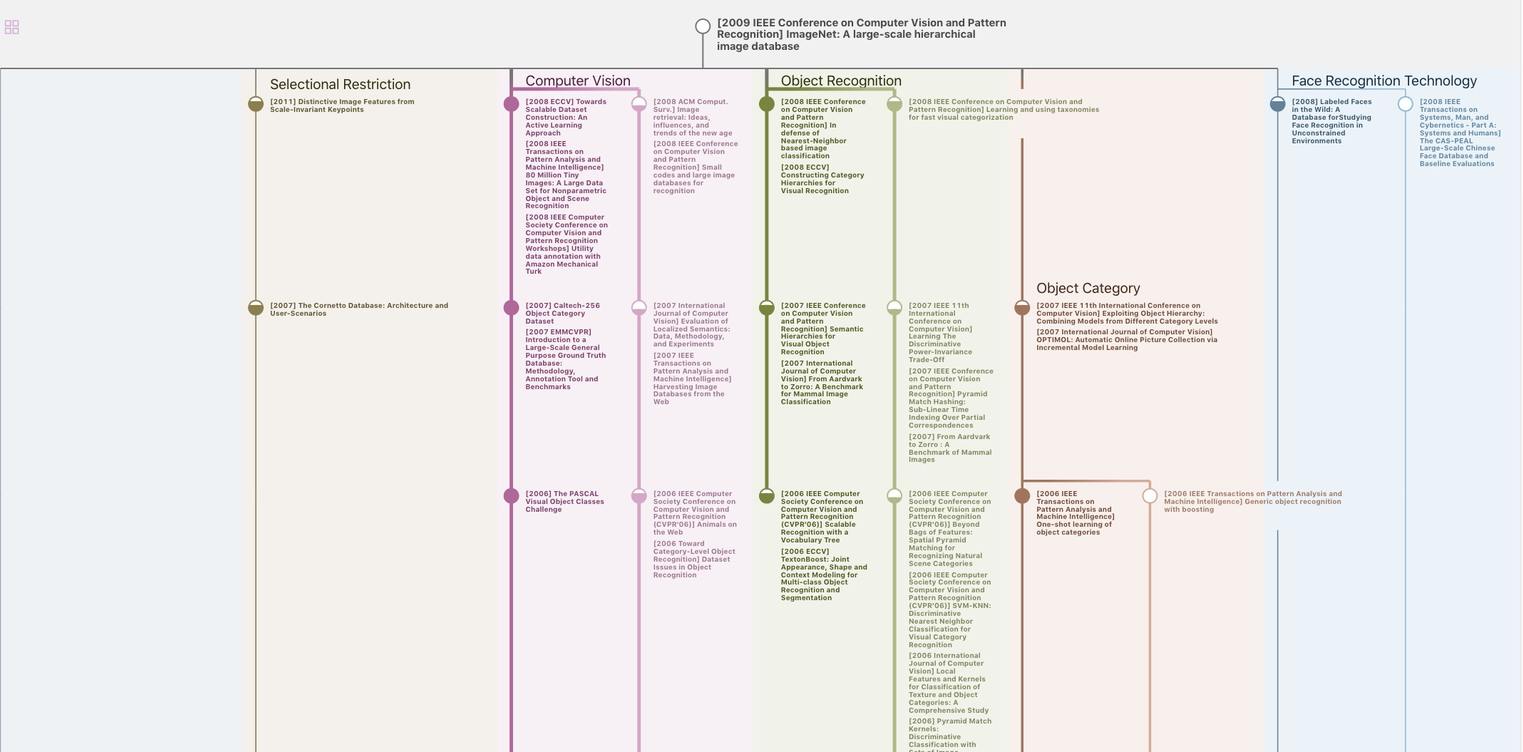
生成溯源树,研究论文发展脉络
Chat Paper
正在生成论文摘要